Artificial Intelligence Applications in Road Traffic Forecasting: A Review of Current Research
2023 International Conference on Innovation and Intelligence for Informatics, Computing, and Technologies (3ICT)(2023)
摘要
Artificial intelligence (AI) is revolutionizing data processing and analysis due to its exceptional capability to detect and forecast future trends. This makes it an obvious alternative to traditional methods of traffic demand forecast, which is the core element of transportation planning. While AI real-time and short-term traffic forecasts application has been widely investigated, long-term applications are notably limited. This paper examines the current state of AI in traffic forecasting, outlining both its challenges and opportunities. Most AI applications in traffic forecasting related research focus on single-point analysis methods, lacking a broader spatio-temporal context. To fully leverage AI's capabilities, it's important to address the gap in understanding how spatial attributes affect traffic behaviour. While the choice of input variables, analysis methods, and model structures significantly impacts the effectiveness of traffic forecasts, data validation is another crucial aspect of the effective application of AI methods. Knowledge-based systems are effective for data analysis, modelling, and multi-criteria decision-making. Neural networks are increasingly vital for traffic control and route guidance. Two AI techniques have shown promise in traffic forecasting: Back Propagation Neural Network functions (BPNN) and Agent-Based Modelling and Simulation (ABMS). BPNN excels in capturing both linear and nonlinear time-series traffic data with multi-layer models. ABMS, a newer approach, divides the transportation system into interacting agents, providing a holistic view of traffic demand dynamics. AI's data-driven approaches often lack context, particularly in understanding spatial parameters, which limits their applicability in long-term forecasts. To make AI more competitive with traditional macro-modelling in transportation planning, new methods are needed to integrate spatial and temporal data effectively.
更多查看译文
关键词
Road traffic forecasting,Transportation Planning,AI Modelling,Machine Learning,Agent-Based Modelling,Back Propagation Neural Network
AI 理解论文
溯源树
样例
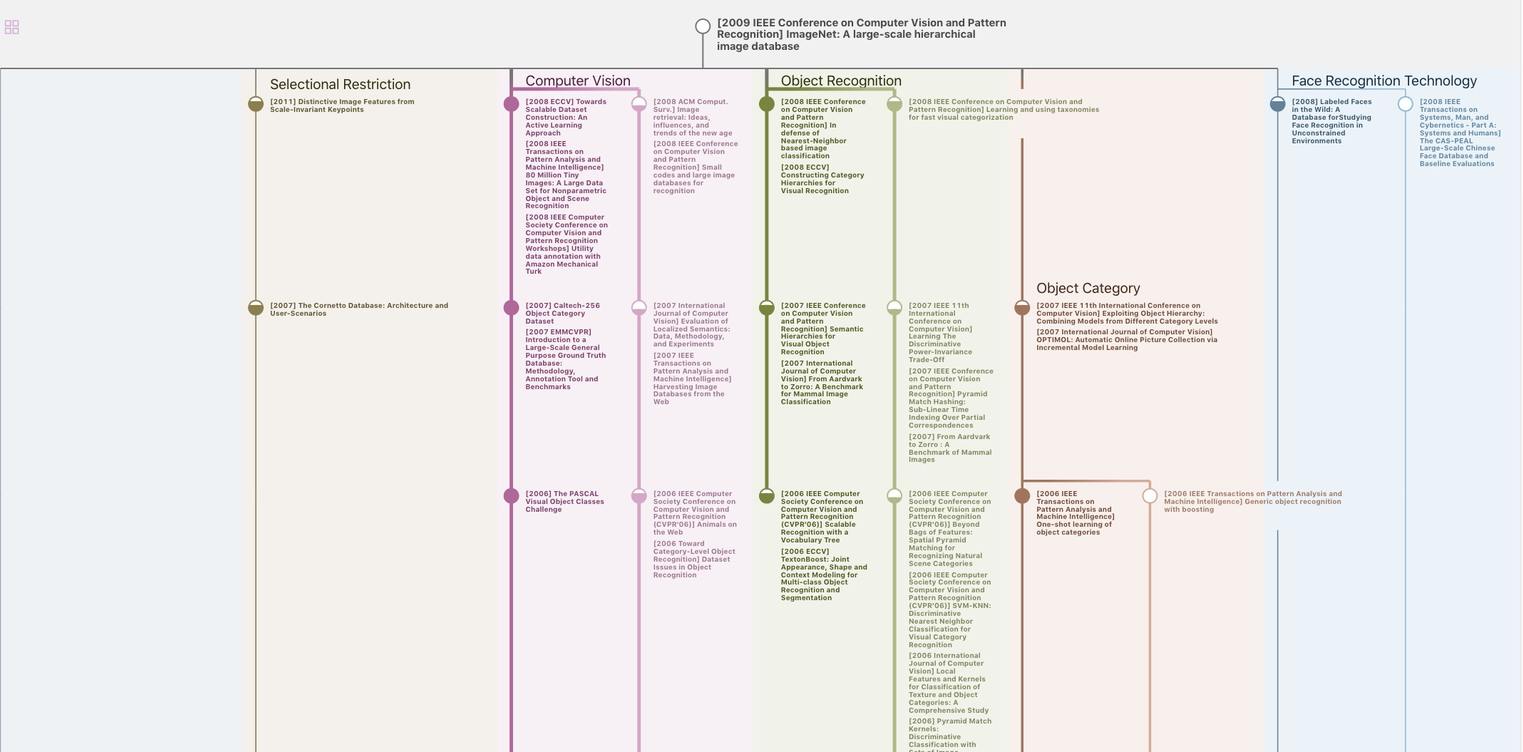
生成溯源树,研究论文发展脉络
Chat Paper
正在生成论文摘要