TGAAL: Combining Transformer-based GAN and Active Learning to Identify the Coding Potential of Sorfs in Plant Lncrnas
2023 IEEE International Conference on Bioinformatics and Biomedicine (BIBM)(2023)
Key words
Transformer,Generative Adversarial Network,Active Learning,LncRNA,sORFs
AI Read Science
Must-Reading Tree
Example
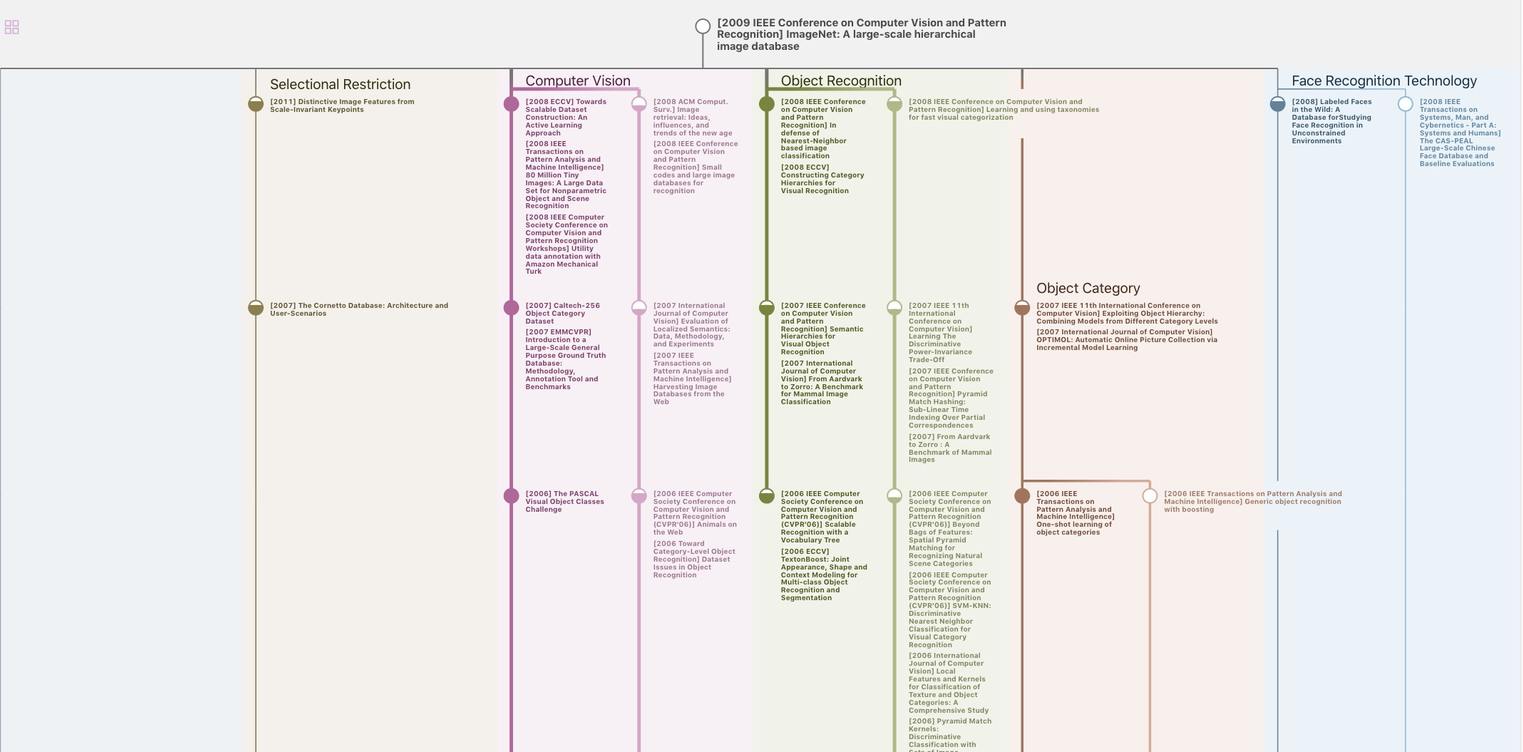
Generate MRT to find the research sequence of this paper
Chat Paper
Summary is being generated by the instructions you defined