Biomarker Discovery Using Multimodal Data with the Potential Application in Lung Tumor Diagnosis
2023 IEEE International Conference on Bioinformatics and Biomedicine (BIBM)(2023)
关键词
biomarkers,machine learning,lung tumor diagnosis,multi-omics,multimodal
AI 理解论文
溯源树
样例
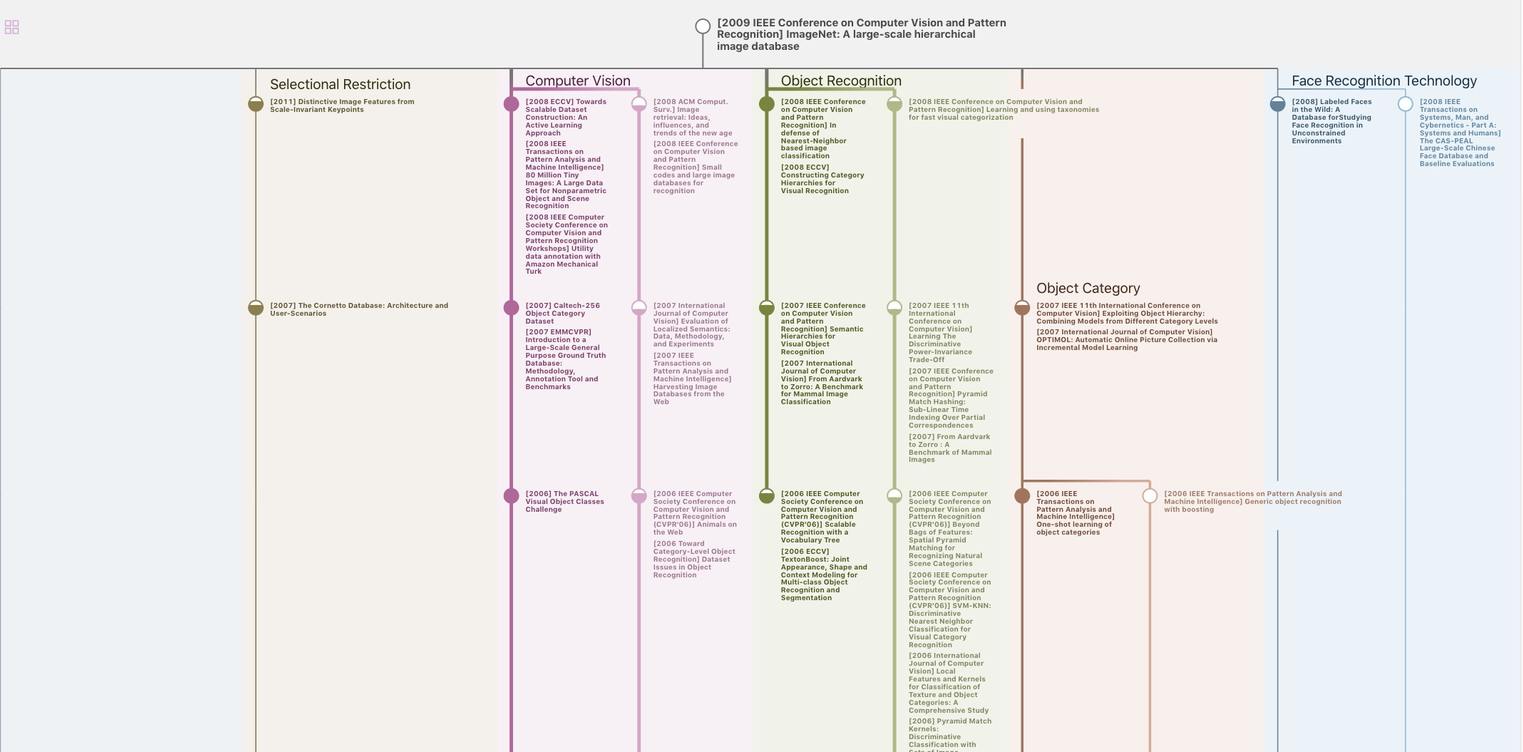
生成溯源树,研究论文发展脉络
Chat Paper
正在生成论文摘要