Audio Mixing Inversion via Embodied Self-supervised Learning
Machine Intelligence Research(2024)
摘要
Audio mixing is a crucial part of music production. For analyzing or recreating audio mixing, it is of great importance to conduct research on estimating mixing parameters used to create mixdowns from music recordings, i.e., audio mixing inversion. However, approaches of audio mixing inversion are rarely explored. A method of estimating mixing parameters from raw tracks and a stereo mixdown via embodied self-supervised learning is presented. In this work, several commonly used audio effects including gain, pan, equalization, reverb, and compression, are taken into consideration. This method is able to learn an inference neural network that takes a stereo mixdown and the raw audio sources as input and estimate mixing parameters used to create the mixdown by iteratively sampling and training. During the sampling step, the inference network predicts a set of mixing parameters, which is sampled and fed to an audio-processing framework to generate audio data for the training step. During the training step, the same network used in the sampling step is optimized with the sampled data generated from the sampling step. This method is able to explicitly model the mixing process in an interpretable way instead of using a black-box neural network model. A set of objective measures are used for evaluation. The experimental results show that this method has better performance than current state-of-the-art methods.
更多查看译文
关键词
Audio mixing inversion,intelligent audio mixing,self-supervised learning,audio signal processing,deep learning
AI 理解论文
溯源树
样例
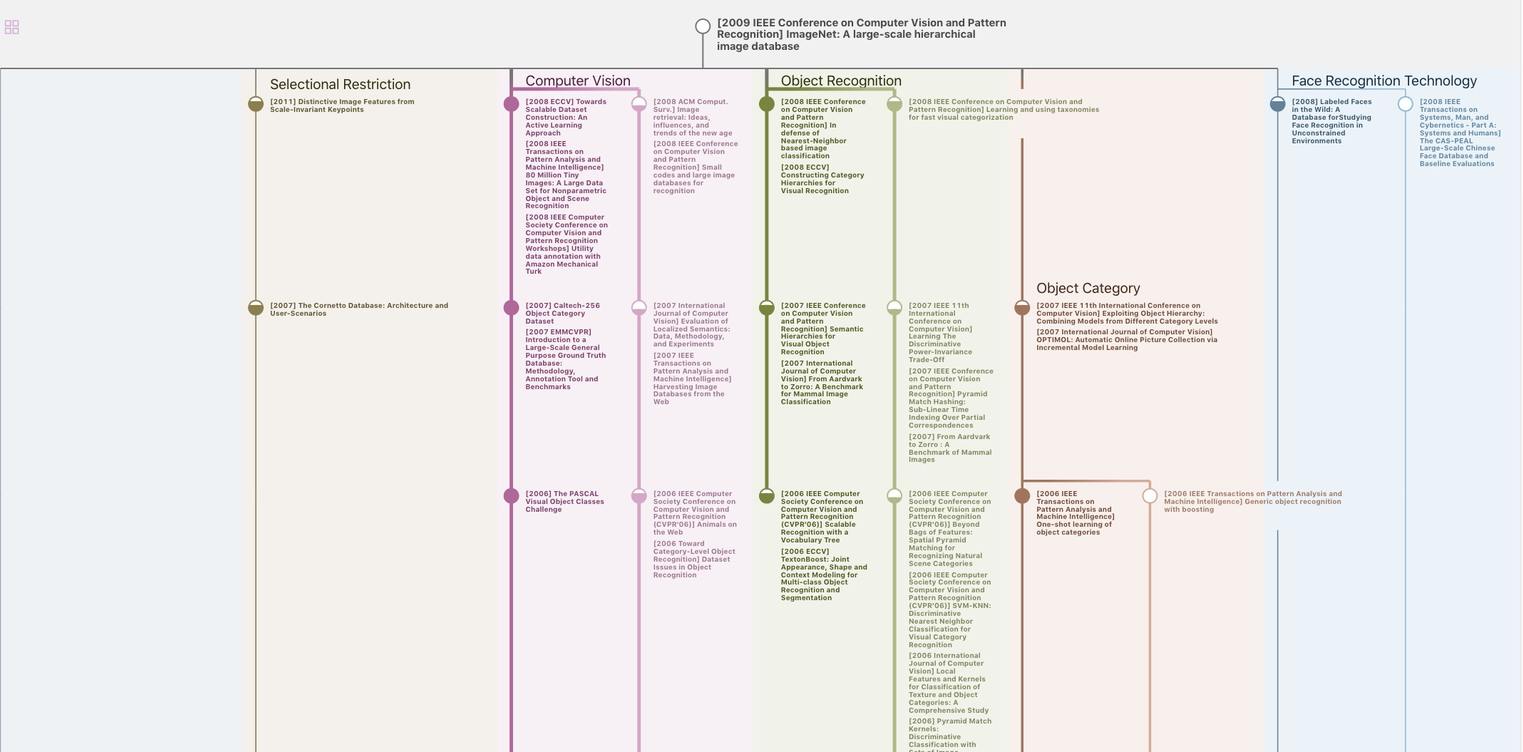
生成溯源树,研究论文发展脉络
Chat Paper
正在生成论文摘要