CARE-30: A Causally Driven Multi-Modal Model for Enhanced 30-Day ICU Readmission Predictions.
2023 IEEE International Conference on Bioinformatics and Biomedicine (BIBM)(2023)
摘要
Accurate prediction of unplanned readmissions allows healthcare systems to adopt preventive measures, reducing these occurrences. Creating a model that accurately predicts readmissions while simultaneously providing insights that are clinically interpretable is a complex and demanding task. This interpretability is vital, as it informs clinicians about the underlying reasons behind a model’s decisions, thus aiding in more informed clinical decision-making. In light of this, we introduce CARE-30 (Causal Analysis based Readmission Estimator within a 30-day time frame), a novel causal inference-based model, designed with clear cause-and-effect relationships, enhancing its potential acceptance and understanding among clinicians.In our approach, we generate a directed acyclic graph (DAG) to elucidate the latent causal relationships among pivotal clinical variables. Leveraging this causal graph, we can effectively apply the front-door criterion to construct the model and eliminate bias. By utilizing transformers, we integrate clinical texts, time-series data and categorical data into patient representation data as a robust input to the CARE-30 model. Our work signifies the effective application of knowledge from the field of causal inference in the domain of clinical medicine, and it also reflects the originality and impact of our research. CARE-30 unites causality and clinical prediction, unlocking new vistas in healthcare forecasting. Experiments demonstrated that our model outperforms all other methods in Accuracy and F1 scores.
更多查看译文
关键词
Causal inference,readmission prediction,multi-modal,MIMIC-III
AI 理解论文
溯源树
样例
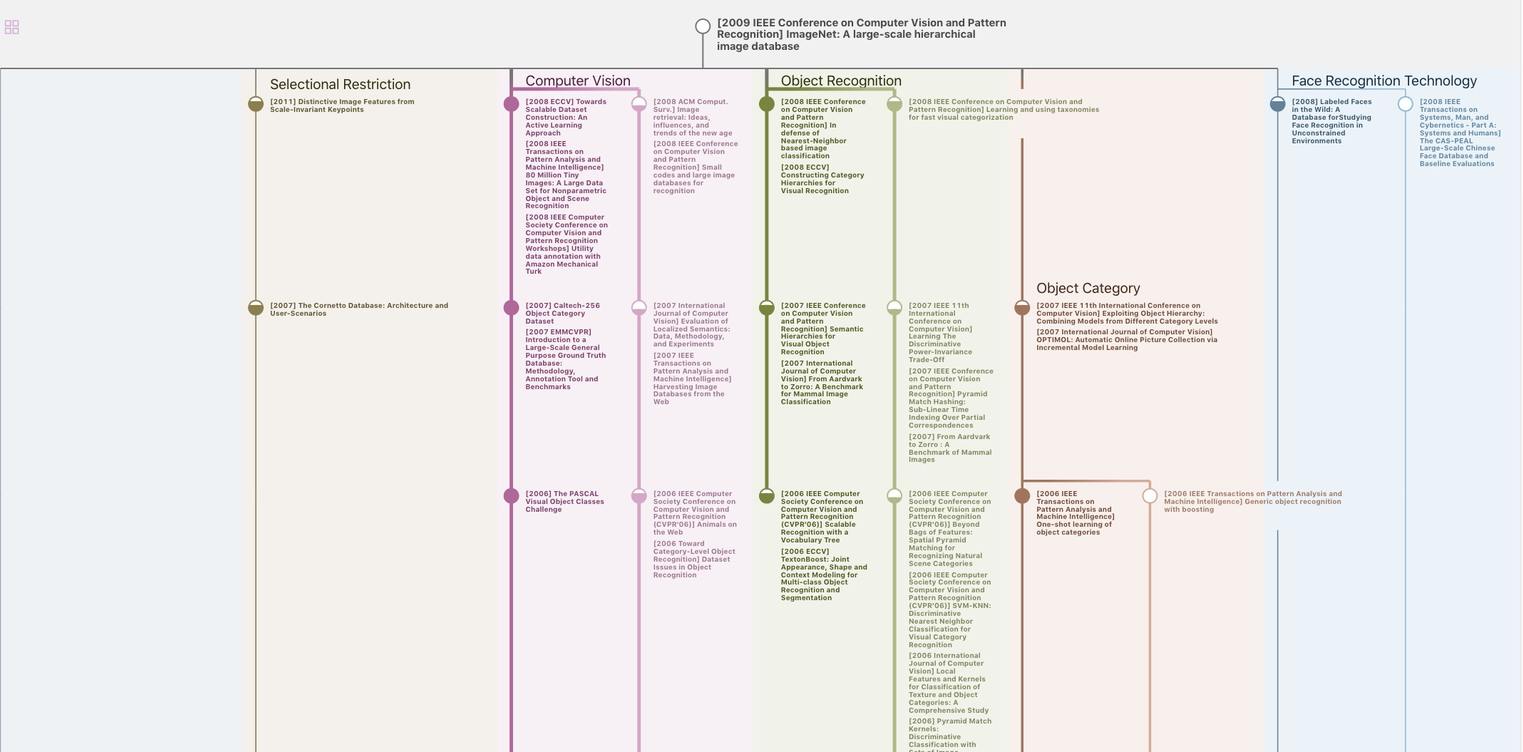
生成溯源树,研究论文发展脉络
Chat Paper
正在生成论文摘要