Keypoint-Driven Unsupervised Learning for Histopathology Image Registration.
2023 IEEE International Conference on Bioinformatics and Biomedicine (BIBM)(2023)
摘要
In this research, a new keypoint-centric training technique is presented, targeting efficient and precise unsupervised training of localized patch-based models applicable to histopathological whole slide image (WSI). The challenges imposed by the enormous gigapixel size of WSI and possible staining inconsistencies have historically hindered high-level training of local patch-based models. The method proposed here utilizes local patches focused around identified keypoints, thereby simplifying the training process for both local affine and non-rigid models. Separate networks were employed for training, and the method was assessed using local patch pairs from a dataset supplied by the University Hospital Frankfurt, consisting of 36 multi-stained histopathological WSIs. The subsequent experimental comparison shows that the keypoint-based approach enhances registration accuracy in both local affine and non-rigid contexts. These results affirm that the keypoint-oriented training method could serve as a significant step forward in improving the accuracy and efficiency of unsupervised learning within the intricate realm of histopathological image analysis, thus contributing to potential progress in image processing and medical diagnosis.
更多查看译文
关键词
Histopathological image,Affine transformation,Non-rigid registration,Unsupervised Learning,Keypoints
AI 理解论文
溯源树
样例
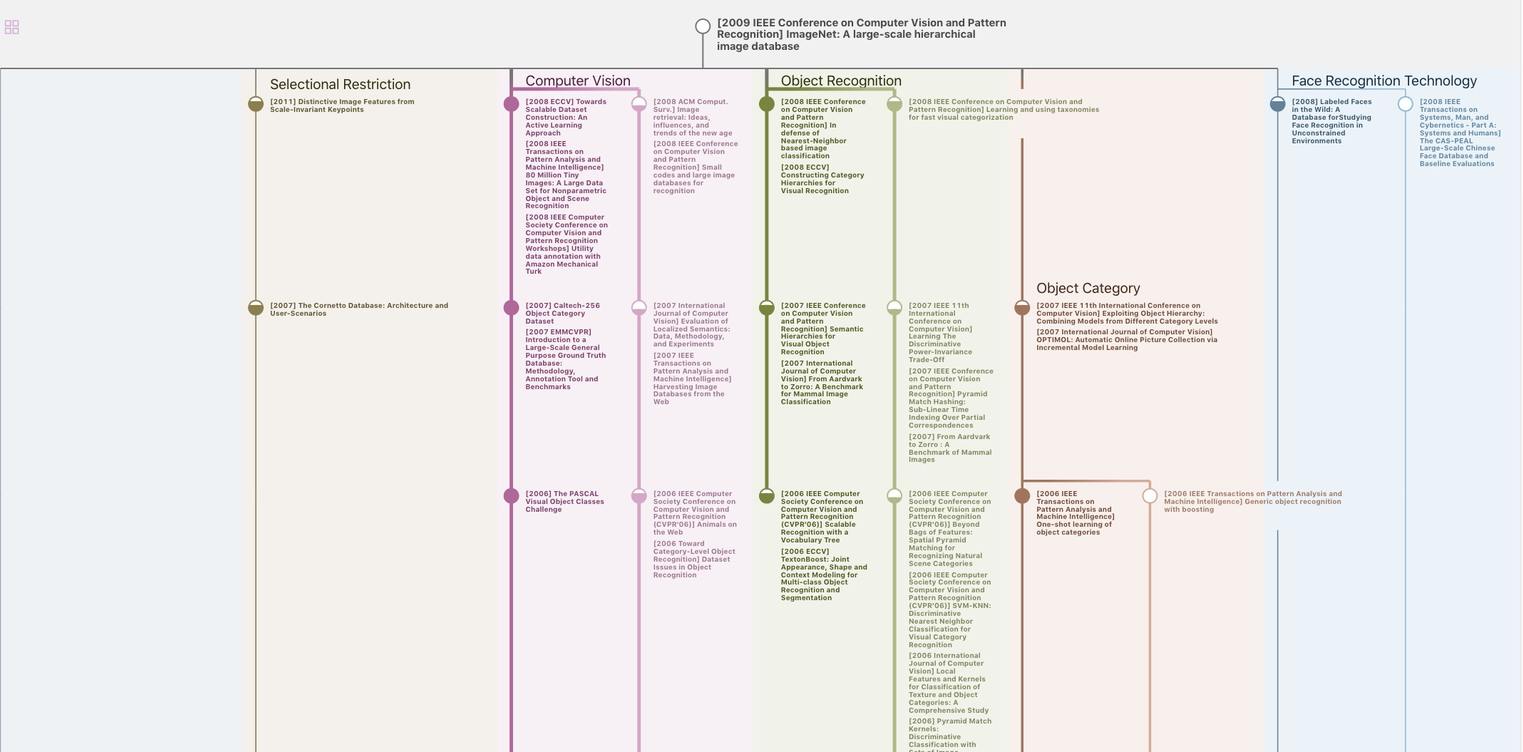
生成溯源树,研究论文发展脉络
Chat Paper
正在生成论文摘要