Enhancing Small Object Encoding in Deep Neural Networks: Introducing Fast Focused-Net with Volume-wise Dot Product Layer
CoRR(2024)
摘要
In this paper, we introduce Fast Focused-Net, a novel deep neural network
architecture tailored for efficiently encoding small objects into fixed-length
feature vectors. Contrary to conventional Convolutional Neural Networks (CNNs),
Fast Focused-Net employs a series of our newly proposed layer, the Volume-wise
Dot Product (VDP) layer, designed to address several inherent limitations of
CNNs. Specifically, CNNs often exhibit a smaller effective receptive field than
their theoretical counterparts, limiting their vision span. Additionally, the
initial layers in CNNs produce low-dimensional feature vectors, presenting a
bottleneck for subsequent learning. Lastly, the computational overhead of CNNs,
particularly in capturing diverse image regions by parameter sharing, is
significantly high. The VDP layer, at the heart of Fast Focused-Net, aims to
remedy these issues by efficiently covering the entire image patch information
with reduced computational demand. Experimental results demonstrate the prowess
of Fast Focused-Net in a variety of applications. For small object
classification tasks, our network outperformed state-of-the-art methods on
datasets such as CIFAR-10, CIFAR-100, STL-10, SVHN-Cropped, and Fashion-MNIST.
In the context of larger image classification, when combined with a transformer
encoder (ViT), Fast Focused-Net produced competitive results for OpenImages V6,
ImageNet-1K, and Places365 datasets. Moreover, the same combination showcased
unparalleled performance in text recognition tasks across SVT, IC15, SVTP, and
HOST datasets. This paper presents the architecture, the underlying motivation,
and extensive empirical evidence suggesting that Fast Focused-Net is a
promising direction for efficient and focused deep learning.
更多查看译文
AI 理解论文
溯源树
样例
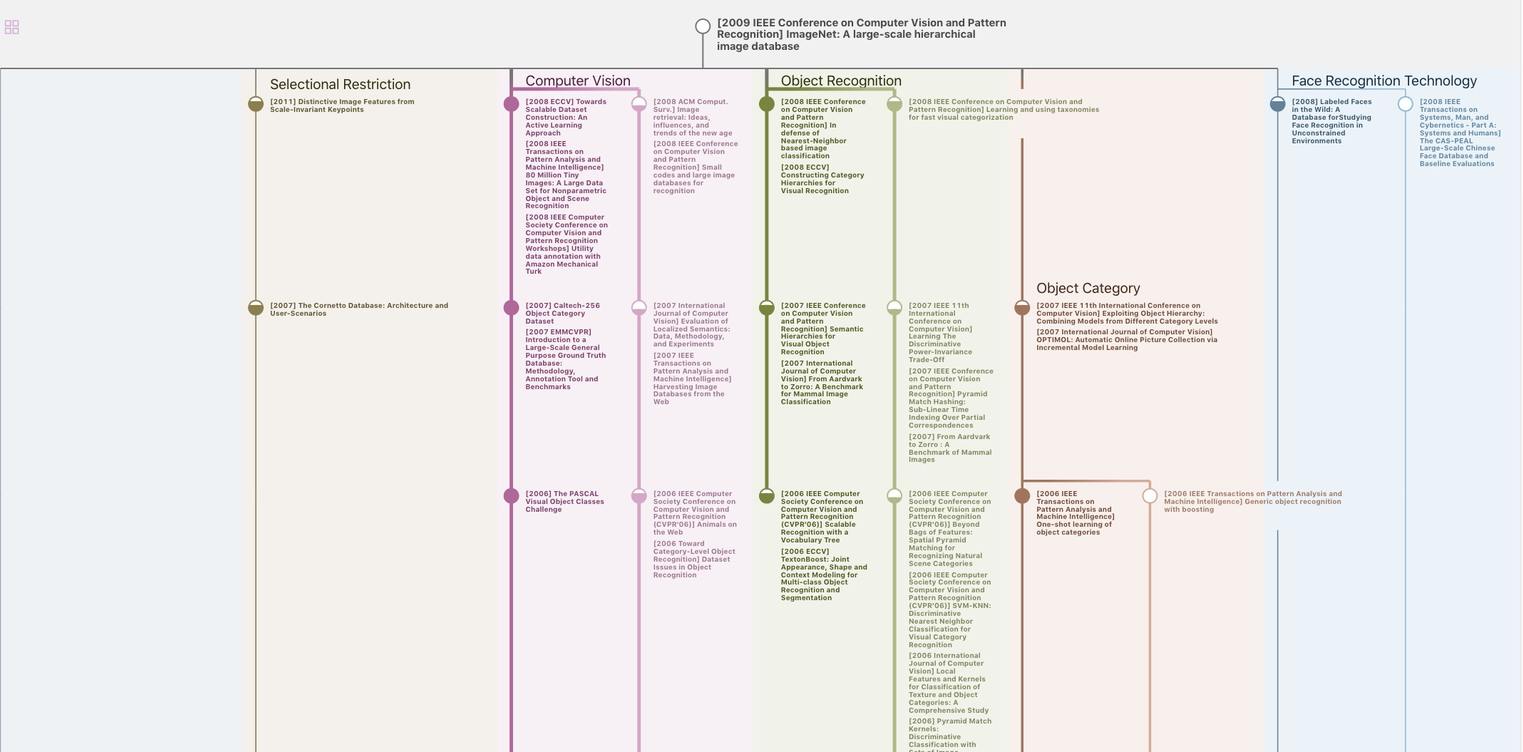
生成溯源树,研究论文发展脉络
Chat Paper
正在生成论文摘要