Stock price prediction: comparison of different moving average techniques using deep learning model
Neural Computing and Applications(2024)
摘要
The stock market is changing quickly, and its nonlinear characteristics make stock price prediction difficult. Predicting stock prices is challenging due to several factors, including a company’s financial performance, unforeseen circumstances, general economic conditions, politics, current assets, global situation, etc. Despite these terms, sufficient data are available to identify stock price movement trends using the different technical approaches. In this research, we empirically analyzed long short-term memory (LSTM) networks in the context of time-series prediction. Our investigation leveraged a diverse set of real-world datasets and provided quantitative insights into the performance of LSTMs. Across a spectrum of time-series forecasting tasks, LSTM models demonstrated an impressive mean absolute error (MAE) reduction of 23.4% compared to traditional forecasting methods. Specifically, LSTM achieved an average prediction accuracy of 89.7% in financial market predictions, outperforming baseline models by a significant margin. The aim is to obtain a value that can be compared to the present price of an asset to determine whether it is overvalued or undervalued, which anticipates the price patterns by analyzing previous market information, such as price and volume, compared to this stock analysis approach.
更多查看译文
关键词
Stock price,Simple moving average,Exponential moving average,Deep learning,Machine learning,Prediction,Moving average,Stock market price movement
AI 理解论文
溯源树
样例
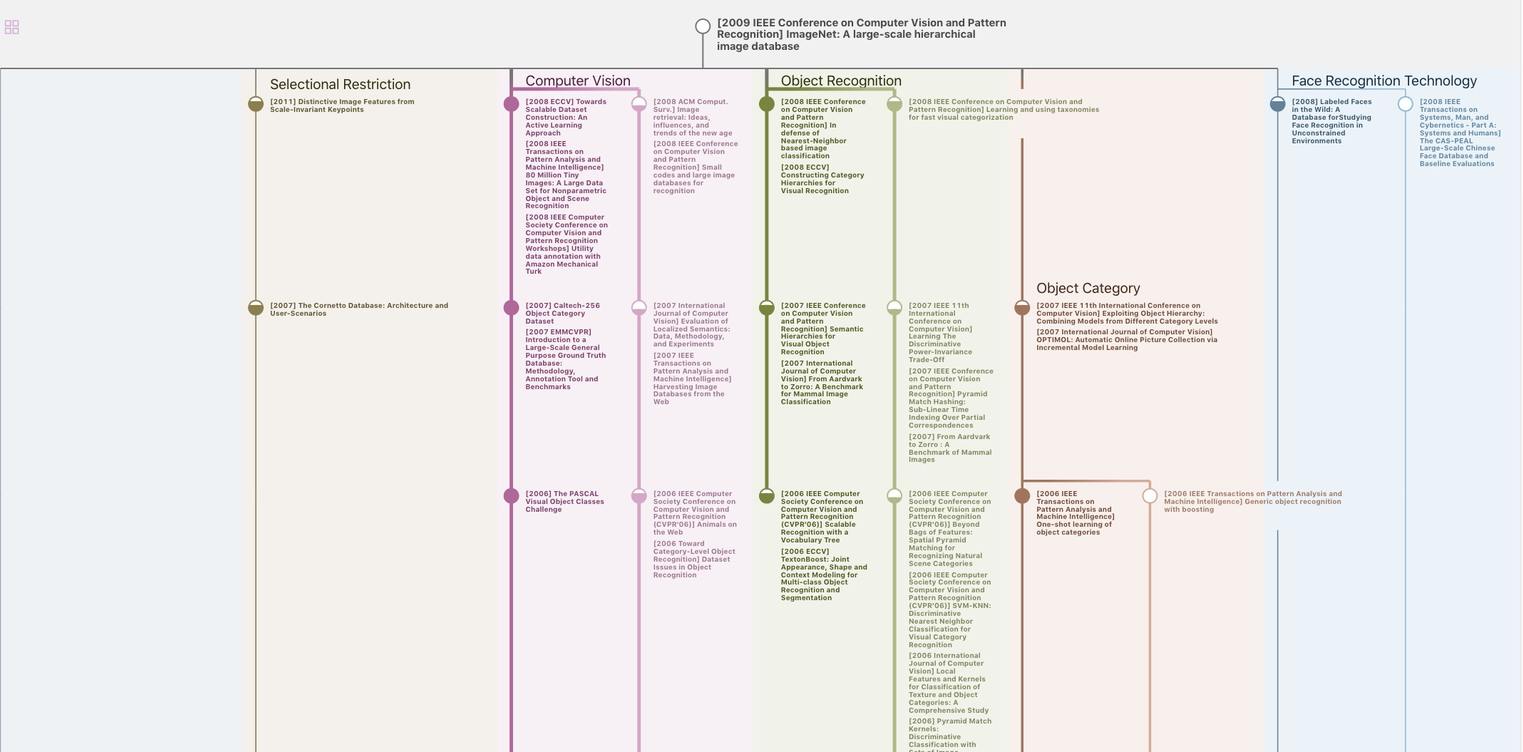
生成溯源树,研究论文发展脉络
Chat Paper
正在生成论文摘要