R2L-Net: Rapid Medical Image Segmentation Network Regularized by Self-Supervised Relative Localization Task.
2023 IEEE International Conference on Bioinformatics and Biomedicine (BIBM)(2023)
摘要
Medical image segmentation plays a crucial role in medical imaging, especially with advancements in techniques like magnetic resonance imaging (MRI) and computed tomography (CT). UNet, a widely used architecture, has shown promising results in medical image segmentation. Several variants based on UNet, and Transformer-based models, like TransUNet, have also exhibited potential for improving segmentation performance. However, these models often require substantial data and computational resources, making them less suitable for on-the-fly segmentation in medical scenarios. This paper proposes a fast medical image segmentation network called R2L-Net, which leverages self-supervised and supervised learning. R2L-Net introduces a self-supervised relative localization task as a regularization term during network training to enhance performance. Compared to UNeXt, our proposed R2L-Net achieves superior results on two public datasets (ISIC and BUSI), with an improved Intersection over Union (IoU) by 5.22 and 5.36, respectively. Moreover, R2L-Net offers several advantages over existing models, including a small number of parameters, low computational complexity, and fast image processing.
更多查看译文
关键词
medical image segmentation,self-supervised,bedside device,rapid
AI 理解论文
溯源树
样例
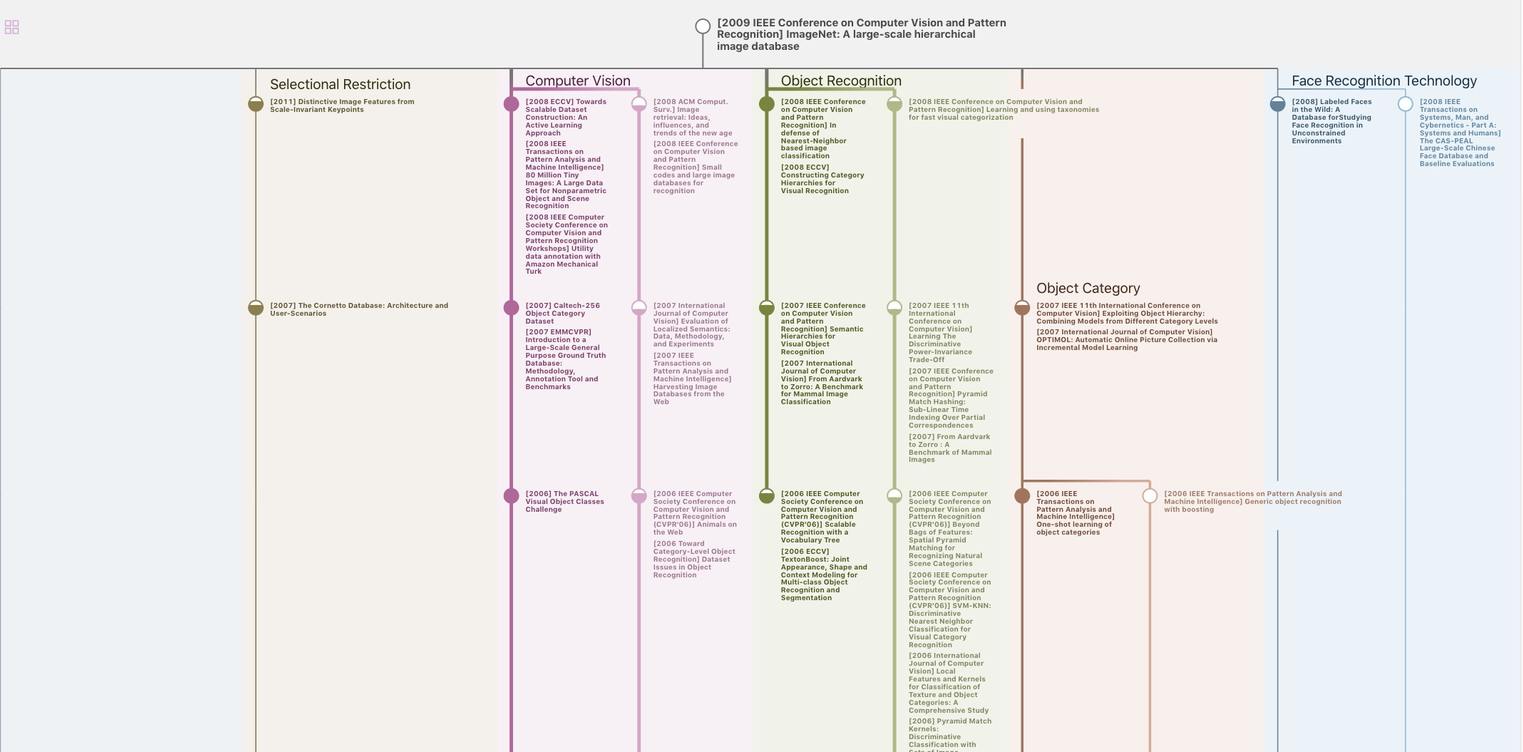
生成溯源树,研究论文发展脉络
Chat Paper
正在生成论文摘要