Gridsemble: Selective Ensembling for False Discovery Rates
arxiv(2024)
摘要
In this paper, we introduce Gridsemble, a data-driven selective ensembling algorithm for estimating local false discovery rates (fdr) in large-scale multiple hypothesis testing. Existing methods for estimating fdr often yield different conclusions, yet the unobservable nature of fdr values prevents the use of traditional model selection. There is limited guidance on choosing a method for a given dataset, making this an arbitrary decision in practice. Gridsemble circumvents this challenge by ensembling a subset of methods with weights based on their estimated performances, which are computed on synthetic datasets generated to mimic the observed data while including ground truth. We demonstrate through simulation studies and an experimental application that this method outperforms three popular R software packages with their default parameter values$\unicode{x2014}$common choices given the current landscape. While our applications are in the context of high throughput transcriptomics, we emphasize that Gridsemble is applicable to any use of large-scale multiple hypothesis testing, an approach that is utilized in many fields. We believe that Gridsemble will be a useful tool for computing reliable estimates of fdr and for improving replicability in the presence of multiple hypotheses by eliminating the need for an arbitrary choice of method. Gridsemble is implemented in an open-source R software package available on GitHub at jennalandy/gridsemblefdr.
更多查看译文
AI 理解论文
溯源树
样例
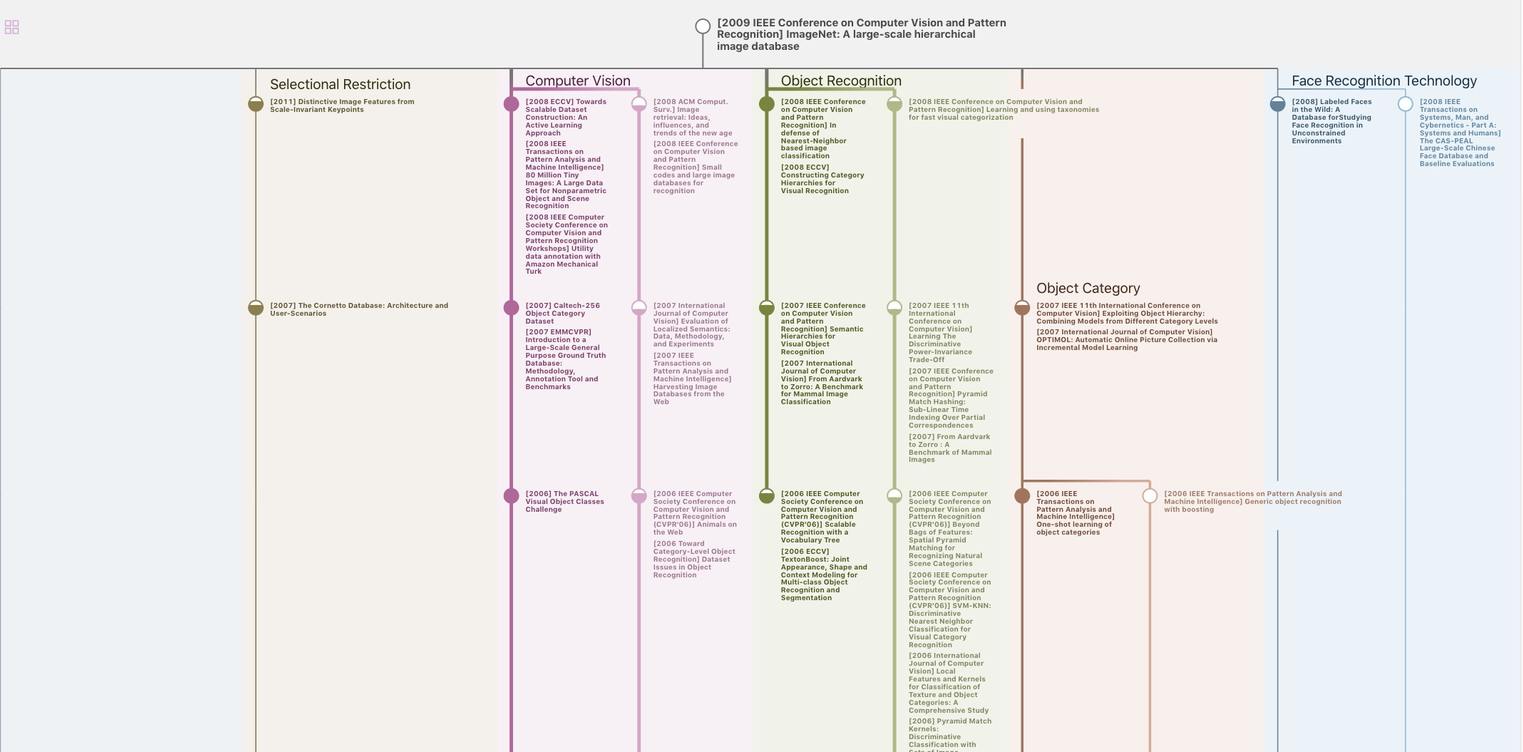
生成溯源树,研究论文发展脉络
Chat Paper
正在生成论文摘要