Analysis of incompressible viscous fluid flow in convergent and divergent channels with a hybrid meta-heuristic optimization techniques in ANN: An intelligent approach
Journal of Central South University(2023)
摘要
In this research article, we introduce a numerical investigation through artificial neural networks (ANN) integrated with evolutionary algorithm especially Archimedean optimization algorithm (AOA) hybrid with the water cycle algorithm (WCA) to address and enhance the analysis of the non-linear magneto-hydrodynamic (MHD) Jeffery-Hamel problem, especially stretching/shrinking in convergent and divergent channel. This combined technique is referred to as ANN-AOA-WCA. The complex nonlinear magneto-hydrodynamic Jeffery-Hamel problem based partial differential equations are transformed into non-linear system of ordinary differential equations for velocity and temperature. We formulate the ANN based fitness function to find the solution of non-linear differential. Subsequently, we employ a novel hybridization of AOA and WCA (AOA-WCA) to optimize the ANN based fitness function and identify the best optimal weights and biases for ANN. To demonstrate the effectiveness and versatility of our proposed hybrid method, we explore MHD models across a range of Reynolds numbers, channel angles and stretchable boundary value leading to the development of two distinct cases. ANN-AOA-WCA numerical results closely align with reference solutions (NDSOLVE) and the absolute error between NDSOLVE and ANN-AOA-WCA is up to 3.35 × 10 −8 , particularly critical to the understanding of stretchable convergent and divergent channel. Furthermore, to validate the ANN-AOA-WCA technique, we conducted a statistical analysis over 150 independence runs to find the fitness value.
更多查看译文
关键词
ANN,Archimedes optimization,water cycle algorithm,stretching/shrinking surface,convergent/divergent channel,人工神经网络,阿基米德优化,水循环算法,拉伸/收缩表面,收敛或发散通道
AI 理解论文
溯源树
样例
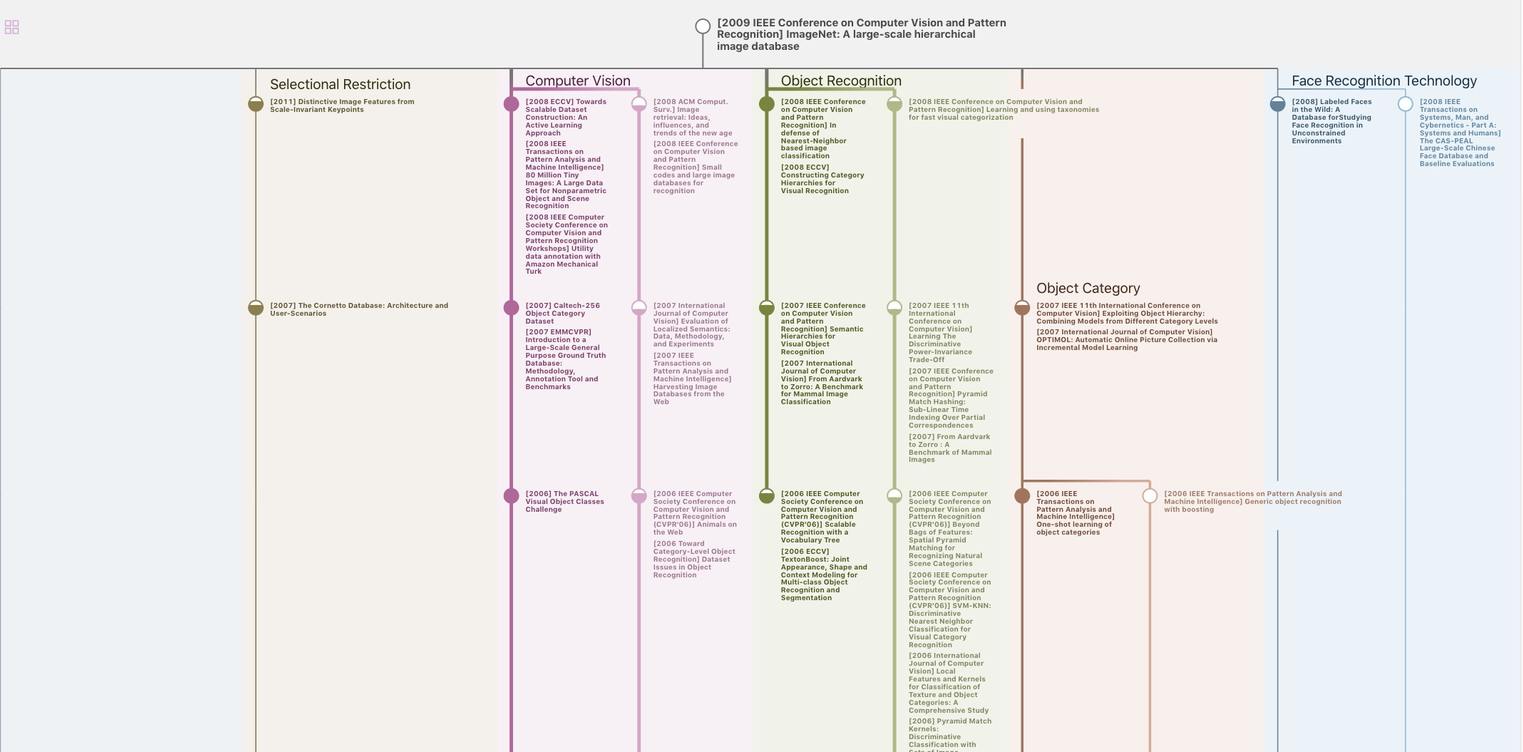
生成溯源树,研究论文发展脉络
Chat Paper
正在生成论文摘要