Contrastive and adversarial regularized multi-level representation learning for incomplete multi-view clustering
NEURAL NETWORKS(2024)
摘要
Incomplete multi-view clustering is a significant task in machine learning, given that complex systems in nature and society cannot be fully observed; it provides an opportunity to exploit the structure and functions of underlying systems. Current algorithms are criticized for failing either to balance data restoration and clustering or to capture the consistency of the representation of various views. To address these problems, a novel Multi-level Representation Learning Contrastive and Adversarial Learning (aka MRL_CAL) for incomplete multi-view clustering is proposed, in which data restoration, consistent representation, and clustering are jointly learned by exploiting features in various subspaces. Specifically, MRL_CAL employs nu auto-encoder to obtain a low-level specific-view representation of instances, which restores data by estimating the distribution of the original incomplete data with adversarial learning. Then, MRL_CAL extracts a high-level representation of instances, in which the consistency of various views and labels of clusters is incorporated with contrastive learning. In this case, MRL_CAL simultaneously learns multi-level features of instances in various subspaces, which not only overcomes the confliction of representations but also improves the quality of features. Finally, MRL_CAL transforms incomplete multi-view clustering into an overall objective, where features are learned under the guidance of clustering. Extensive experimental results indicate that MRL_CAL outperforms state-of-the-art algorithms in terms of various measurements, implying that the proposed method is promising for incomplete multi-view clustering.
更多查看译文
关键词
Incomplete multi-view clustering,Contrastive learning,Adversarial learning,Multi-level representation learning
AI 理解论文
溯源树
样例
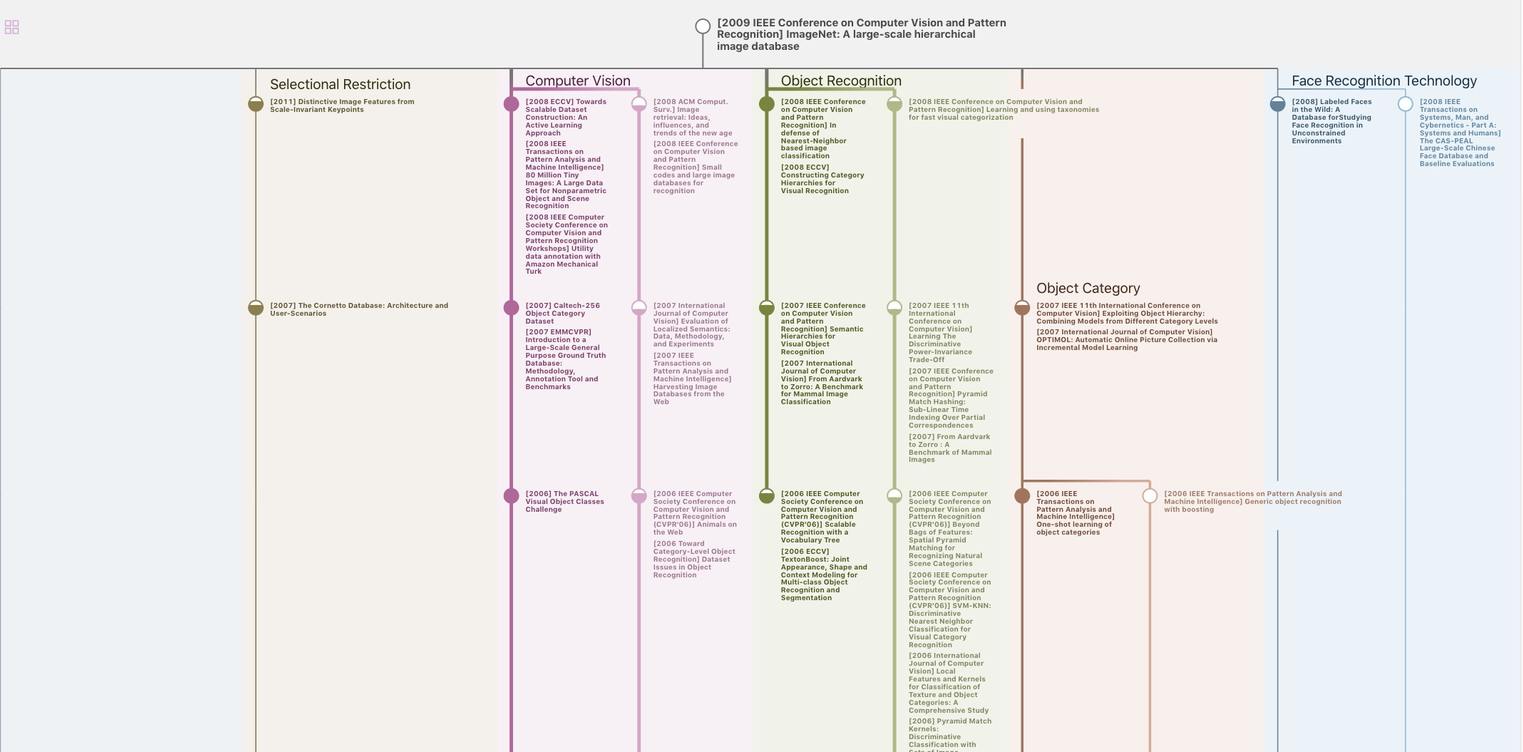
生成溯源树,研究论文发展脉络
Chat Paper
正在生成论文摘要