Spectral clustering based on multi-similarity learning method for single-cell RNA-seq data.
2023 IEEE International Conference on Bioinformatics and Biomedicine (BIBM)(2023)
摘要
The inherent complexities of single-cell RNA-seq data (scRNA-seq), such as high dimensionality, low signal-to-noise ratio, cellular heterogeneity, and imbalanced distribution of subcellular types, pose significant challenges when conducting cell type analysis. To address these obstacles, employing appropriate data preprocessing techniques in the single-cell clustering process is crucial, and spectral clustering is particularly effective due to its robustness to noise and outliers. Therefore, this paper presents a novel spectral clustering algorithm based on multi-similarity learning method (MSSC). However, utilizing a pairwise strategy to assess the similarity between two data points in conventional spectral clustering results in an insufficient representation of the intricate relationships within the dataset. In light of this issue, the proposed algorithm employs two similarity measurement methods, namely Euclidean distance and Spearman rank correlation coefficient, to obtain similarity matrices. These matrices are then fused for use in spectral clustering. Additionally, prior to performing spectral clustering, the scRNA-seq data is preprocessed using the Sigmoid kernel similarity method and normalization techniques. As a consequence, our method yields a more extensive and intricate dataset similarity information, thereby enhancing the performance of spectral clustering. Finally, the Gaussian mixture model (GMM) is used for clustering. In most cases, experiments validated that the MSSC method outperforms the other four clustering methods on seven benchmark scRNA-seq datasets.
更多查看译文
关键词
single-cell RNA-seq data,spectral clustering,Sigmoid kernel similarity method,Spearman rank correlation coefficient,Gaussian mixture model
AI 理解论文
溯源树
样例
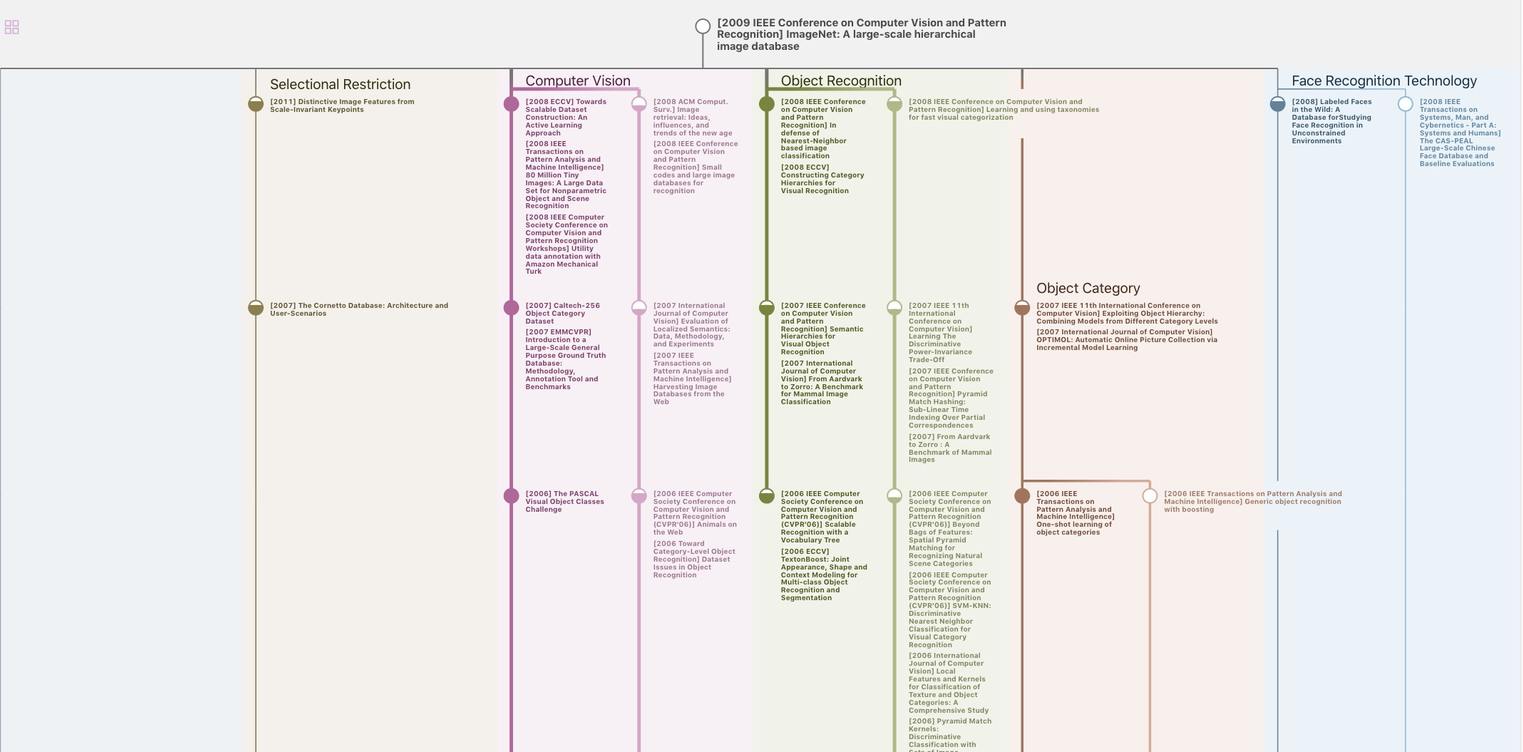
生成溯源树,研究论文发展脉络
Chat Paper
正在生成论文摘要