ECG arrhythmia detection in an inter-patient setting using Fourier decomposition and machine learning
MEDICAL ENGINEERING & PHYSICS(2024)
摘要
ECG beat classification or arrhythmia detection through artificial intelligence (AI) is an active topic of research. It is vital to recognize and detect the type of arrhythmia for monitoring cardiac abnormalities. The AI -based ECG beat classification algorithms proposed in the literature suffer from two main drawbacks. Firstly, some of the works have not considered any unseen test data to validate the performance of their algorithms. Secondly, the accuracy of detecting superventricular ectopic beats (SVEB) needs to be improved. In this work, we address these issues by considering an inter -patient paradigm where the test dataset is collected from a different set of subjects than the training data. Also, the proposed methodology detects SVEB with an F1 score of 89.35%, which is better than existing algorithms. We have used the Fourier decomposition method (FDM) for multi -scale analysis of ECG signals and extracted time -domain and statistical features from the narrow -band signal components obtained using FDM. Feature selection techniques, including the Kruskal-Wallis test and minimum redundancy maximum relevance (mRMR) have been used to select only the relevant features and rank these features to remove any redundancy. Since the dataset used is highly imbalanced, Mathew's correlation coefficient (MCC) has also been used to analyze the performance of the proposed method. Support vector machine classifier with linear kernel achieves an overall 98.03% accuracy and 91.84% MCC for the MIT-BIH arrhythmia dataset.
更多查看译文
关键词
Arrhythmia detection,Beat classification,ECG,Fourier decomposition method,Machine learning
AI 理解论文
溯源树
样例
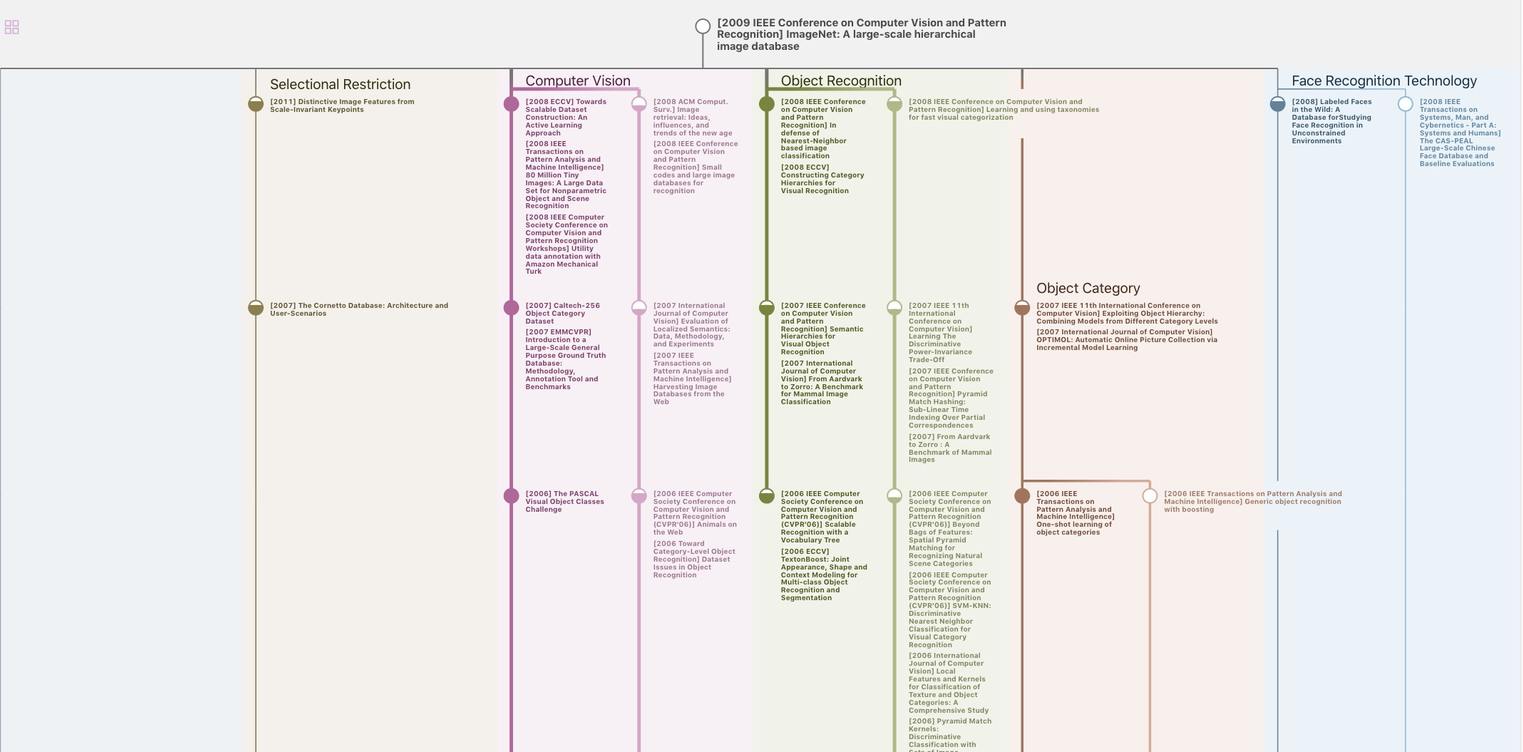
生成溯源树,研究论文发展脉络
Chat Paper
正在生成论文摘要