pFedLN: Personalized Federated Learning Framework With Layer-Wised and Neighbor-Based Aggregation for QoS Prediction
IEEE ACCESS(2024)
摘要
In the era of a more advanced and intelligent Internet, the highly sophisticated service-oriented internet provides users with a diverse array of similar services. Accurate Quality of Service (QoS) prediction plays a pivotal role in helping users choose the optimal service from a multitude of available options. Traditional federated learning models offer a secure method for multiple clients to collaborate on QoS predictions. However, these models still employ a uniform approach that overlooks the unique requirements of individual clients. In order to meet the different needs of a wide range of customers for models, we propose an innovative personalized federated learning framework with layer-wised and neighbor-based aggregation for QoS prediction (pFedLN). In the proposed framework, we consider the privacy and functional disparities among layers in neural network models and employ diverse aggregation strategies for layers serving different functions. In addition, the similarity between neighbors will be taken into account during the aggregation process. This results in the creation of personalized models for each client that better align with their specific requirements. Sufficient experiments are conducted on a real-world dataset and the results indicate that our approach have a clear advantage in improving the effectiveness of personalization compared to existing approaches.
更多查看译文
关键词
Internet of service,personalized federated learning,QoS prediction
AI 理解论文
溯源树
样例
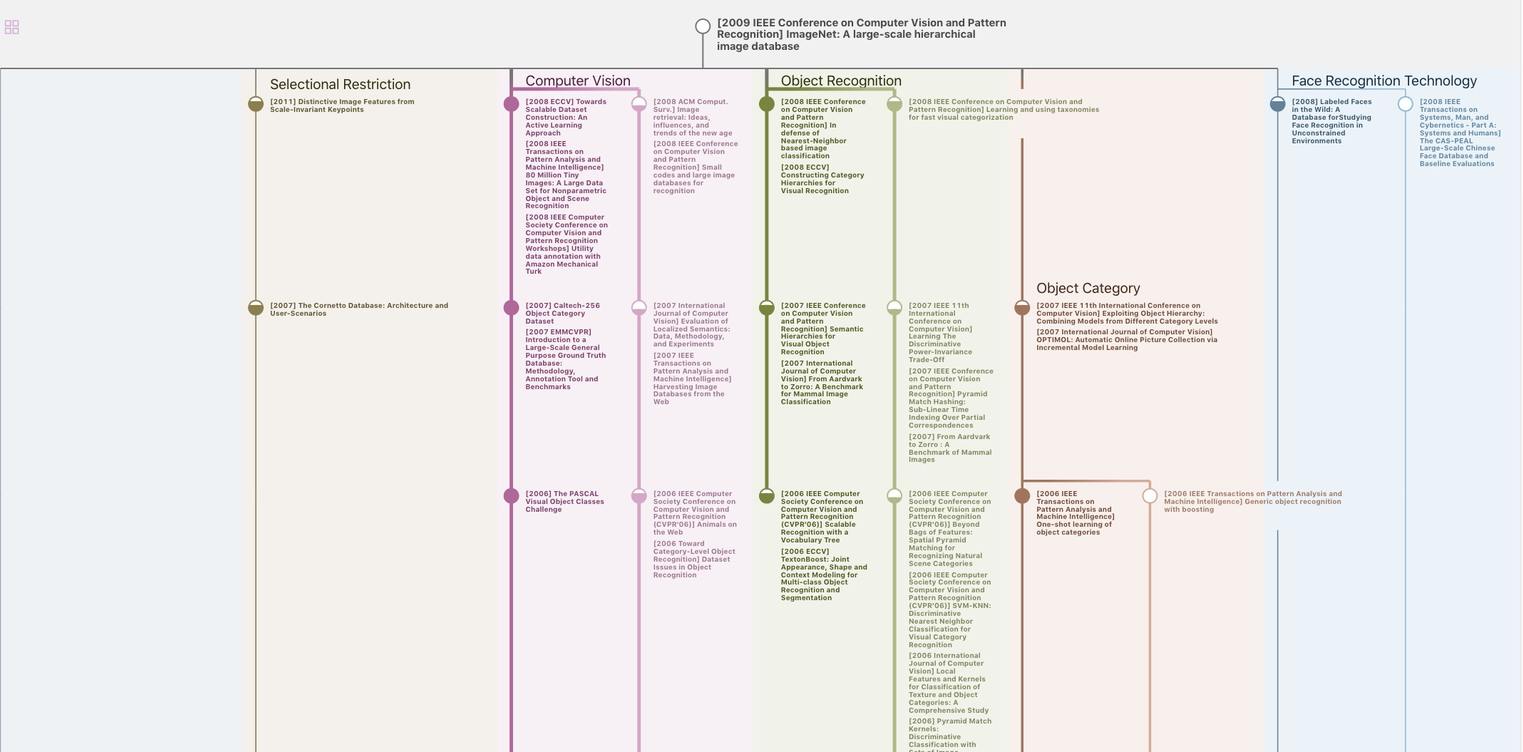
生成溯源树,研究论文发展脉络
Chat Paper
正在生成论文摘要