A System for Automated Vehicle Damage Localization and Severity Estimation Using Deep Learning
IEEE TRANSACTIONS ON INTELLIGENT TRANSPORTATION SYSTEMS(2023)
摘要
Vehicle damage localization and severity estimation is essential to post-accident assessments, with a traditional process taking an average of seven days and requiring substantial work from both customers and dealers. Towards improving this process, we propose an end-to-end system which inputs a set of user-acquired photographs of a vehicle after an accident and outputs a damage assessment report including the set of damaged parts and the type and size of the damage for each part. The system is composed of three deep learning modules: a model to identify whether a vehicle is present in the image, a model to localize the vehicle parts in the image, and a model to localize the damage in the image. We demonstrate the effectiveness of each module by evaluating them on labeled datasets containing images of vehicles after an accident, some collected by the OE (Original Equipment) Insured Fleet and some acquired by users of the OEM (Original Equipment Manufacturer) mobile application. We also describe how the modules fit together with a post-processing step to aggregate outputs between the different modules across multiple user-acquired views of the accident. Our approach demonstrates the potential for an accurate and automated vehicle damage estimation system to support a substantially more efficient vehicle damage assessment process.
更多查看译文
关键词
Vehicle part localization,vehicle damage estimation,deep learning,computer vision,machine learning
AI 理解论文
溯源树
样例
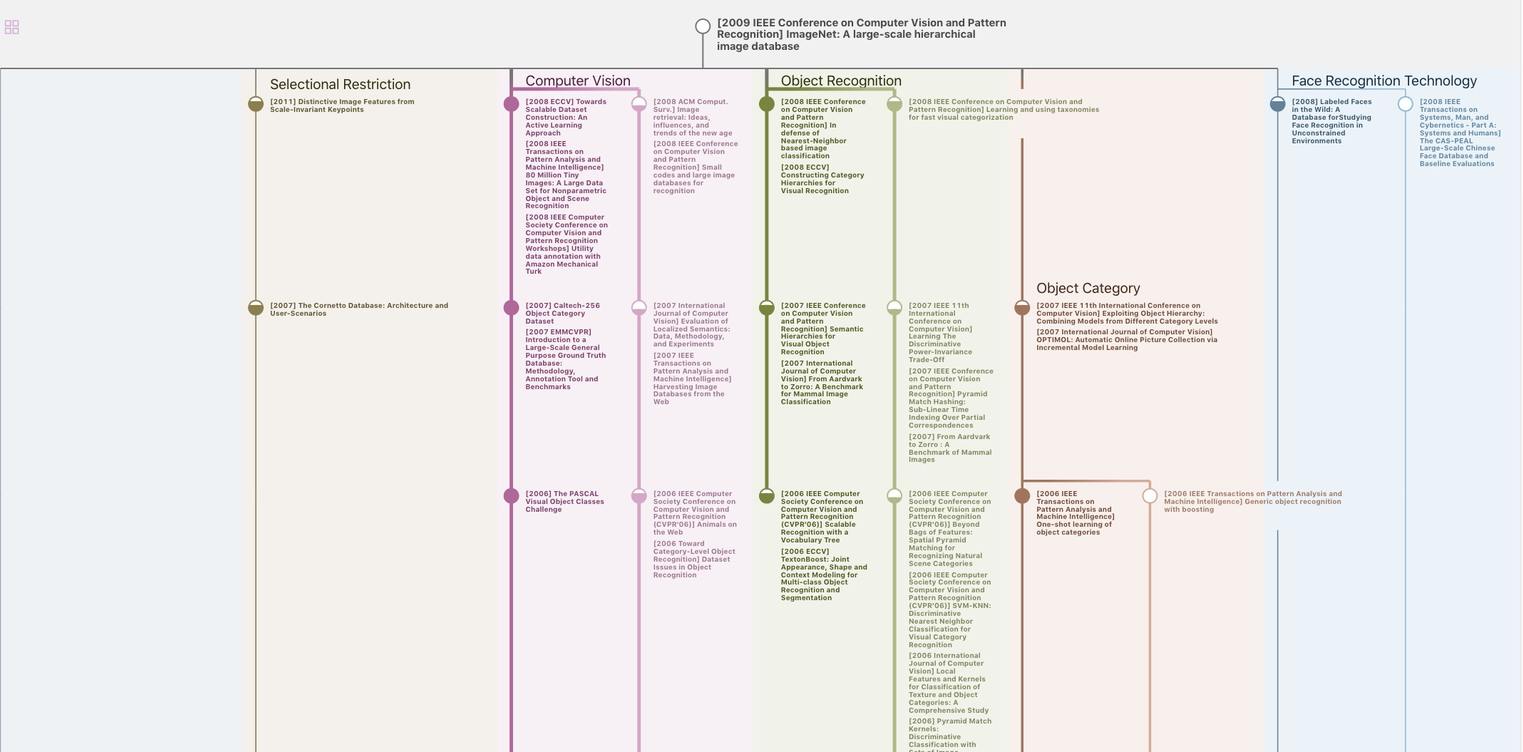
生成溯源树,研究论文发展脉络
Chat Paper
正在生成论文摘要