A multi-level semantic-assisted unsupervised heterogeneous network representation learning model
NEUROCOMPUTING(2024)
摘要
Heterogeneous networks, which model various real -world scenarios, have gained significant attention in recent years due to their rich semantic information. Existing heterogeneous network representation models often focus on capturing semantic information through meta -paths, but they overlook the semantic information of higher -order neighborhoods based on meta -paths and the clusters to which nodes belong. Moreover, while many existing methods perform well with labeled data, unsupervised network representation learning becomes essential for dealing with large amounts of unlabeled data in practical applications. To address these issues, this paper proposes an unsupervised heterogeneous network representation learning model with multi -level semantic assistance. The model leverages mutual information maximization to construct a loss function for unsupervised learning. It captures first -level semantics of nodes through graph convolutional networks and meta -path fusion mechanisms to create initial node representations. Then, it utilizes mutual information to acquire second -level semantics, i.e., higher -order neighborhood information based on metapaths, by maximizing the mutual information between the initial node representations and the global graph representation based on meta -paths. Additionally, a cluster -aware mechanism is designed to capture thirdlevel semantic information by maximizing the mutual information between the initial node representation and the representation of the cluster to which the node belongs, considering the cluster information of the node. Comprehensive experiments on three real datasets demonstrate the effectiveness and efficiency of the proposed method in node classification and clustering tasks. Moreover, the method exhibits better robustness when the network is destroyed to some extent.
更多查看译文
关键词
Heterogeneous networks,Representation learning,Mutual information,Cluster awareness
AI 理解论文
溯源树
样例
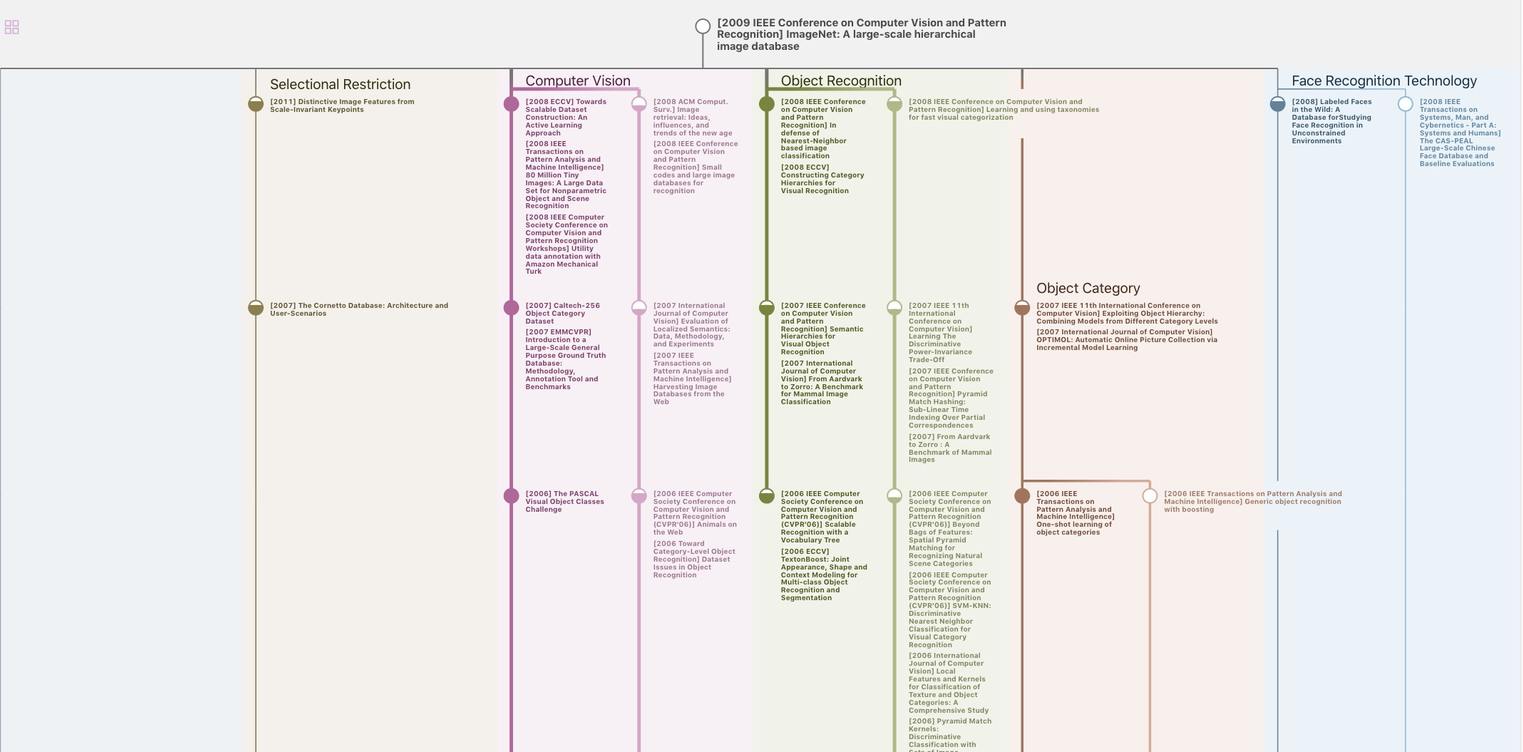
生成溯源树,研究论文发展脉络
Chat Paper
正在生成论文摘要