GNN-IR: Examining graph neural networks for influencer recommendations in social media marketing
JOURNAL OF RETAILING AND CONSUMER SERVICES(2024)
摘要
With the notable growth of the Internet, a number of platforms have emerged and attracted an enormous number of users. Based on the impact of these platforms, some 'influencers' are highlighted. These influencers wield significant power, shaping consumer behavior. This influence spawned the concept of influencer marketing, where companies leverage these personalities to advertise their products. YouTube stands out as a prominent platform in this trend. However, considering the limited number of influencers and their concepts, the majority of companies, which hope to conduct their marketing campaigns with influencers face challenges in identifying suitable influencers for their campaigns. With this trend, we introduce GNN-IR, a graph neural network for influencer recommendation, based on the connections between companies and influencers of YouTube, one of the largest content platforms. In developing GNN-IR, we adopted a data -driven methodology utilizing a meticulously curated dataset collected in-house. Our dataset comprises a total of 25,174 relationship entries between advertisers and influencers, involving 1,886 distinct advertisers and 3,812 unique YouTube influencers. It encompasses diverse data modalities, including images, text, and assorted metadata. The data was sourced from two primary platforms: YouTube and ugwanggi. Ugwanggi provided valuable insights into the relationships between advertisers and influencers via their information. Meanwhile, YouTube offered more comprehensive and detailed influencer -centric information. We employed PyTorch Geometric to construct a bipartite graph representing interconnected data. Our recommendation system operates via link prediction, suggesting the Topk influencers to advertisers based on the calculated connection probability between nodes. To assess GNN-IR's performance, we employed a range of evaluation metrics. For link prediction, we measured Accuracy, Precision, Recall, and F1 -score. Additionally, in the recommendation phase, we evaluated Precision@k, Recall@k, and F1-score@k. Using GNN-IR and incorporating profile images from YouTube, keyword features, metadata, and sentiment gleaned from YouTube comments, we achieved Precision levels of 96.51% at k=1 and 93.68% at k=10. Based on the experimental results, several implications and limitations are presented.
更多查看译文
关键词
Influencer marketing,Recommendation,Link prediction,Bipartite graph,YouTube commercial
AI 理解论文
溯源树
样例
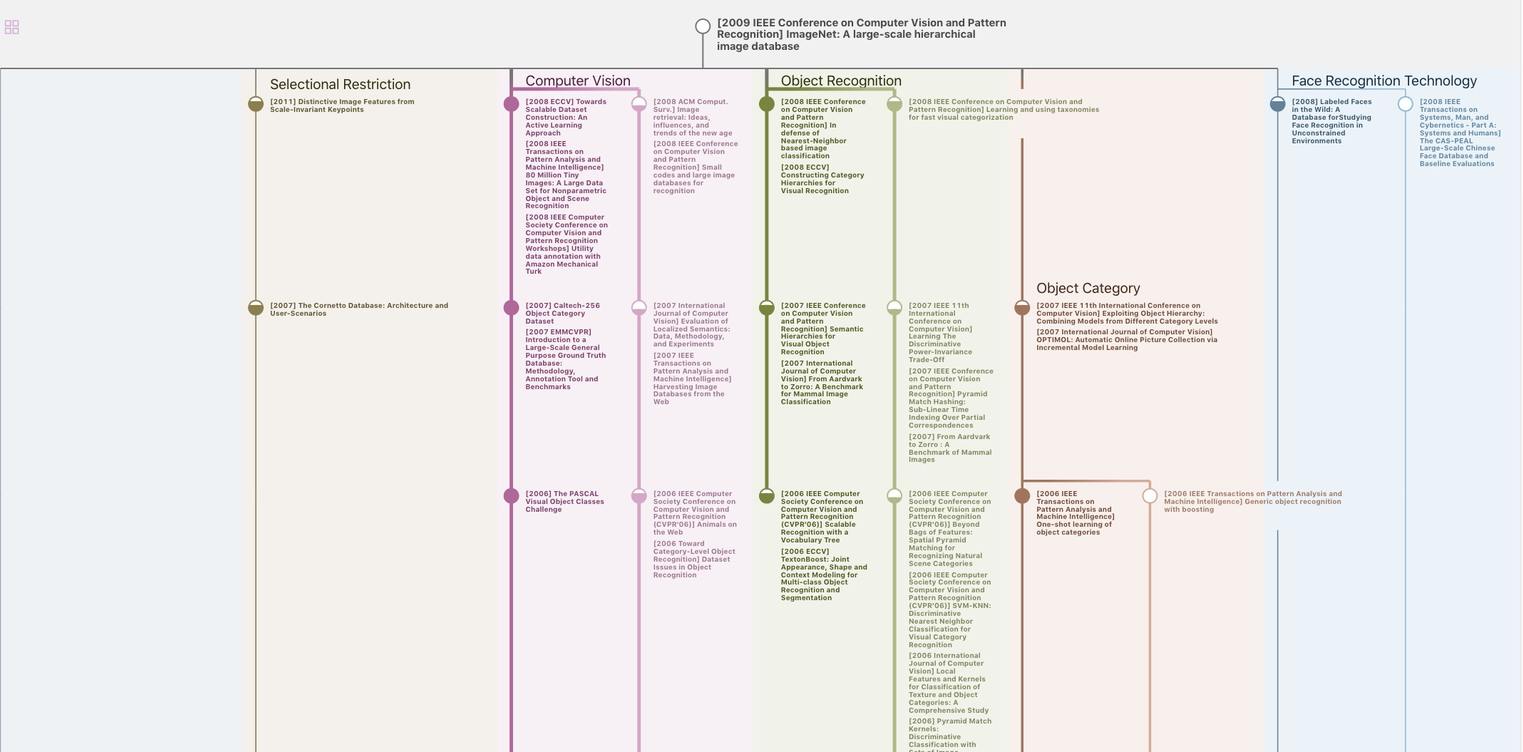
生成溯源树,研究论文发展脉络
Chat Paper
正在生成论文摘要