Trans-Trip: Translation-based embedding with Triplets for Heterogeneous Graphs.
International Conference on Knowledge-Based Intelligent Information & Engineering Systems(2023)
摘要
Heterogeneous graphs (HG) are an effective way of abstracting complex systems, including social, biological, and economic systems. However, modeling these graphs is challenging due to their high dimensionality, sparsity, and heterogeneity. Traditional approaches designed for homogeneous graphs struggle to handle the diverse types of entities and relationships present in HG, leading to a loss of information and potentially inaccurate embeddings. To address these challenges, we introduce a novel method, Trans-Trip: Translation-based embedding with Triplets for Heterogeneous Graphs, that leverages the power of triplets of (entity, relation, entity) to accurately represent the various types of relationships among nodes and links. Trans-trip effectively captures the rich semantics embedded in HGs, overcoming the challenges of global coherence and entity projection. By leveraging the flexibility and interpretability of triplets, our method can handle the multi-types nodes/links present in HG and can capture complex higher-order structures. We demonstrate the effectiveness of our proposed method on several benchmark datasets, showing that it outperforms existing embedding methods. Trans-trip provides a more accurate and interpretable representation of HG, which can be used across various fields, such as biology, social networks, and e-commerce.
更多查看译文
关键词
Heterogeneous graphs (HG),Network representation learning,Graph embedding, Translation-based model
AI 理解论文
溯源树
样例
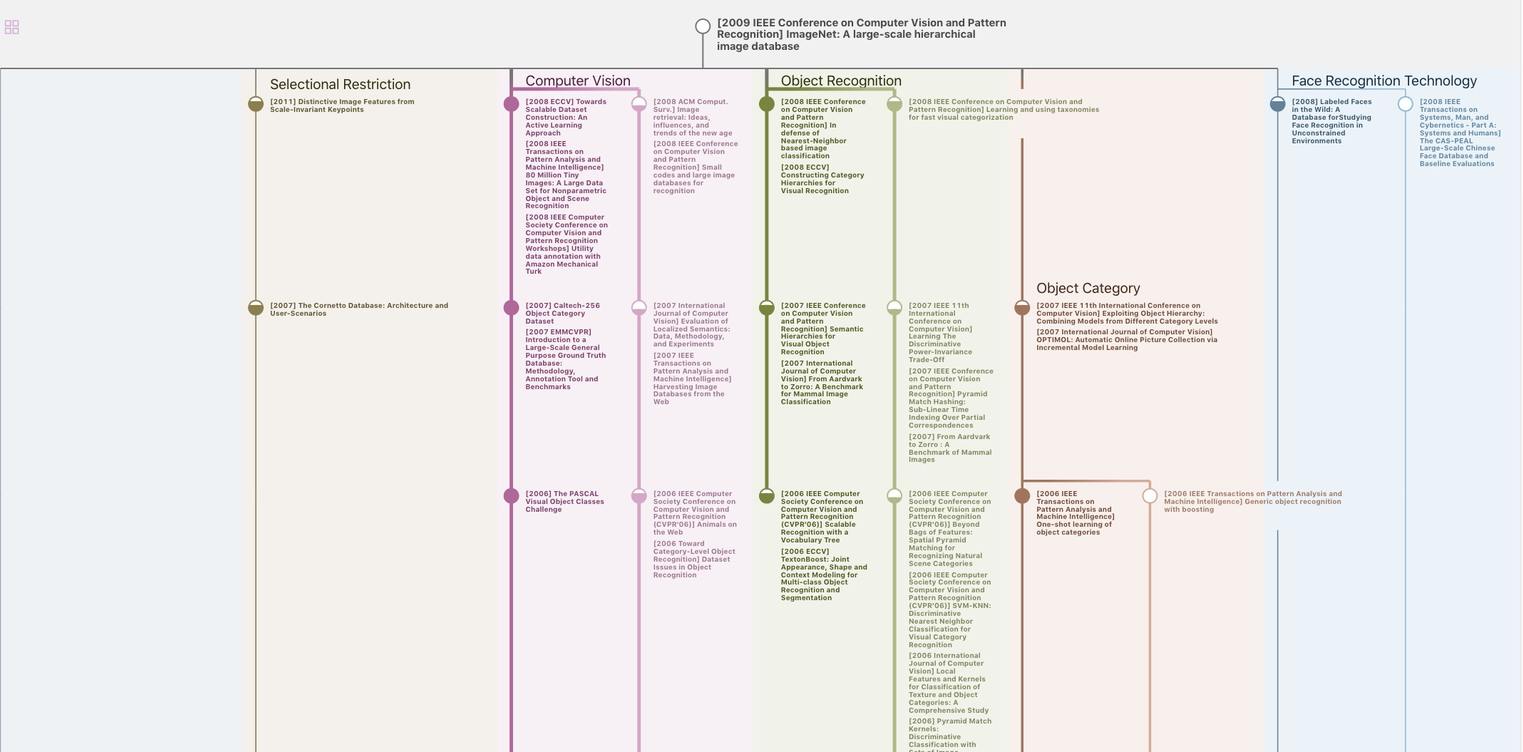
生成溯源树,研究论文发展脉络
Chat Paper
正在生成论文摘要