HierSFL: Local Differential Privacy-aided Split Federated Learning in Mobile Edge Computing
CoRR(2024)
摘要
Federated Learning is a promising approach for learning from user data while
preserving data privacy. However, the high requirements of the model training
process make it difficult for clients with limited memory or bandwidth to
participate. To tackle this problem, Split Federated Learning is utilized,
where clients upload their intermediate model training outcomes to a cloud
server for collaborative server-client model training. This methodology
facilitates resource-constrained clients' participation in model training but
also increases the training time and communication overhead. To overcome these
limitations, we propose a novel algorithm, called Hierarchical Split Federated
Learning (HierSFL), that amalgamates models at the edge and cloud phases,
presenting qualitative directives for determining the best aggregation
timeframes to reduce computation and communication expenses. By implementing
local differential privacy at the client and edge server levels, we enhance
privacy during local model parameter updates. Our experiments using CIFAR-10
and MNIST datasets show that HierSFL outperforms standard FL approaches with
better training accuracy, training time, and communication-computing
trade-offs. HierSFL offers a promising solution to mobile edge computing's
challenges, ultimately leading to faster content delivery and improved mobile
service quality.
更多查看译文
关键词
Federated Learning,Mobile Edge Computing,Training Time,Cloud Computing,Data Privacy,MNIST Dataset,Edge Server,Local Updates,Differential Privacy,Collaborative Training,Loss Function,Learning Models,Deep Neural Network,Gradient Descent,Source Code,Network Topology,Stochastic Gradient Descent,Weight Vector,Unmanned Aerial Vehicles,Learning Problem,Central Server,Weight Sensitivity,Model Aggregation,Laplace Distribution,Communication Delay,Parameter Server
AI 理解论文
溯源树
样例
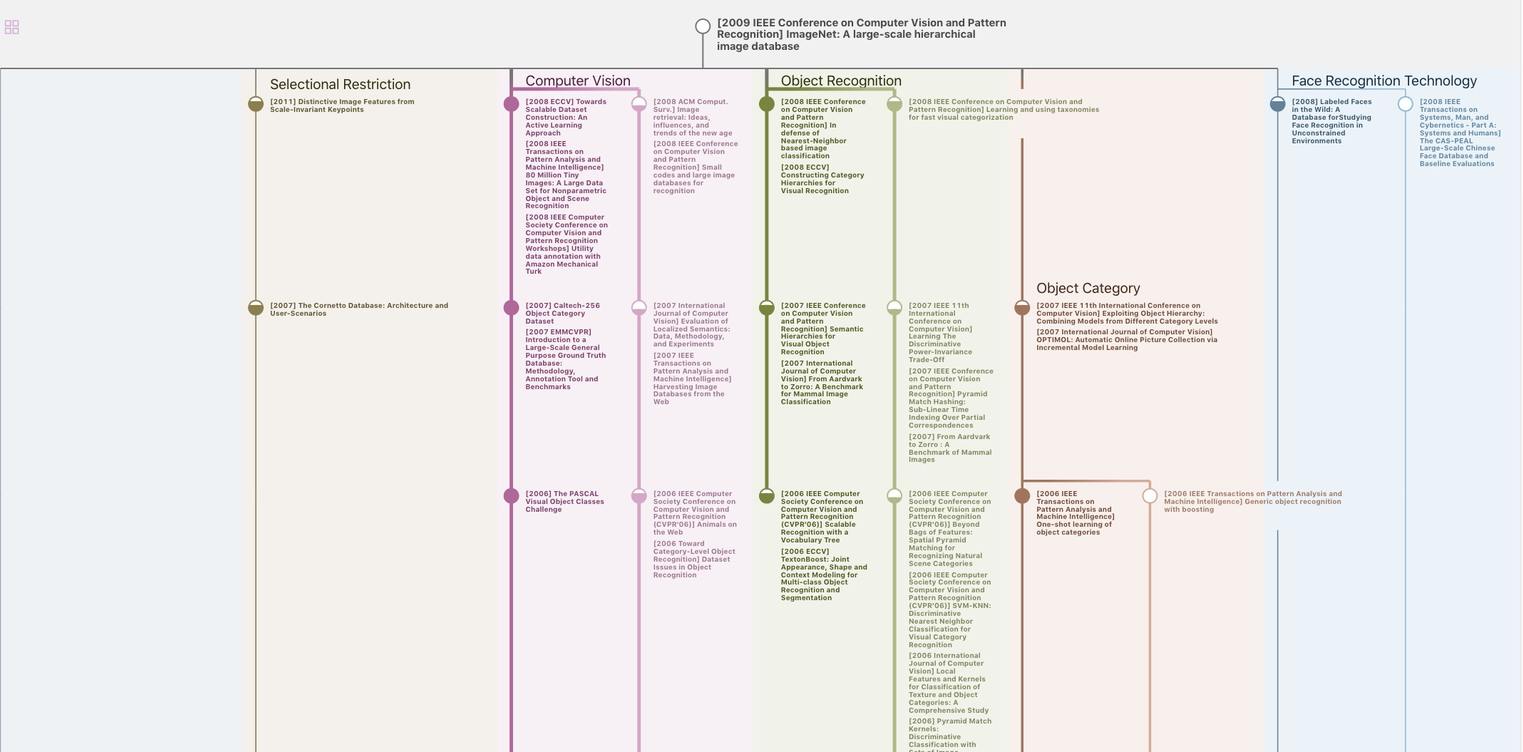
生成溯源树,研究论文发展脉络
Chat Paper
正在生成论文摘要