Less Could Be Better: Parameter-efficient Fine-tuning Advances Medical Vision Foundation Models
CoRR(2024)
摘要
Parameter-efficient fine-tuning (PEFT) that was initially developed for
exploiting pre-trained large language models has recently emerged as an
effective approach to perform transfer learning on computer vision tasks.
However, the effectiveness of PEFT on medical vision foundation models is still
unclear and remains to be explored. As a proof of concept, we conducted a
detailed empirical study on applying PEFT to chest radiography foundation
models. Specifically, we delved into LoRA, a representative PEFT method, and
compared it against full-parameter fine-tuning (FFT) on two self-supervised
radiography foundation models across three well-established chest radiograph
datasets. Our results showed that LoRA outperformed FFT in 13 out of 18
transfer learning tasks by at most 2.9
Combining LoRA with foundation models, we set up new state-of-the-art on a
range of data-efficient learning tasks, such as an AUROC score of 80.6
1
attention from the community in the use of PEFT for transfer learning on
medical imaging tasks. Code and models are available at
https://github.com/RL4M/MED-PEFT.
更多查看译文
AI 理解论文
溯源树
样例
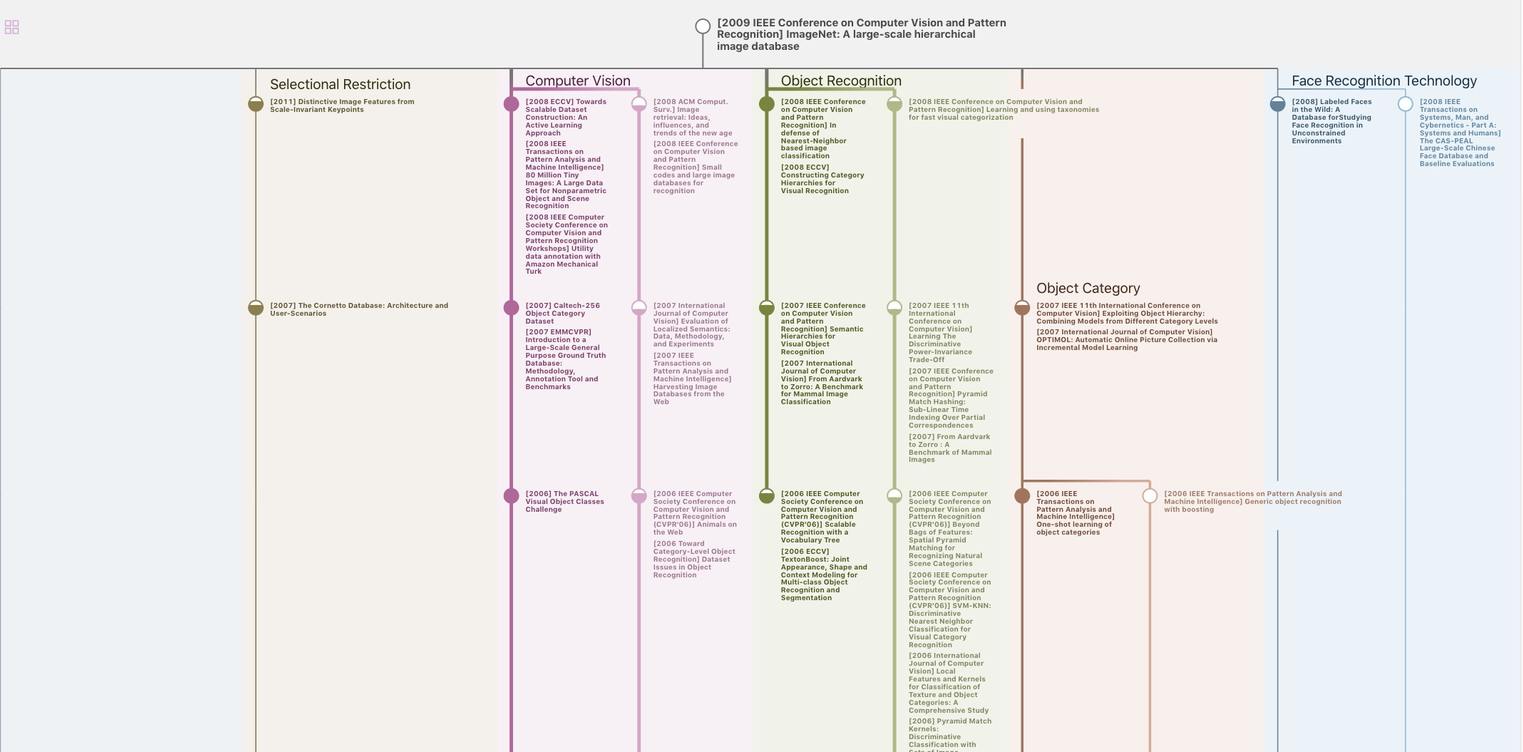
生成溯源树,研究论文发展脉络
Chat Paper
正在生成论文摘要