Self organizing optimization and phase transition in reinforcement learning minority game system
Frontiers of Physics(2024)
摘要
Whether the complex game system composed of a large number of artificial intelligence (AI) agents empowered with reinforcement learning can produce extremely favorable collective behaviors just through the way of agent self-exploration is a matter of practical importance. In this paper, we address this question by combining the typical theoretical model of resource allocation system, the minority game model, with reinforcement learning. Each individual participating in the game is set to have a certain degree of intelligence based on reinforcement learning algorithm. In particular, we demonstrate that as AI agents gradually becomes familiar with the unknown environment and tries to provide optimal actions to maximize payoff, the whole system continues to approach the optimal state under certain parameter combinations, herding is effectively suppressed by an oscillating collective behavior which is a self-organizing pattern without any external interference. An interesting phenomenon is that a first-order phase transition is revealed based on some numerical results in our multi-agents system with reinforcement learning. In order to further understand the dynamic behavior of agent learning, we define and analyze the conversion path of belief mode, and find that the self-organizing condensation of belief modes appeared for the given trial and error rates in the AI system. Finally, we provide a detection method for period-two oscillation collective pattern emergence based on the Kullback–Leibler divergence and give the parameter position where the period-two appears.
更多查看译文
关键词
oscillatory evolution,collective behaviors,phase transition,reinforcement learning,minority game
AI 理解论文
溯源树
样例
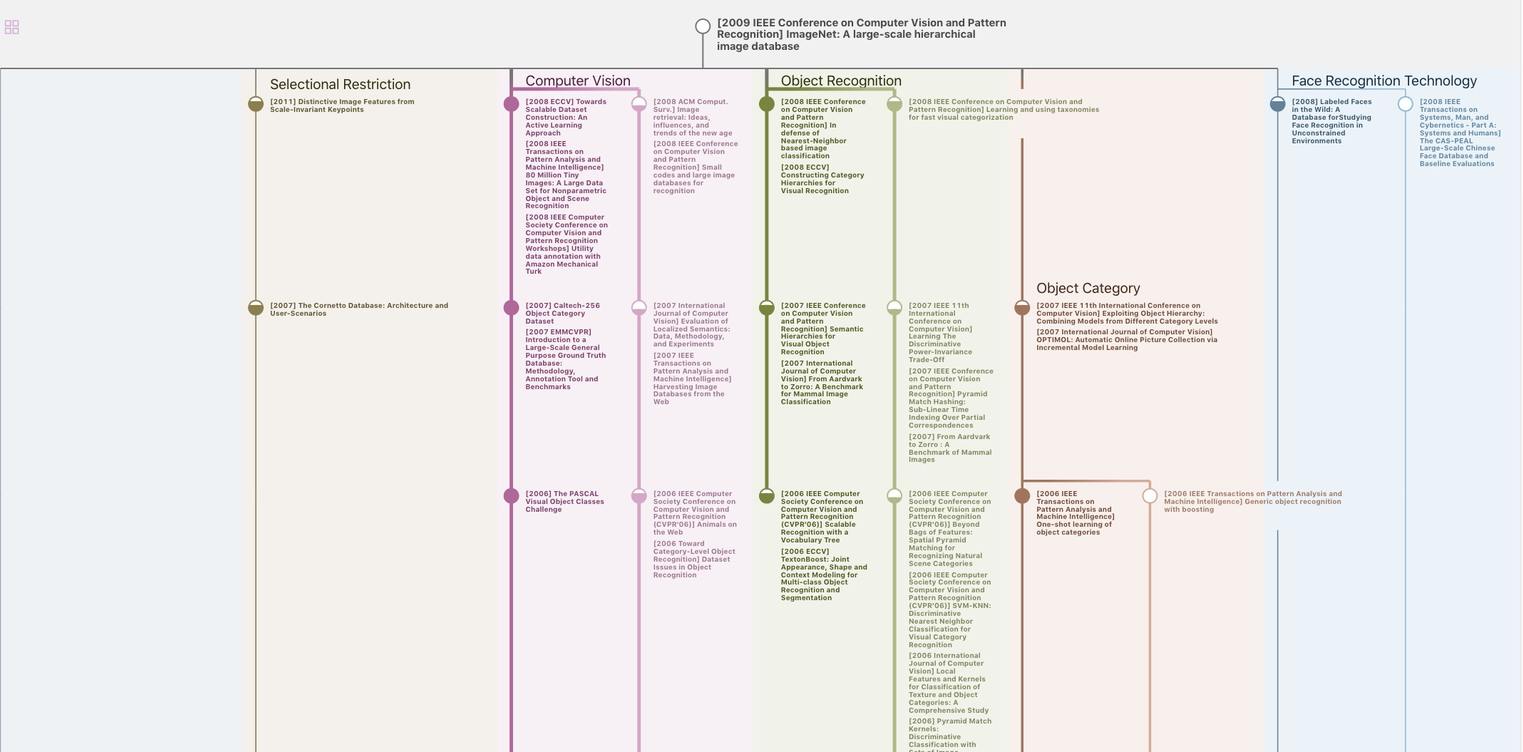
生成溯源树,研究论文发展脉络
Chat Paper
正在生成论文摘要