Optimization model based on attention mechanism for few-shot image classification
Machine Vision and Applications(2024)
摘要
Deep learning has emerged as the leading approach for pattern recognition, but its reliance on large labeled datasets poses challenges in real-world applications where obtaining annotated samples is difficult. Few-shot learning, inspired by human learning, enables fast adaptation to new concepts with limited examples. Optimization-based meta-learning has gained popularity as a few-shot learning method. However, it struggles with capturing long-range dependencies of gradients and has slow convergence rates, making it challenging to extract features from limited samples. To overcome these issues, we propose MLAL, an optimization model based on attention for few-shot learning. The model comprises two parts: the attention-LSTM meta-learner, which optimizes gradients hierarchically using the self-attention mechanism, and the cross-attention base-learner, which uses the cross-attention mechanism to cross-learn the common category features of support and query sets in a meta-task. Extensive experiments on two benchmark datasets show that MLAL achieves exceptional 1-shot and 5-shot classification accuracy on MiniImagenet and TiredImagenet. The codes for our proposed method are available at https://github.com/wflrz123/MLAL .
更多查看译文
关键词
Meta-learning,Deep learning,Few-shot learning,Deep neural network,Attention
AI 理解论文
溯源树
样例
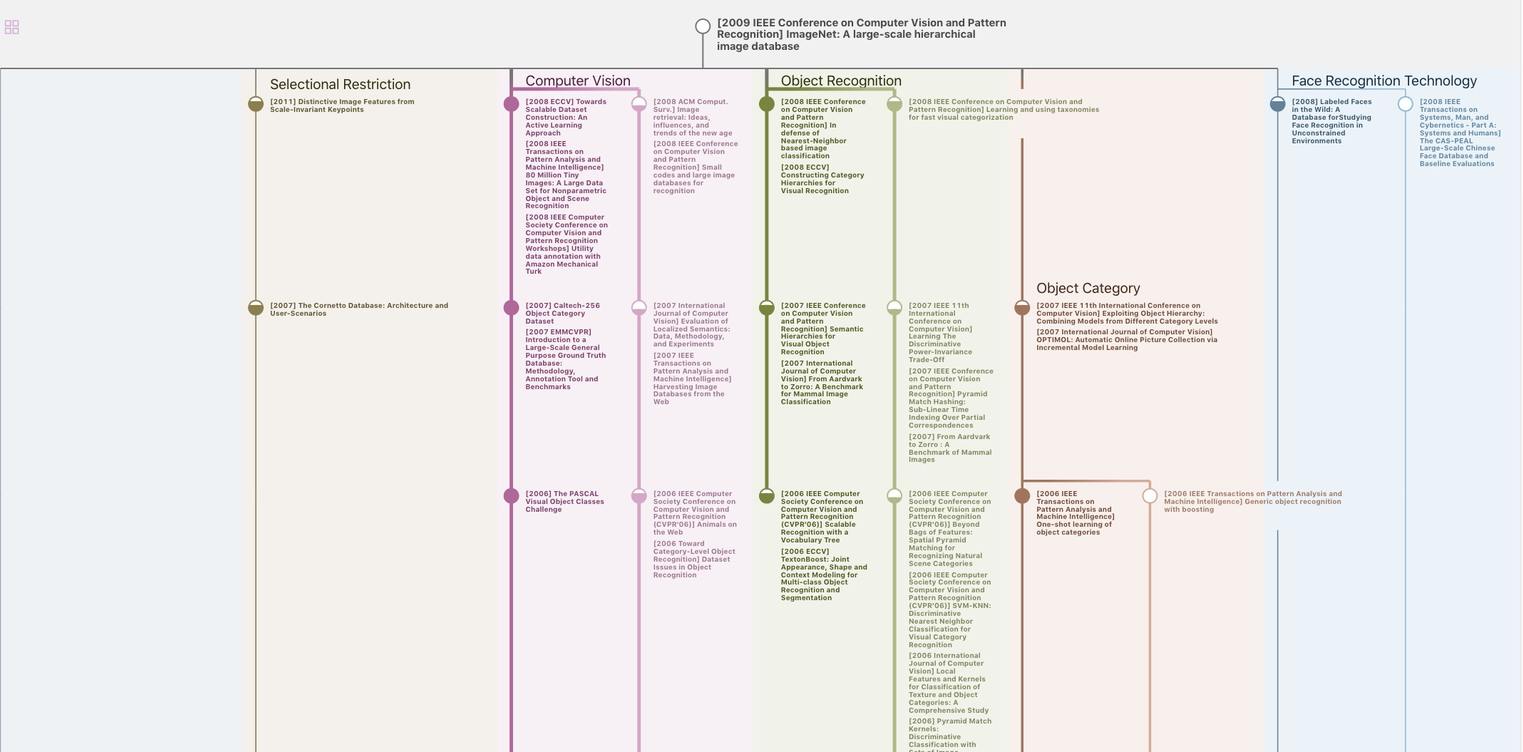
生成溯源树,研究论文发展脉络
Chat Paper
正在生成论文摘要