Adaptive Multi-modal Data Fusion Method for Dermatosis Diagnosis.
2023 IEEE International Conference on Bioinformatics and Biomedicine (BIBM)(2023)
摘要
Meta-data, e.g., gender, medical history, etc., is of vital importance for dermatosis diagnosis clinically, while most existing AI-based methods ignore this information and only employ dermoscopy images. Due to the modality disequilibrium of image and meta-data, there would be unequal optimization during the modal fusion, resulting in the weak meta-data modality being suppressed and losing their contributions to the classification. To address this issue, we propose an Adaptive Multi-modal Fusion (AMF) method for dermatosis diagnosis, which reweights the fusion factor of image and meta-data by evaluating their optimization discrepancy through the Euclidean norm. What’s more, the Co-Attention mechanism is employed to align the two modalities implicitly. To evaluate the effectiveness of the proposed method, extensive experiments are conducted on multi-modal dermatosis public datasets including ISIC 2019, PDA-UFES-20, and HAM10000. The results demonstrate that the proposed method can achieve better performance in comparison to the state-of-the-art methods.
更多查看译文
关键词
Dermatosis Diagnosis,Multi-modal Fusion,Transformer,Deep Learning
AI 理解论文
溯源树
样例
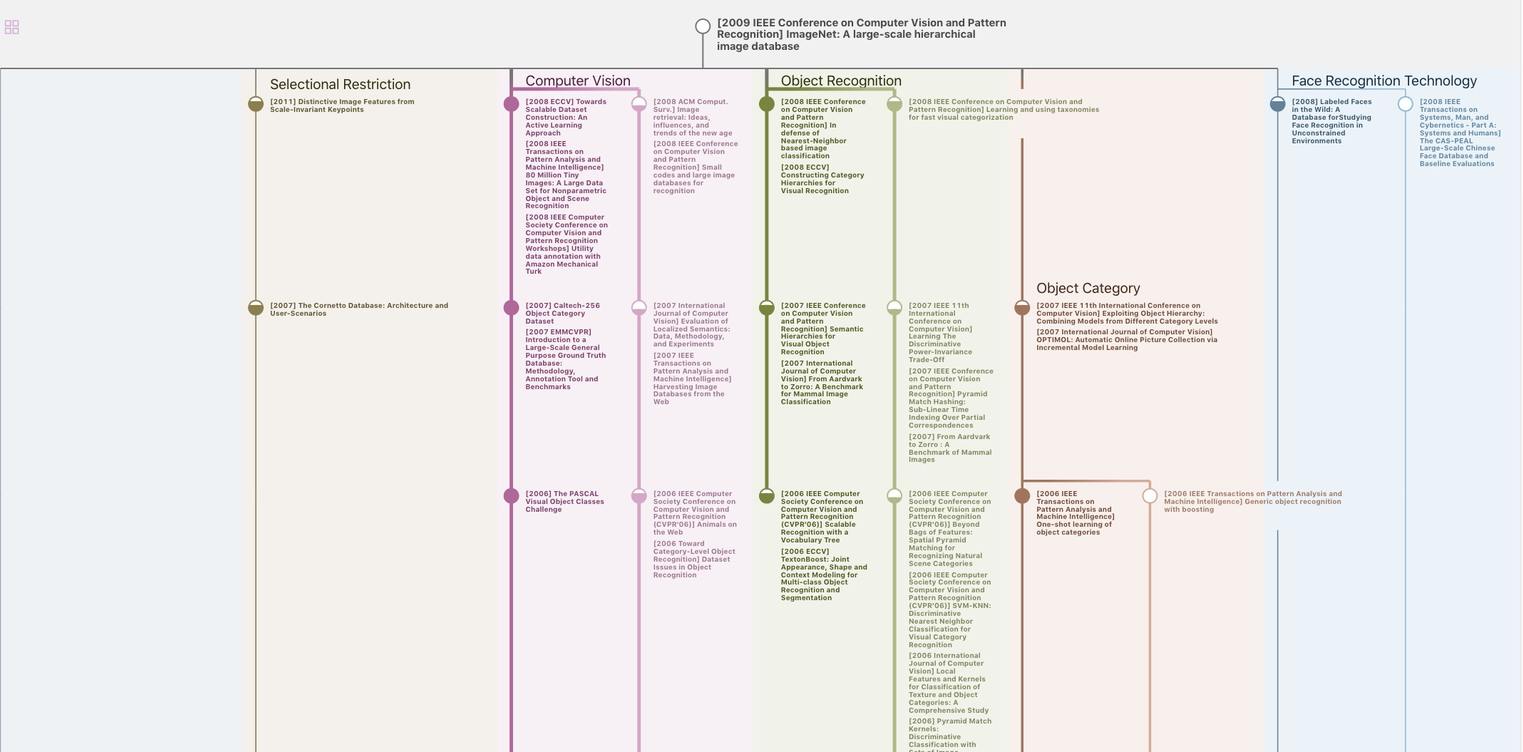
生成溯源树,研究论文发展脉络
Chat Paper
正在生成论文摘要