Few-Shot Object Counting With Dynamic Similarity-Aware in Latent Space.
IEEE Transactions on Geoscience and Remote Sensing(2024)
摘要
Few-shot object counting (FSOC) estimates object quantities in query images using a few of support information. Unlike traditional counting methods, FSOC prioritizes more discriminative and generalized similarity measures between query and support data. This facilitates counting objects from new categories without extensive dataset creation or costly retraining. However, existing approaches often rely on fixed similarity rules, leading to spatial information loss. Limited training data can yield sparse similarity feature distribution, hampering the model’s learning and its ability to handle objects with large intraclass differences. In this study, we introduce a novel FSOC network named DSALVANet that comprises the dynamic similarity-aware module (DSAM) and the latent variable augmentation module (LVAM). DSAM establishes adaptive metric rules for support features to find similar regions in the metric space for accurate object counting. LVAM utilizes prior similarity knowledge from DSAM to model the latent distribution of the density map, improving the decoder’s robustness by sampling diverse latent variables during training. Extensive experiments on the FSOC benchmark and remote-sensing datasets demonstrate our method’s effectiveness and state-of-the-art performance. The code and model are available at DSALVANet.
更多查看译文
关键词
Few-shot object counting (FSOC),latent variable augmentation,metric learning
AI 理解论文
溯源树
样例
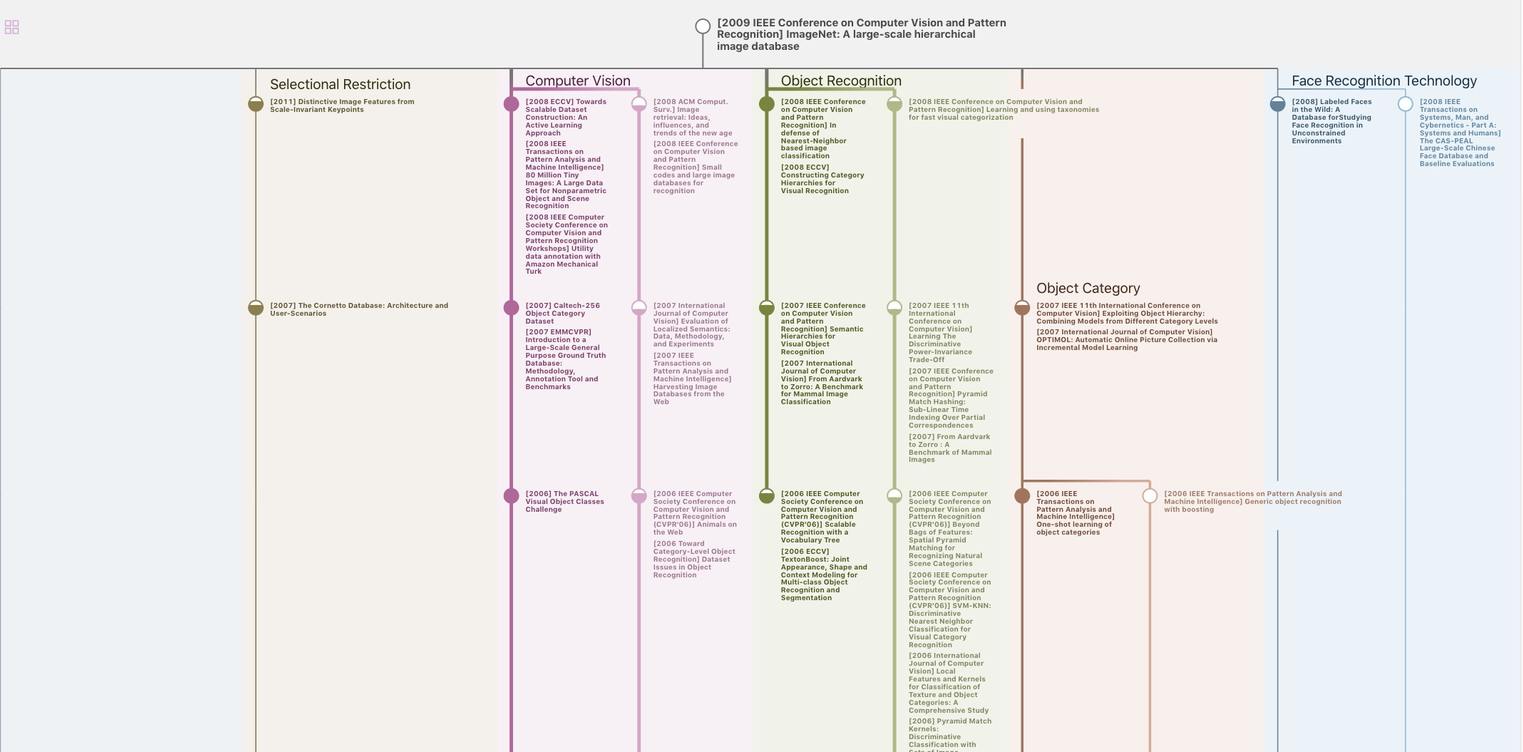
生成溯源树,研究论文发展脉络
Chat Paper
正在生成论文摘要