A Survey of Resource-efficient LLM and Multimodal Foundation Models
CoRR(2024)
摘要
Large foundation models, including large language models (LLMs), vision
transformers (ViTs), diffusion, and LLM-based multimodal models, are
revolutionizing the entire machine learning lifecycle, from training to
deployment. However, the substantial advancements in versatility and
performance these models offer come at a significant cost in terms of hardware
resources. To support the growth of these large models in a scalable and
environmentally sustainable way, there has been a considerable focus on
developing resource-efficient strategies. This survey delves into the critical
importance of such research, examining both algorithmic and systemic aspects.
It offers a comprehensive analysis and valuable insights gleaned from existing
literature, encompassing a broad array of topics from cutting-edge model
architectures and training/serving algorithms to practical system designs and
implementations. The goal of this survey is to provide an overarching
understanding of how current approaches are tackling the resource challenges
posed by large foundation models and to potentially inspire future
breakthroughs in this field.
更多查看译文
AI 理解论文
溯源树
样例
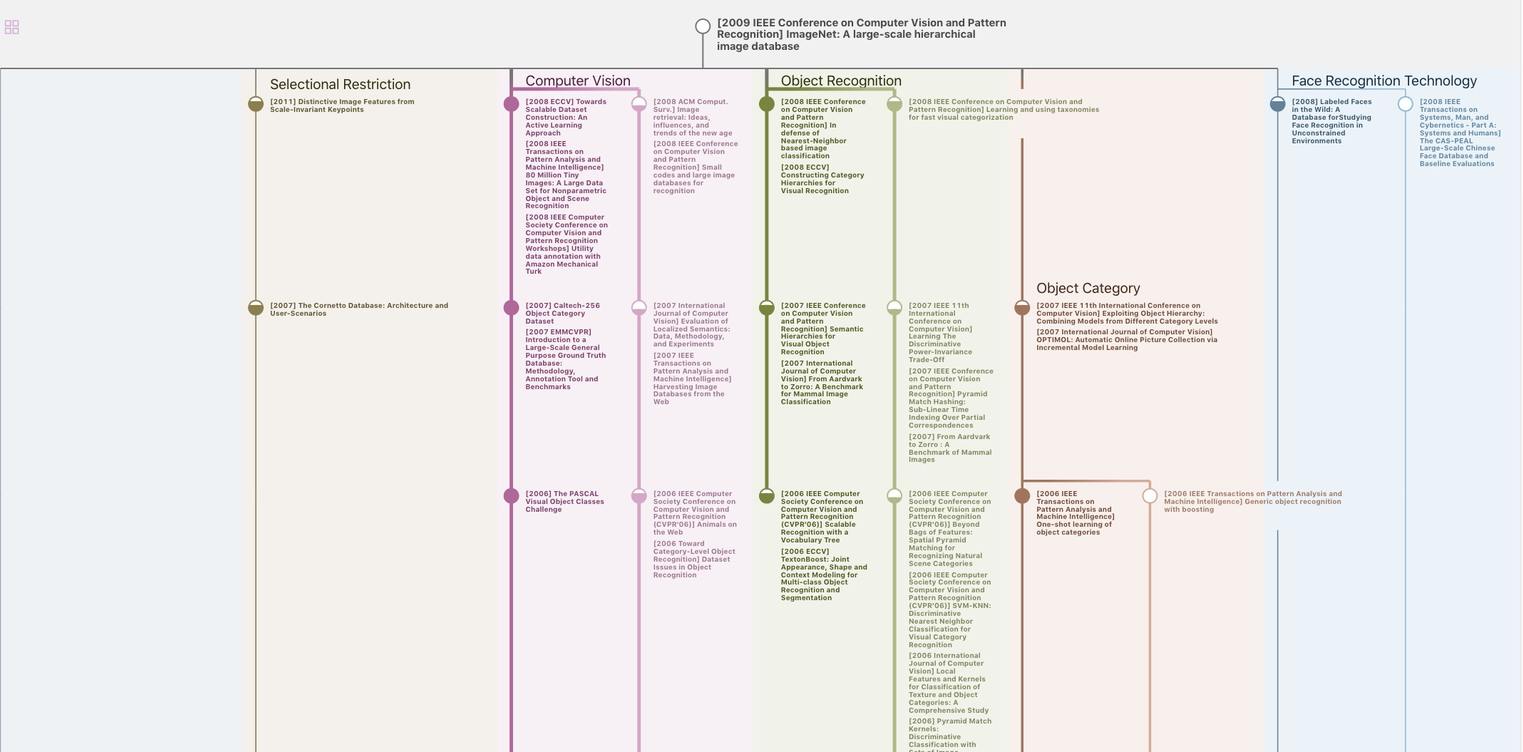
生成溯源树,研究论文发展脉络
Chat Paper
正在生成论文摘要