Spikeformer: Training high-performance spiking neural network with transformer
NEUROCOMPUTING(2024)
摘要
Although spiking neural networks (SNNs) have made great progress on both performance and efficiency over the last few years, their unique working pattern makes it hard to train high-performance low -latency SNNs and their development still lags behind traditional artificial neural networks (ANNs). To compensate this gap, many extraordinary works have been proposed, but these works are mainly based on the same network structure (i.e. CNN) and their performance is worse than their ANN counterparts, which limits the applications of SNNs. To this end, we propose a Transformer -based SNN, termed "Spikeformer", which outperforms its ANN counterpart on both static dataset and neuromorphic datasets. First, to deal with the problem of "data hungry"and the unstable training period exhibited in the vanilla model, we design the Convolutional Tokenizer (CT) module, which stabilizes training and improves the accuracy of the original model on DVS -Gesture by more than 16%. Besides, we integrate Spatio-Temporal Attention (STA) into Spikeformer to better incorporate the attention mechanism inside Transformer and the spatio-temporal information inherent to SNN. With our proposed method, we achieve 98.96%/75.89% top -1 accuracy on DVS-Gesture/ImageNet datasets with 16/4 simulation time steps. On DVS-CIFAR10, we further conduct energy consumption analysis and obtain 81.4%/80.3% top -1 accuracy with 4/1 time step(s), achieving 1.7/6.4x energy efficiency over its ANN counterpart. Moreover, our Spikeformer outperforms its ANN counterpart by 3.13% and 0.12% on DVS -Gesture and ImageNet respectively, indicating that Spikeformer may be a more suitable architecture for training SNNs compared to CNN. We believe that this work shall promote the development of SNNs to be in step with ANNs as much as possible. Code will be publicly available.
更多查看译文
关键词
Spiking neural networks,Transformer,Spatio-temporal attention,Deep neural architectures,Artificial intelligence
AI 理解论文
溯源树
样例
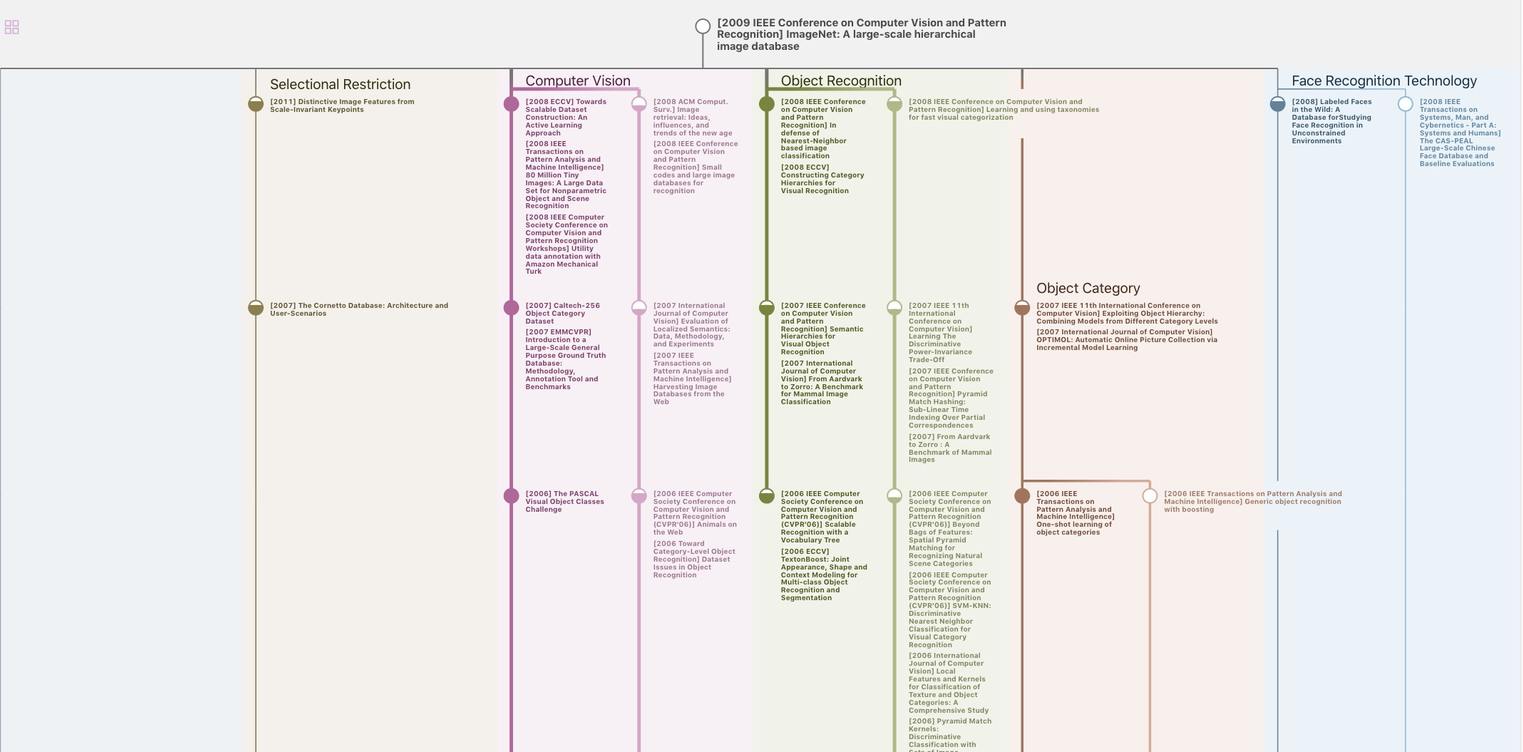
生成溯源树,研究论文发展脉络
Chat Paper
正在生成论文摘要