HyperScore: A unified measure to model hypertension progression using multi-modality measurements and semi-supervised learning.
2023 IEEE International Conference on Bioinformatics and Biomedicine (BIBM)(2023)
摘要
Hypertension is a serious medical condition that affects over a billion people worldwide. The proper management of disease progression requires an extended knowledge of the overall functional and structural changes in the whole body in response to the hypertension. Here, we propose HyperScore, an integrative and unified measure of hypertension progression relative to multi-organ and multi-modality clinical measurements and based on a semi-supervised machine learning (ML) approach. We developed the measure based on a large participating cohort from the UK Biobank database (n=27,099) with over 500 imaging and clinical variables from multiple modalities. The semi-supervised approach was developed based on the contrastive trajectory inference mechanism to provide a score that reflects the proximity of a participant to the disease state (range: 0–1). Modelling revealed that majority of hypertensive participants had scores above 0.25, whereas normotensives had scores below this threshold. The sensitivity and specificity were above 89%, with an area under the receiver operating characteristics of 96.4%. The modelling showed a stable performance when evaluating hidden testing sets on a 10-fold cross-validation scheme with nearly 0.1 error. There was a strong association (r
2
>0.6) between HyperScore and organs’ phenotypic patterns, especially for variables such as white matter hyperintensity and body mass index. This study is the first to potentiate ML-based modelling of hypertension progression from a multi-organ perspective, which could significantly aid in clinical decision making to save lives.
更多查看译文
关键词
Hypertension,blood pressure,machine learning,semi-supervised learning,contrastive PCA
AI 理解论文
溯源树
样例
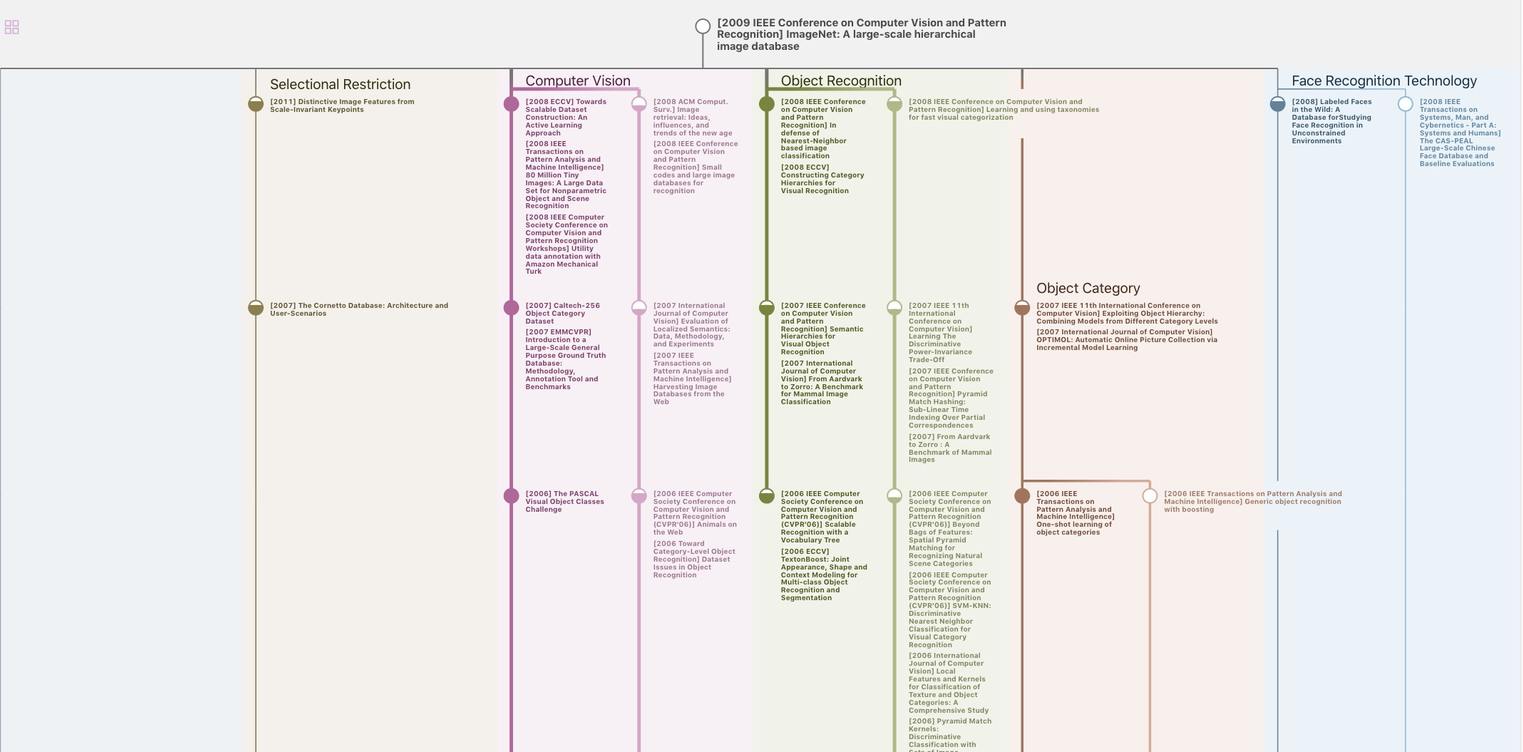
生成溯源树,研究论文发展脉络
Chat Paper
正在生成论文摘要