Multiomics-integrated deep language model enables in silico genome-wide detection of transcription factor binding site in unexplored biosamples
BIOINFORMATICS(2024)
摘要
Motivation Transcription factor binding sites (TFBS) are regulatory elements that have significant impact on transcription regulation and cell fate determination. Canonical motifs, biological experiments, and computational methods have made it possible to discover TFBS. However, most existing in silico TFBS prediction models are solely DNA-based, and are trained and utilized within the same biosample, which fail to infer TFBS in experimentally unexplored biosamples.Results Here, we propose TFBS prediction by modified TransFormer (TFTF), a multimodal deep language architecture which integrates multiomics information in epigenetic studies. In comparison to existing computational techniques, TFTF has state-of-the-art accuracy, and is also the first approach to accurately perform genome-wide detection for cell-type and species-specific TFBS in experimentally unexplored biosamples. Compared to peak calling methods, TFTF consistently discovers true TFBS in threshold tuning-free way, with higher recalled rates. The underlying mechanism of TFTF reveals greater attention to the targeted TF's motif region in TFBS, and general attention to the entire peak region in non-TFBS. TFTF can benefit from the integration of broader and more diverse data for improvement and can be applied to multiple epigenetic scenarios.Availability and implementation We provide a web server (https://tftf.ibreed.cn/) for users to utilize TFTF model. Users can train TFTF model and discover TFBS with their own data.
更多查看译文
AI 理解论文
溯源树
样例
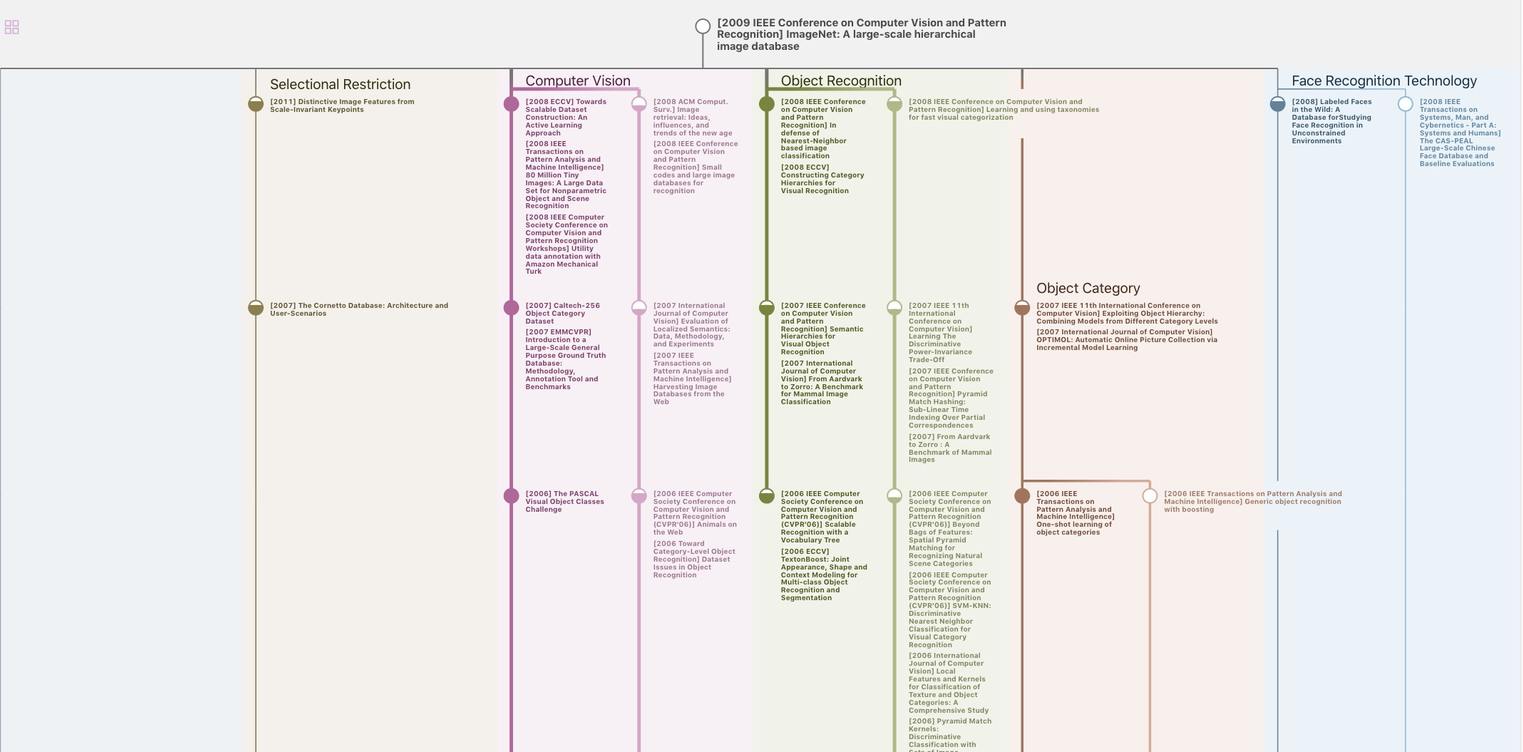
生成溯源树,研究论文发展脉络
Chat Paper
正在生成论文摘要