Semi-supervised class incremental broad network for continuous diagnosis of rotating machinery faults with limited labeled samples
KNOWLEDGE-BASED SYSTEMS(2024)
摘要
Machine learning has demonstrated remarkable success in the field of intelligent fault diagnosis. In current machine learning systems, models tend to be fixed after training, which makes them can only generalize to classes that appear in the training set, and cannot continuously learn newly emerging classes. However, in real industrial scenarios, industrial data is constantly growing, which requires models to be able to acquire new knowledge while retaining existing knowledge. Furthermore, a substantial proportion of the acquired samples in industry are unlabeled and only few samples are labeled. To tackle the above two challenges, this article proposes a novel semi-supervised class incremental broad network (SSCIBN) for incremental intelligent diagnosis of rotating machinery faults under limited labeled samples. Specifically, a semi-supervised graph embedding loss function is designed. On the one hand, this loss function can learn the structural information of a large amount of unlabeled data, which overcomes the limitation that the scarcity of labeled samples leads to poor model performance. On the other hand, local structure information within old and new classes is considered in the continuous learning process to fully learn the unique manifold structure information of different classes, which in turn enhances the discriminative performance between old and new classes. Furthermore, a novel semisupervised class incremental learning mechanism is proposed, which does not need to utilize the old class data during the incremental learning process, but can effectively retain the old class knowledge. The effectiveness of the proposed method is evaluated through multiple mechanical failure increment cases. Experimental analysis demonstrates that the designed SSCIBN has significant advantages in the class incremental application with the lack of labeled samples.
更多查看译文
关键词
Incremental learning,Broad learning system,Semi-supervised,Intelligent fault diagnosis
AI 理解论文
溯源树
样例
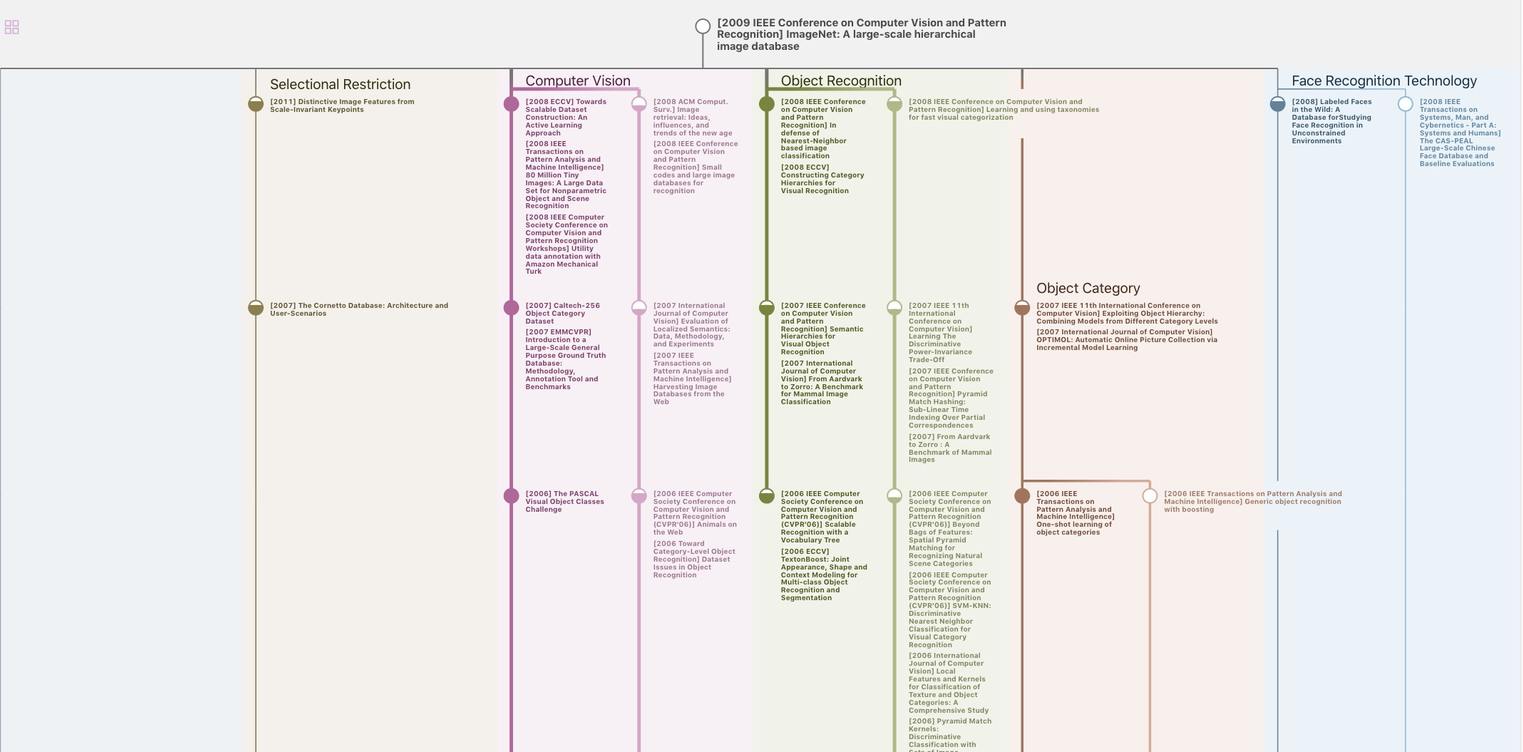
生成溯源树,研究论文发展脉络
Chat Paper
正在生成论文摘要