Joint Distribution Adaptive-Alignment for Cross-Domain Segmentation of High-Resolution Remote Sensing Images
IEEE TRANSACTIONS ON GEOSCIENCE AND REMOTE SENSING(2024)
摘要
Although existing unsupervised domain adaptation (UDA) methods have successfully applied to semantic segmentation tasks for high-resolution remote sensing (HRS) images, they still have some limitations that need to be addressed: 1) they mainly focus on aligning the marginal distributions while ignoring the interdomain differences in the conditional distributions, which may be suboptimal because they assume that the boundaries of category decision are identical across domains; and 2) they depend on self-supervised learning for easy-to-hard alignment, which may result in model learning erroneous knowledge from the pseudo labels. To address the above limitations, we propose a joint distribution adaptive-alignment framework (JDAF) to eliminate the distribution difference between the source and target domains, which is mainly composed of a marginal distribution alignment (MDA) module, a conditional distribution alignment (CDA) module, and an improved easy-to-hard adaptation strategy. The MDA module is used to narrow local semantic and global spatial differences between domains and first advance, and then, the CDA module that includes a category-invariant feature alignment (CFA) block and a dataset-level context aggregation (DCA) block is presented and designed, which can dynamically update and align the feature representations that are invariant to category change and adaptively incorporate dataset-level context into the features of source domain to enhance the pixel-level representation. An uncertainty-adaptive learning (UAL) method is, moreover, proposed to improve the easy-to-hard adaptation strategy by enabling the model to learn accurate knowledge from the pseudo labels, which can boost the adaptive performance of the whole JDAF. Comprehensive experiments with four cross-domain tasks on two benchmark datasets of aerospace HRS images demonstrate that the proposed JDAF achieves significant performance gains compared to the state-of-the-art cross-domain semantic segmentation methods. Our code is available at: https://github.com/maple-hx/JDAF.
更多查看译文
关键词
Dataset-level context aggregation (DCA),high-resolution remote sensing (HRS) images,joint distribution alignment,semantic segmentation,uncertainty-adaptive learning (UAL),unsupervised domain adaptation (UDA)
AI 理解论文
溯源树
样例
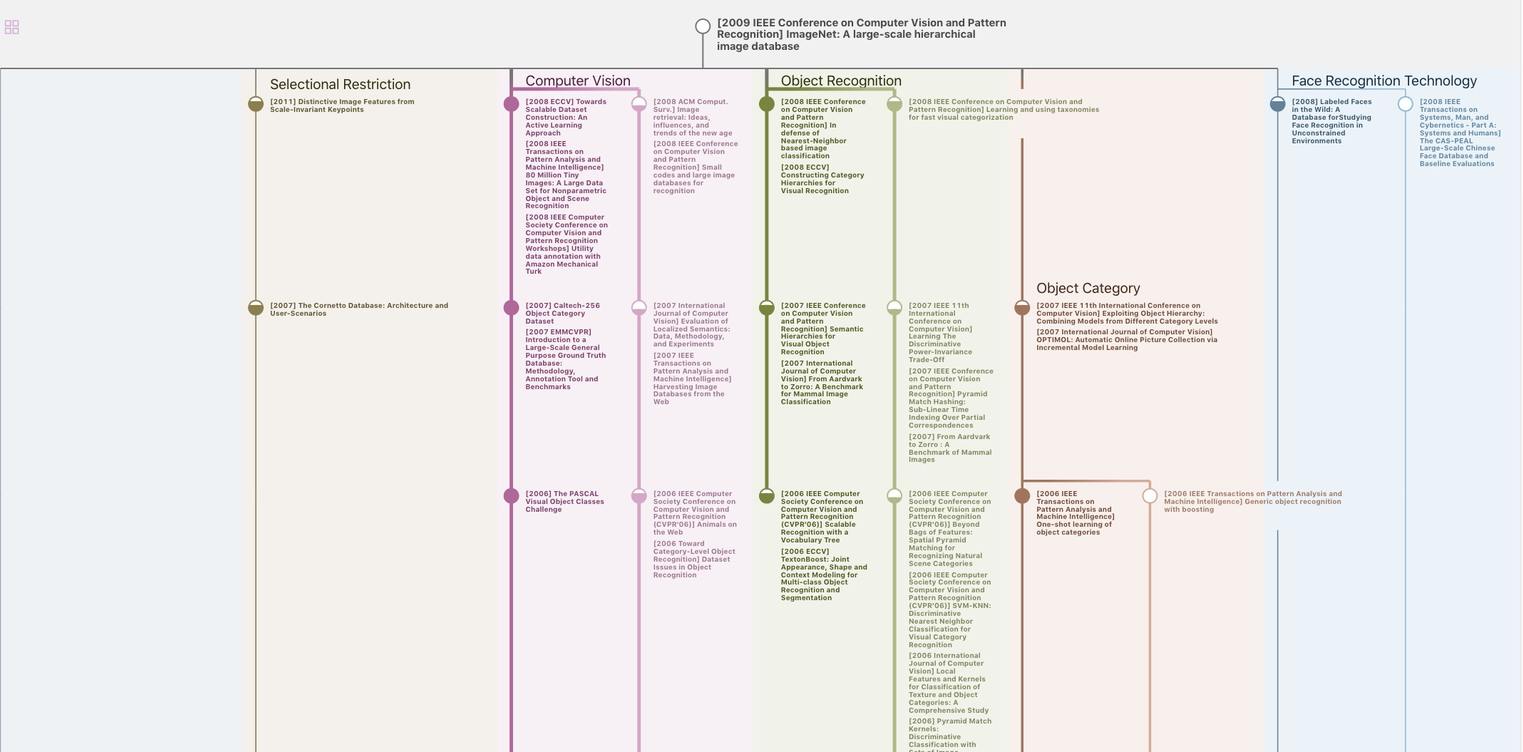
生成溯源树,研究论文发展脉络
Chat Paper
正在生成论文摘要