Implicit Feature Augmentation with Feature Transfer For Class-Imbalanced Medical Image Classification.
2023 IEEE International Conference on Bioinformatics and Biomedicine (BIBM)(2023)
摘要
The class imbalance problem, which is prevalent in medical image datasets, seriously affects the diagnostic effectiveness of deep learning-based network models. To alleviate this problem, data re-sampling and loss re-weighting techniques are often used to reshape the decision boundary of the classifier. However, these techniques still lead to biased decision boundary due to the lack of sufficient and diversified samples in the tail classes of medical image datasets. In this paper, we propose an implicit feature augmentation with feature transfer (FT-IFA) method which solves the class imbalance problem by expanding the feature space of tail classes to reshape the decision boundary of the classifier. FT-IFA utilizes prototype similarity to transfer the rich transformation information from the head classes to tail classes on the basis of the balanced feature space, enriching the intra-class diversity of tail classes. Experimental results on two class-imbalanced medical image datasets show that FT-IFA outperforms the current state-of-the-art methods and effectively solves the class imbalance problem.
更多查看译文
关键词
Medical image classification,Class imbalance,Feature transfer,Feature augmentation
AI 理解论文
溯源树
样例
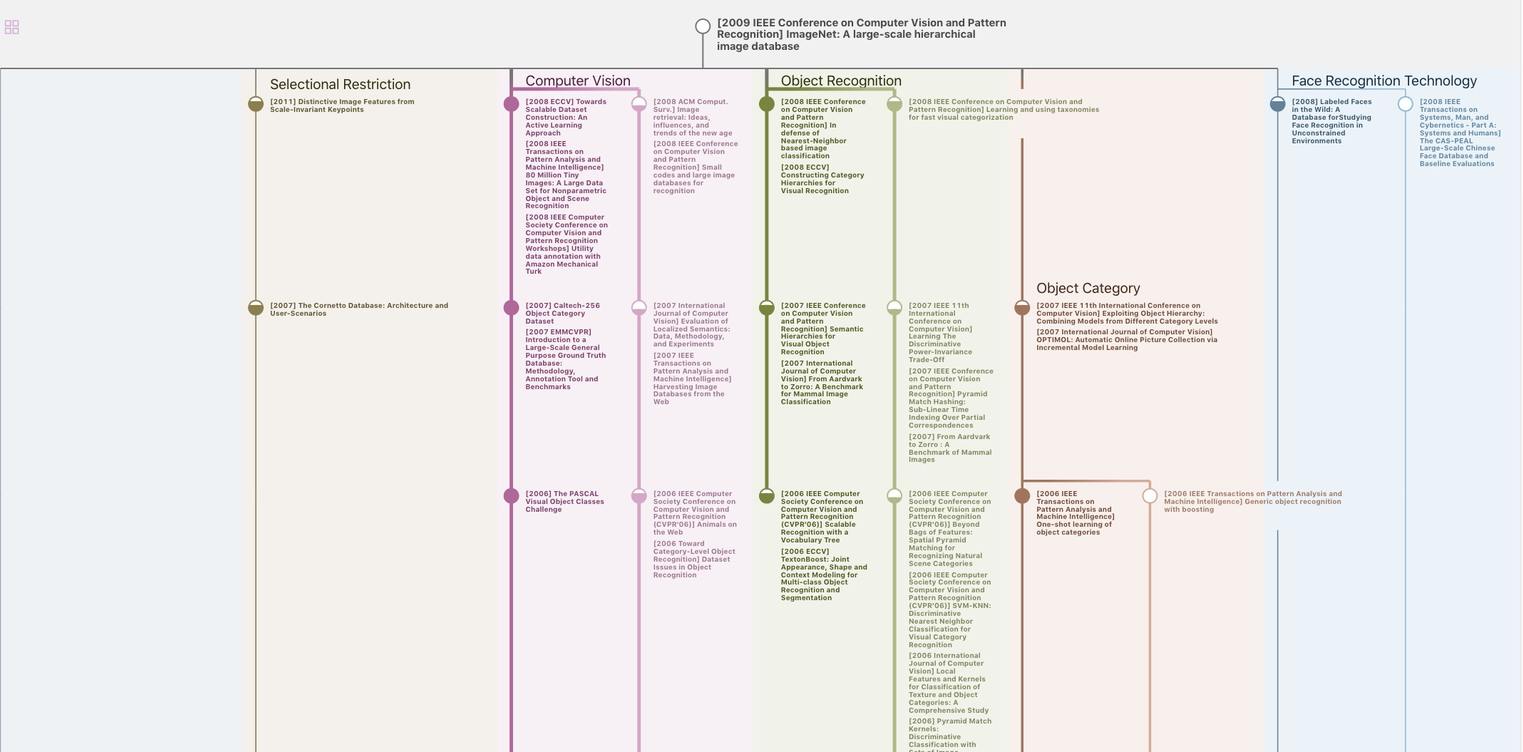
生成溯源树,研究论文发展脉络
Chat Paper
正在生成论文摘要