The Effect of Intrinsic Dataset Properties on Generalization: Unraveling Learning Differences Between Natural and Medical Images
CoRR(2024)
摘要
This paper investigates discrepancies in how neural networks learn from
different imaging domains, which are commonly overlooked when adopting computer
vision techniques from the domain of natural images to other specialized
domains such as medical images. Recent works have found that the generalization
error of a trained network typically increases with the intrinsic dimension
(d_data) of its training set. Yet, the steepness of this relationship
varies significantly between medical (radiological) and natural imaging
domains, with no existing theoretical explanation. We address this gap in
knowledge by establishing and empirically validating a generalization scaling
law with respect to d_data, and propose that the substantial scaling
discrepancy between the two considered domains may be at least partially
attributed to the higher intrinsic "label sharpness" (K_F) of medical imaging
datasets, a metric which we propose. Next, we demonstrate an additional benefit
of measuring the label sharpness of a training set: it is negatively correlated
with the trained model's adversarial robustness, which notably leads to models
for medical images having a substantially higher vulnerability to adversarial
attack. Finally, we extend our d_data formalism to the related metric of
learned representation intrinsic dimension (d_repr), derive a
generalization scaling law with respect to d_repr, and show that d_data
serves as an upper bound for d_repr. Our theoretical results are supported
by thorough experiments with six models and eleven natural and medical imaging
datasets over a range of training set sizes. Our findings offer insights into
the influence of intrinsic dataset properties on generalization, representation
learning, and robustness in deep neural networks.
更多查看译文
关键词
generalization,medical images,scaling law,intrinsic dimension,medical image analysis
AI 理解论文
溯源树
样例
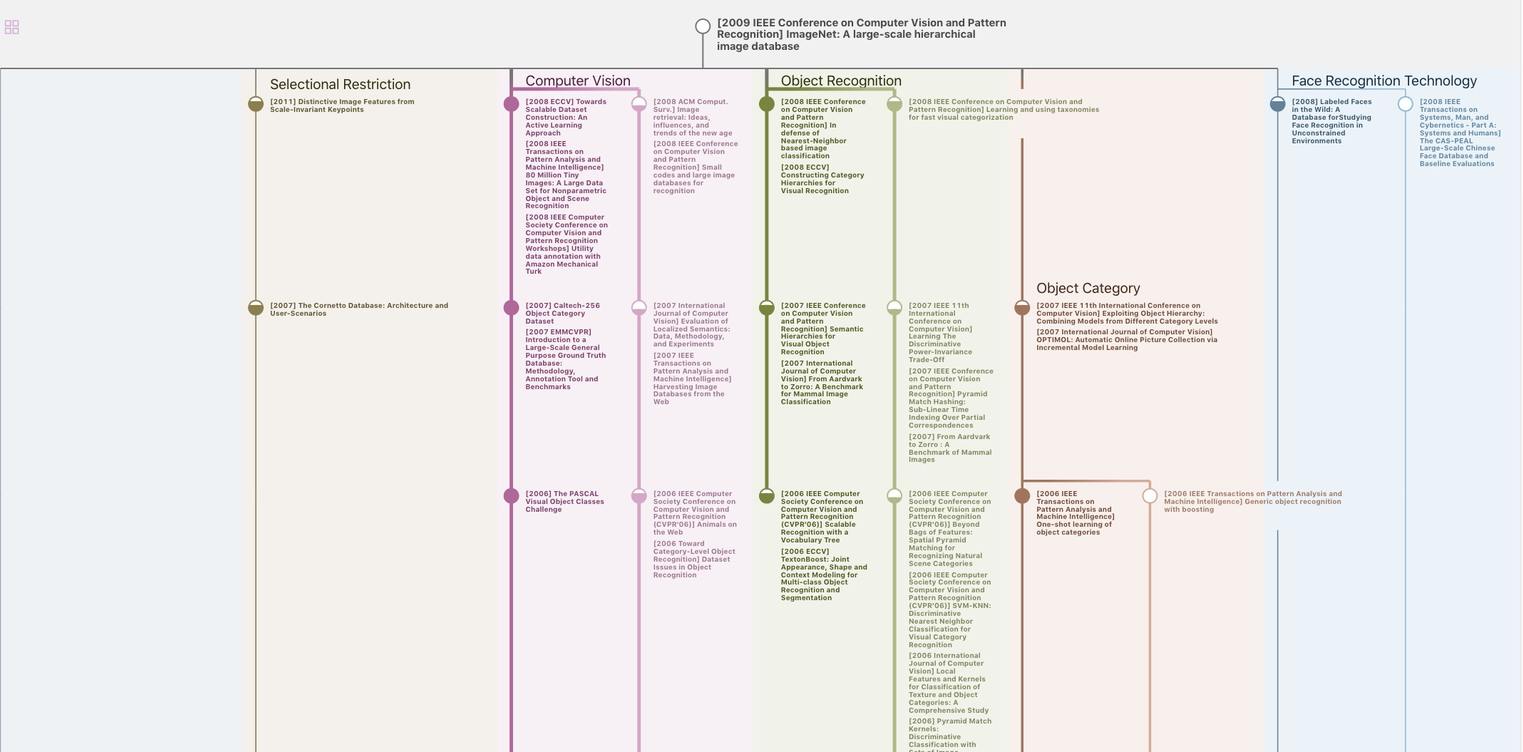
生成溯源树,研究论文发展脉络
Chat Paper
正在生成论文摘要