Discriminative histone imputation using chromatin accessibility
biorxiv(2024)
摘要
Motivation: Histone modifications (HMs) play a crucial role in various biological processes, including transcription, replication, and DNA repair, and they have a significant impact on chromatin structure. However, annotating HMs across different cell types using experimental approaches alone is impractical due to cost and time constraints. Therefore, computational techniques are valuable for predicting HMs, complementing experimental methods, and enhancing our understanding of DNA characteristics and gene expression patterns. Results: In this study, we introduce a deep learning model called discriminative histone imputation using chromatin accessibility (dHICA). dHICA combines extensive DNA sequence information with chromatin accessibility data, utilizing the Transformer architecture. This allows dHICA to have a large receptive field, and more cell-type specific information, which can not only incorporate distal information across the entire genome, but also improve predictive accuracy and interpretability. Comparing performance with other models, we found that dHICA exhibits superior performance in both peak calling and signal predictions, especially in biologically salient regions such as gene elements. dHICA serves as a crucial tool for advancing our understanding of chromatin dynamics, offering enhanced predictive capabilities and interpretability, particularly in critical biological regions such as gene elements.
### Competing Interest Statement
The authors have declared no competing interest.
更多查看译文
AI 理解论文
溯源树
样例
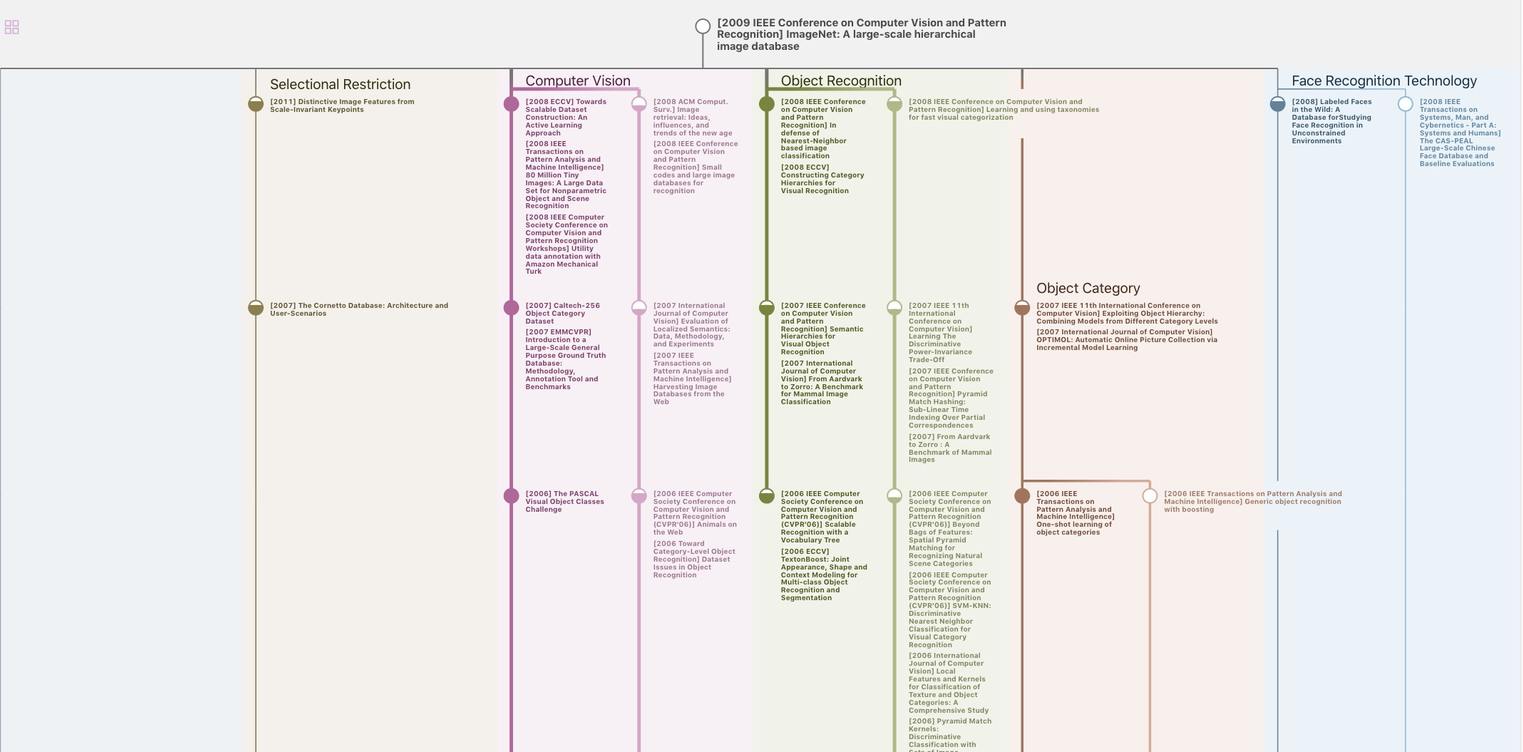
生成溯源树,研究论文发展脉络
Chat Paper
正在生成论文摘要