Multi-label feature selection with global and local label correlation
EXPERT SYSTEMS WITH APPLICATIONS(2024)
摘要
In various application domains, high -dimensional multi -label data has become more prevalent, presenting two significant challenges: instances with high -dimensional features and a large number of labels. In the context of multi -label feature selection, the objective is to choose a subset of features from a given set that is highly pertinent for predicting multiple labels or categories associated with each instance. However, certain characteristics of multi -label classification, such as label dependencies and imbalanced label distribution, have often been overlooked although they hold valuable insights for designing effective multi -label feature selection algorithms. In this paper, we propose a feature selection model which exploits explicit global and local label correlations to select discriminative features across multiple labels. In addition, by representing the feature matrix and label matrix in a shared latent space, the model aims to capture the underlying correlations between features and labels. The shared representation can reveal common patterns or relationships that exist across multiple labels and features. An objective function involving L21 -norm regularization is formulated, and an alternating optimization -based iterative algorithm is designed to obtain the sparse coefficients for multilabel feature selection. The proposed method was evaluated on 14 real -world multi -label datasets using six evaluation metrics, through comprehensive experiments. The results indicate its effectiveness, surpassing that of several representative methods.
更多查看译文
关键词
Feature selection,Multi-label learning,Label correlation,Nonnegative matrix factorization
AI 理解论文
溯源树
样例
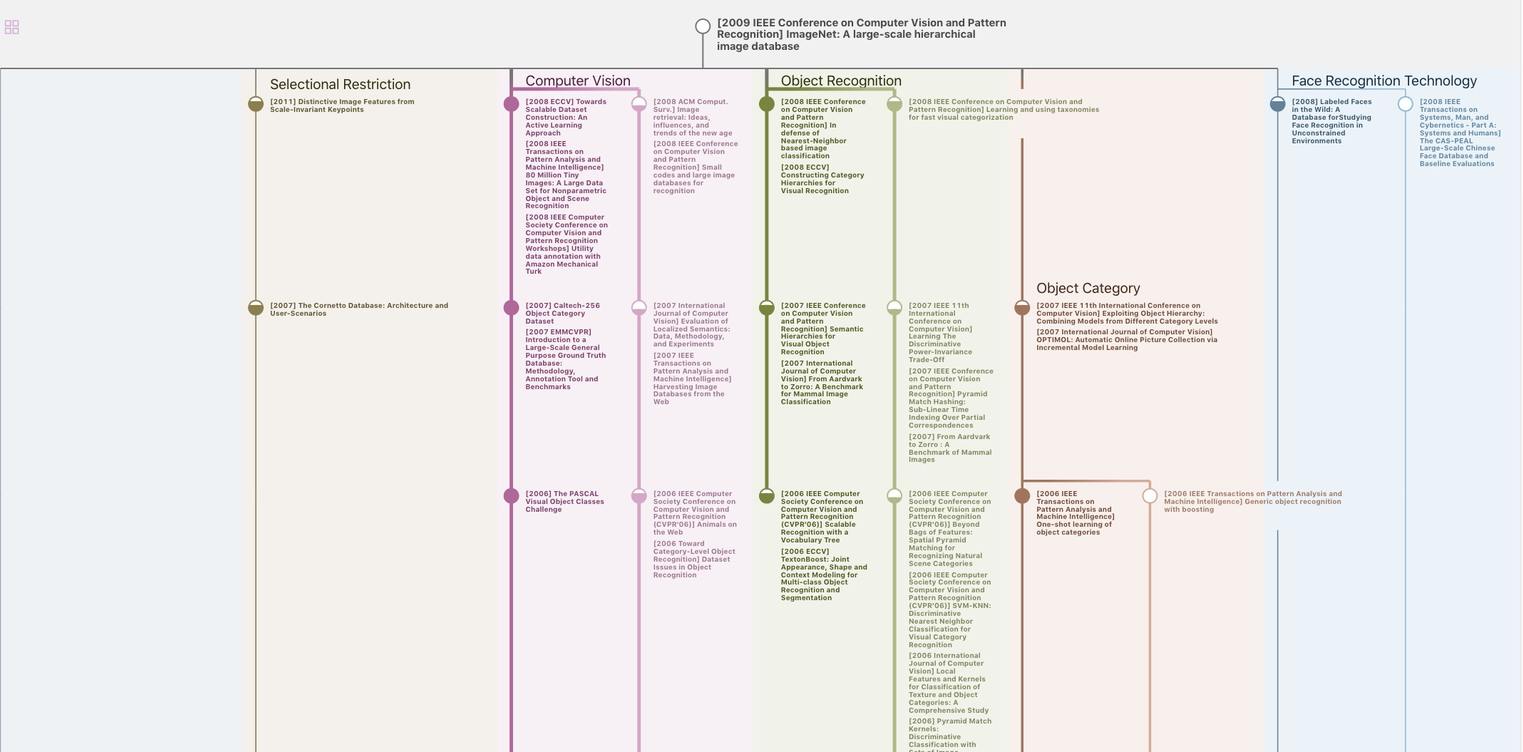
生成溯源树,研究论文发展脉络
Chat Paper
正在生成论文摘要