CTCM: Clustering based on three correlation matrices for multi-omics data integration and cancer subtype identification.
2023 IEEE International Conference on Bioinformatics and Biomedicine (BIBM)(2023)
摘要
Large-scale sequencing data is used by biologists to understand the biological systems and molecular mechanisms of disease. One challenge is to effectively use valuable information from different cancer omics to produce more accurate and reliable subtypes. We propose a clustering strategy based on three correlation matrices (CTCM) to identify cancer subtypes in multi-omics data. Connectivity matrix, similarity matrix and resampling matrix collect useful data information from different perspectives. The connection matrix divides the raw data into stable subtypes by adding noise to simulate the systematic error of the sequencing platform. The similar matrix use Gaussian kernel functions to construct connections between samples as the "skeleton" of the whole. The resampling matrix adapts to the explosive growth of data by sampling subsets. For each omics data, we combine the connectivity matrix and resampling matrix with the similarity matrix to generate an iterative version. Iterating the three relationship matrices for each omics produces a fusion matrix that is used for spectral clustering to identify cancer subtypes. Compared with six other state-of-the-art multi-omics clustering methods, CTCM achieves excellent performance on the benchmark data sets of simulation and TCGA databases. The method is general enough to replace existing unsupervised clustering techniques outside the scope of biomedical research to integrate multiple types of data.
更多查看译文
关键词
subtype discovery,multi-omics integration,correlation matrix,resample,perturbation clustering
AI 理解论文
溯源树
样例
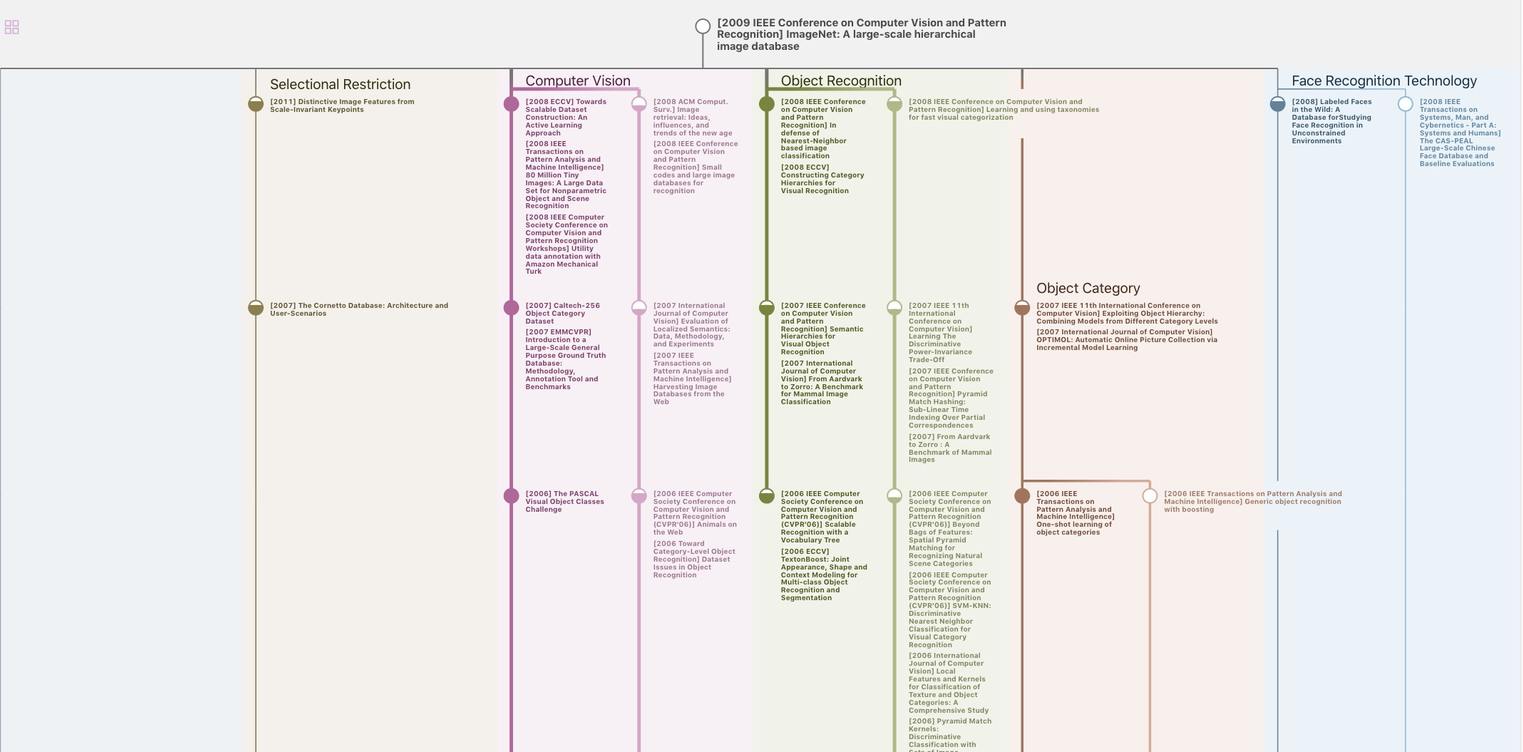
生成溯源树,研究论文发展脉络
Chat Paper
正在生成论文摘要