Unsupervised Feature Selection by Fusing Spectral Clustering and Locality Preserving Projection.
2023 IEEE International Conference on Bioinformatics and Biomedicine (BIBM)(2023)
摘要
Due to feature redundancy in high dimensional data, the unsupervised feature selection methods for dimension reduction have attracted considerable attention. The current feature selection frameworks consider the global information of data, but ignore mutual screening of global and local information, and there have been no important breakthroughs on this approach research recently. We propose a novel feature selection method based on iterative optimization between the pseudo label matrix from spectral clustering and the local projection information (SNUFS), and then prove the convergence of the method. The pseudo label matrix and the local projection are designed in objective function for mutual screening and guiding the regularization feature selection by iterative approach. Our method selects features most relevant to the pseudo label and preserves the local structure of original data from feature selection matrix, where alternate iteration of different optimization items including pseudo label matrix achieve mutual screening. For this method, we give the objective function, iterative optimization fusion approach and convergence analysis in detail. Furthermore, we use K-Nearest Neighbor (KNN) and K-means to implement locality preserving projection and obtain two specific algorithms. Experiments on four real-world datasets in different fields demonstrate that our algorithms can effectively improve the accuracy of feature selection. In particular, our algorithm of KNN implementation is more effective and outperforms other major algorithms.
更多查看译文
关键词
Unsupervised feature selection,Locality preserving projection,Spectral Clustering,K-Nearest Neighbor,Fusion approach
AI 理解论文
溯源树
样例
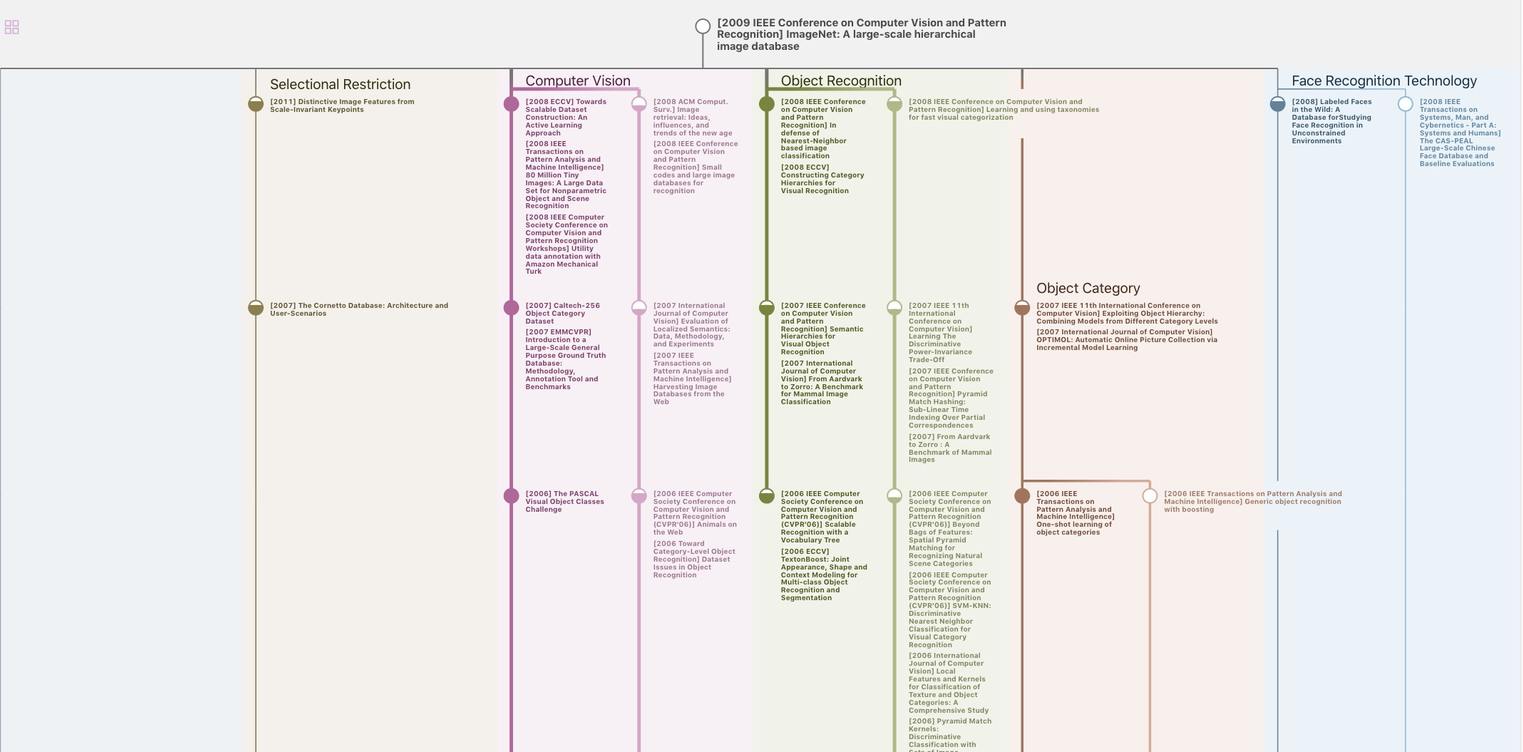
生成溯源树,研究论文发展脉络
Chat Paper
正在生成论文摘要