Abnormal Temperature Detection of Blower Components Based on Infrared Video Images Analysis
IEEE Sensors Journal(2024)
摘要
Industry prioritizes using infrared (IR) sensors to obtain temperature information and detect equipment failures early. Existing methods have accuracy, segmentation, and real-time detection issues. This article uses infrared video data to detect temperature faults in blowers. To improve equipment part segmentation accuracy, a robust segmentation algorithm is adopted based on improved point rendering infrared blower images. A hierarchical multiscene anomaly detection method for analyzing temperature profiles in the blower region is proposed.
${K}$
-means++ clustering is used for coarse classification, followed by k-nearest neighbor (KNN) analysis to identify anomalous data. Anomalous clustering center data is then scored using sliding windows. These scores are then used as the new anomaly indicators, and the time interval of the anomaly is estimated. The main contribution of this work is to propose a method to improve the accuracy and robustness of temperature anomaly detection in industrial objects that are in complex environments and background scenarios.
更多查看译文
关键词
Anomaly detection,infrared (IR) image and video,semantic segmentation,small targets,time series clustering and classification
AI 理解论文
溯源树
样例
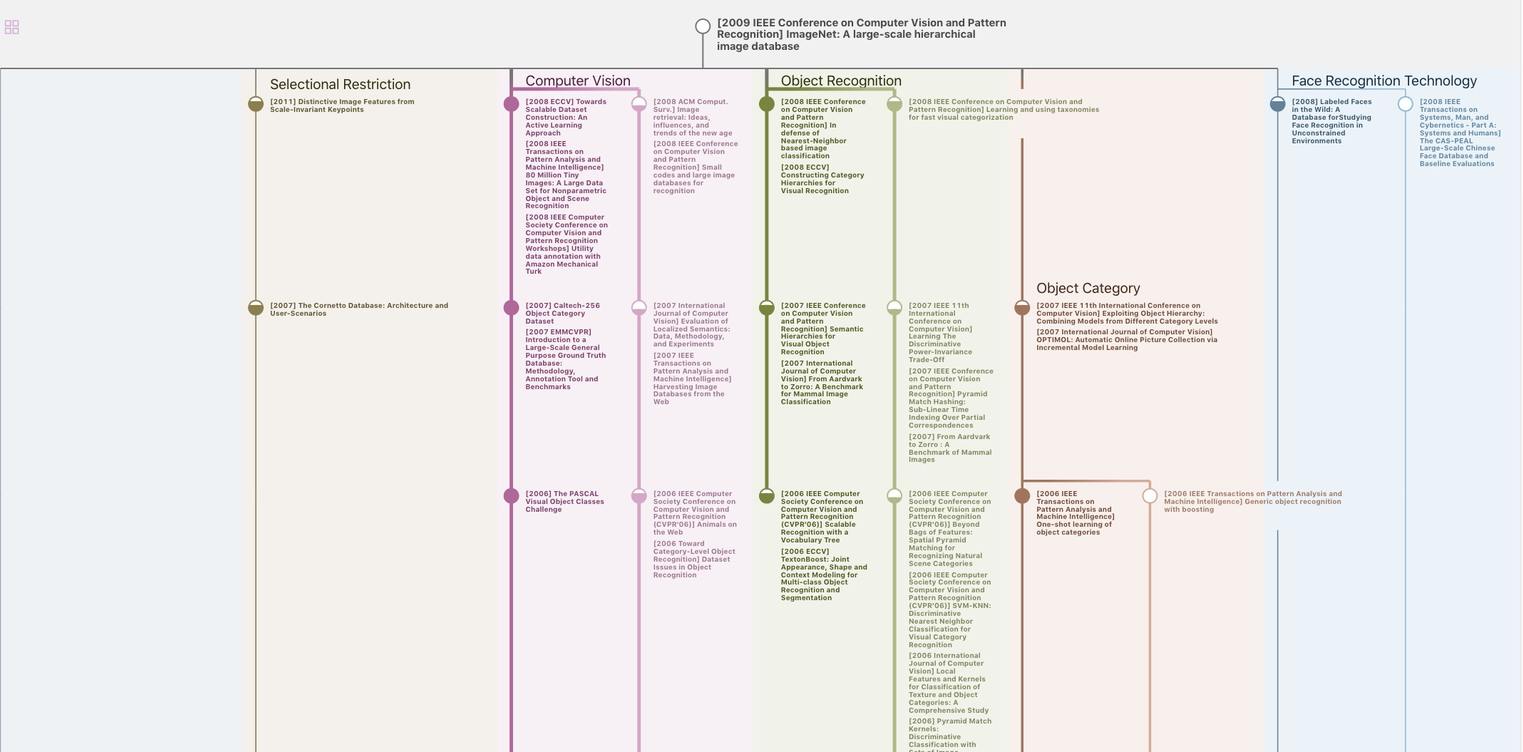
生成溯源树,研究论文发展脉络
Chat Paper
正在生成论文摘要