Detect the Unseen: An Expandable Detection Model for Stem Cell Images.
2023 IEEE International Conference on Bioinformatics and Biomedicine (BIBM)(2023)
摘要
Stem cell culture in vitro is essential for research in cell biology, drug toxicity and translational studies. In recent years, there has been a surge in the development of deep learning-based object detection algorithms tailored for image analysis of stem cell culture. However, many of these algorithms fall short in terms of performance and interpretability. To address these challenges, we present StemCellDet, an innovative multimodal-based method for stem cell detection. By harnessing the power of the pretrained CLIP model, StemCellDet uniquely encodes descriptions of stem cell categories into text embeddings, which are then synchronized with image embeddings. This synchronization enhances the model’s ability to identify critical features for accurate stem cell model categorization. Furthermore, by integrating knowledge distillation and introducing our proposed Semantic Fusion Module (SFM), StemCellDet can adeptly identify stem cell culture categories that were not present during its training phase using only their textual descriptions. Our experiments highlight StemCellDet’s robust detection capabilities and its advantages in terms of accuracy and generalizability.
更多查看译文
关键词
Stem Cell Image,Object Detection,Multimodal,CLIP,Generalization
AI 理解论文
溯源树
样例
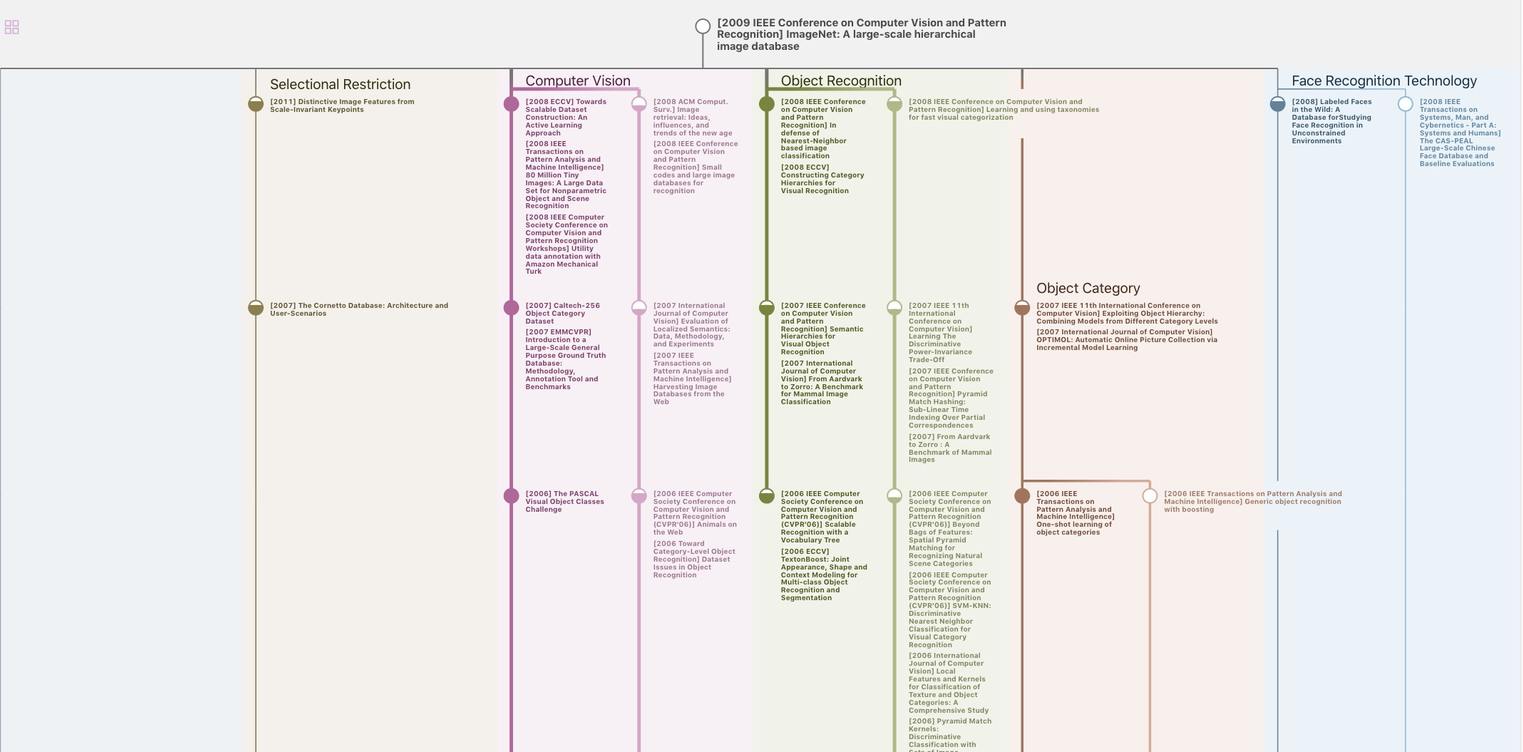
生成溯源树,研究论文发展脉络
Chat Paper
正在生成论文摘要