Semi-Supervised Semantic Segmentation in Remote Sensing Images Using Active Learning and Deep Global Second Order Pooling Network
2023 20th International Computer Conference on Wavelet Active Media Technology and Information Processing (ICCWAMTIP)(2023)
摘要
Training convolutional neural networks (CNNs) for semi-semantic segmentation in remote sensing images is difficult due to the substantial amount of labelled data required. The scarcity of dense pixel-level annotations hampers the model's ability to extract meaningful information from the images. We propose a novel semi-supervised semantic segmentation network called 3S-Net, designed explicitly for high-resolution remote sensing imagery to overcome this challenge. This network leverages a limited number of labelled examples and many unlabeled examples to generate pseudo-labels, expanding the labelled training set. The Deep Global Second-Order Pooling Network (DG2oPN) is employed to learn discriminative and representative features from the entire image information throughout the network. Our approach involves two main steps: First, an active learning-based sampling strategy is utilized to select a representative set of labelled data. Secondly, we introduce the 3S-Net, which learns from limited annotated data and effectively leverages unlabeled images by utilizing the second-order statistics of the entire image. Experimental results on two public remote sensing datasets demonstrate that our semi-supervised semantic model efficiently and effectively addresses the issue of limited labelled samples, outperforming other state-of-the-art methods.
更多查看译文
关键词
Semantic segmentation,Second-order Pooling,Semi-supervised learning,Convolutional neural networks,Unlabeled data,Generative adversarial networks
AI 理解论文
溯源树
样例
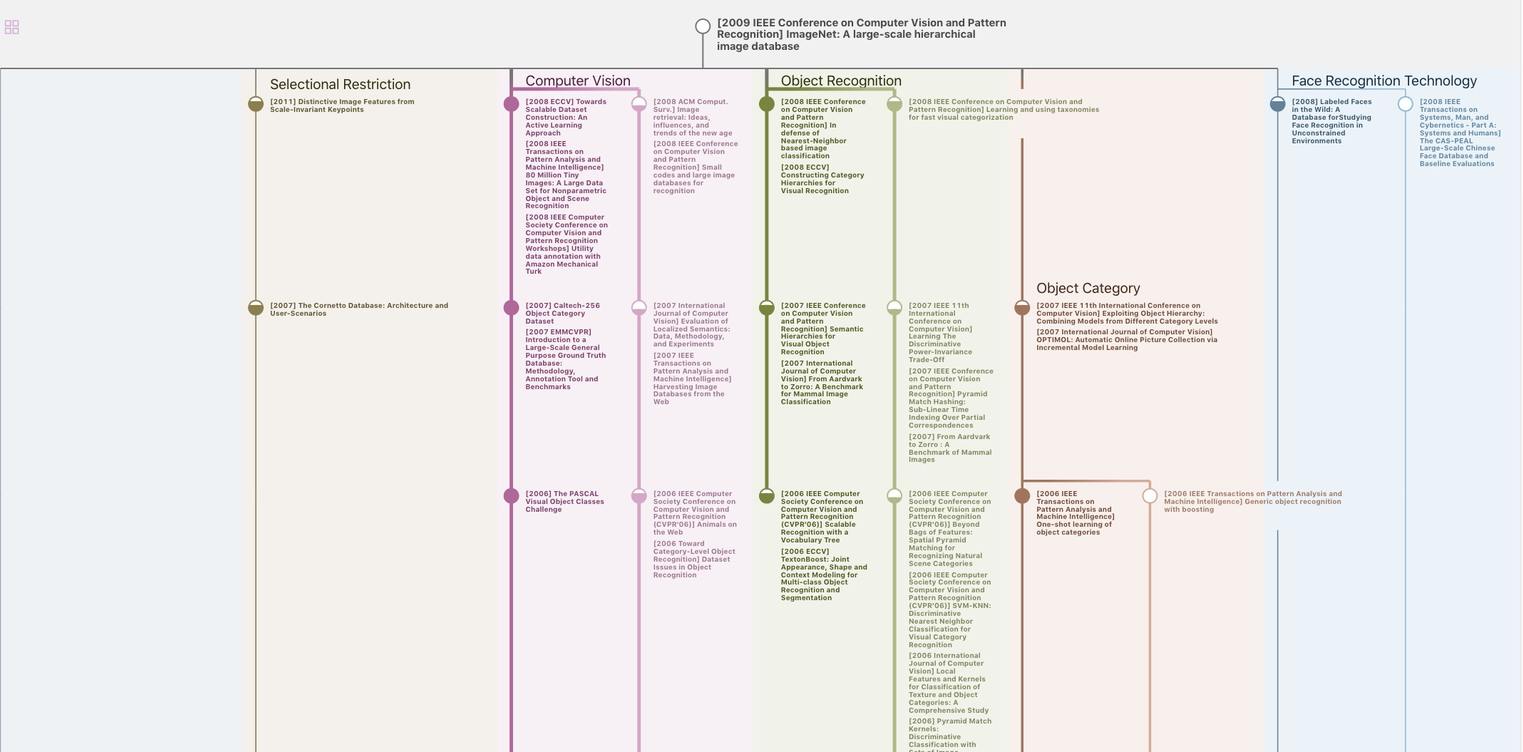
生成溯源树,研究论文发展脉络
Chat Paper
正在生成论文摘要