Identification of high-risk population of pneumoconiosis using deep learning segmentation of lung 3D images and radiomics texture analysis.
Computer methods and programs in biomedicine(2024)
摘要
OBJECTION:The aim of this study is to develop an early-warning model for identifying high-risk populations of pneumoconiosis by combining lung 3D images and radiomics lung texture features.
METHODS:A retrospective study was conducted, including 600 dust-exposed workers and 300 confirmed pneumoconiosis patients. Chest computed tomography (CT) images were divided into a training set and a test set in a 2:1 ratio. Whole-lung segmentation was performed using deep learning models for feature extraction of radiomics. Two feature selection algorithms and five classification models were used. The optimal model was selected using a 10-fold cross-validation strategy, and the calibration curve and decision curve were evaluated. To verify the applicability of the model, the diagnostic efficiency and accuracy between the model and human interpretation were compared. Additionally, the risk probabilities for different risk groups defined by the model were compared at different time intervals.
RESULTS:Four radiomics features were ultimately used to construct the predictive model. The logistic regression model was the most stable in both the training set and testing set, with an area under curve (AUC) of 0.964 (95 % confidence interval [CI], 0.950-0.976) and 0.947 (95 %CI, 0.925-0.964). In the training and testing sets, the Brier scores were 0.092 and 0.14, respectively, with threshold probability ranges of 2 %-99 % and 2 %-85 %. These results indicate that the model exhibits good calibration and clinical benefit. The comparison between the model and human interpretation showed that the model was not inferior in terms of diagnostic efficiency and accuracy. Additionally, the high-risk population identified by the model was diagnosed as pneumoconiosis two years later.
CONCLUSION:This study provides a meticulous and quantifiable method for detecting and assessing the risk of pneumoconiosis, building upon accurate diagnosis. Employing risk scoring and probability estimation, not only enhances the efficiency of diagnostic physicians but also provides a valuable reference for controlling the occurrence of pneumoconiosis.
更多查看译文
AI 理解论文
溯源树
样例
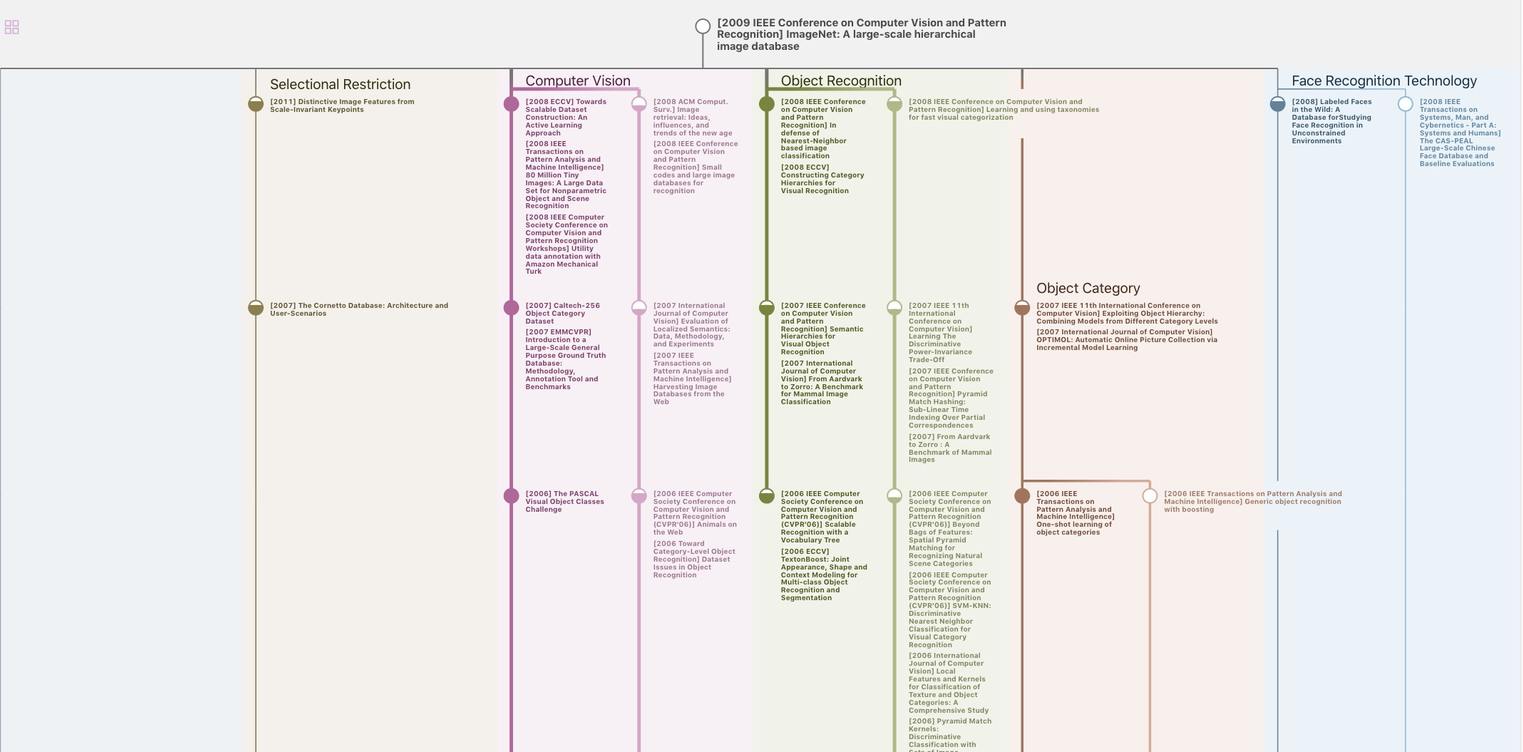
生成溯源树,研究论文发展脉络
Chat Paper
正在生成论文摘要