Medical Extractive Question-Answering Based on Fusion of Hierarchical Features.
2023 IEEE International Conference on Bioinformatics and Biomedicine (BIBM)(2023)
摘要
With the combination of natural language processing and artificial intelligence techniques, medical extractive question-answering (Q&A) provides valuable insights and assists medical professionals in daily work and scientific research, answering medical questions rapidly and accurately, thus holding significant practical significance. Therefore, research on medical extractive Q&A holds significant practical significance. However, the current state of medical extractive question-answering lacks attention to the interaction and prediction layers in the model structure. To address these issues, this paper proposes the Integrating pre-trained multi-layer structural feature information based Bio-BERT (IPMF-Bio-BERT) approach. This method leverages the rich word vector representations generated by the pre-trained Bio-BERT model, incorporating semantic and syntactic structural information to obtain multi-dimensional and complementary interactive feature information. Additionally, we introduce a flexible guidance network based on interactive information, which combines iterative and pointer network techniques to enhance the predictive performance of the question-answering model. We evaluate our proposed model on the specialized biomedical extractive question-answering BioASQ corpus. Experimental results demonstrate that the IPMF-Bio-BERT training strategy enhances the recognition and predictive capabilities of medical extractive Q&A, we establish new state-of-the-art results by outperforming existing approaches.
更多查看译文
关键词
Medical Extractive Question-Answering,Attention Mechanism,Flexible Guidance Network
AI 理解论文
溯源树
样例
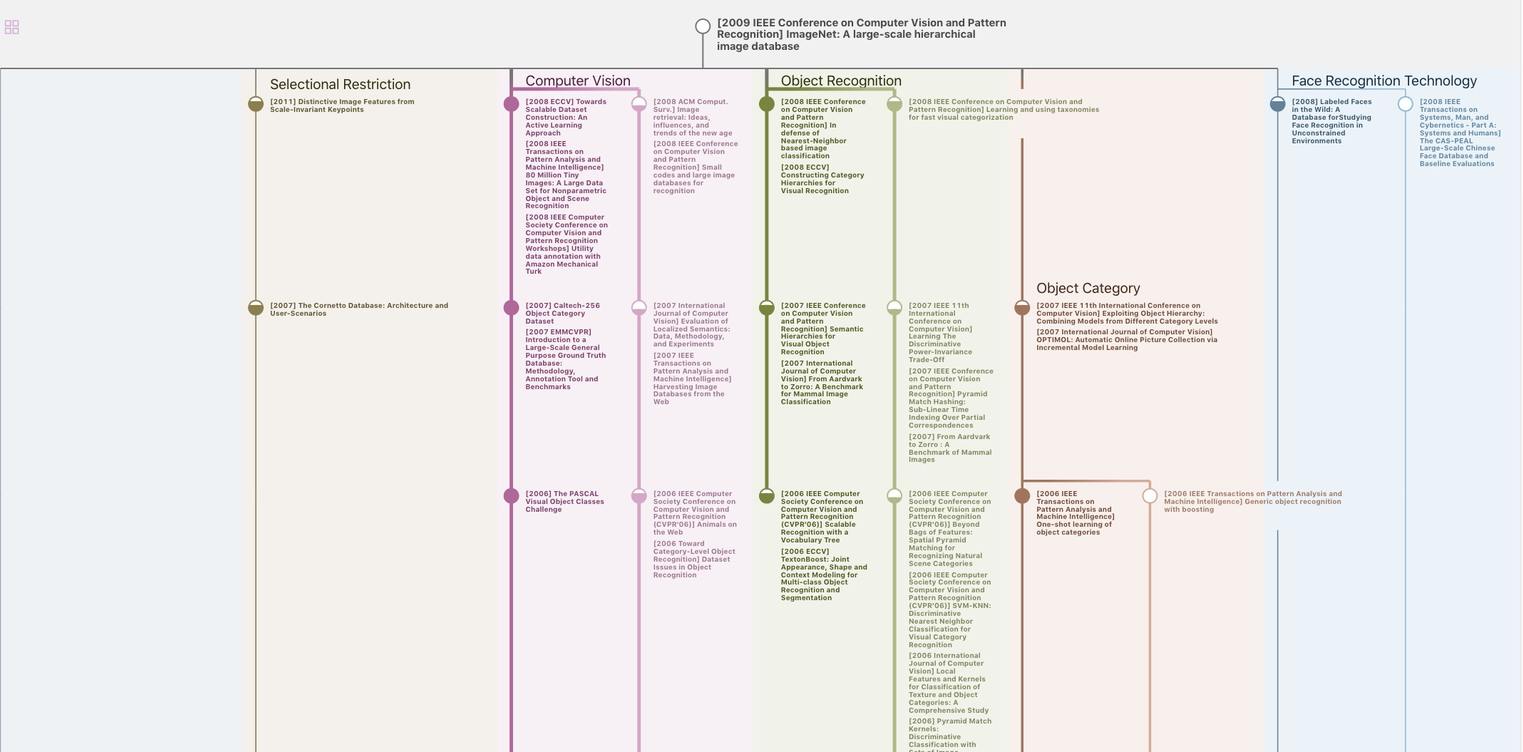
生成溯源树,研究论文发展脉络
Chat Paper
正在生成论文摘要