Engineered Vertically Stacked NSFET Charge-Trapping Synapse for Neuromorphic Applications
ACS APPLIED ELECTRONIC MATERIALS(2023)
Abstract
In this work, a vertically stacked nanosheet FET (NSFET) is engineered for neuromorphic applications, which consists of nonidentical sheets in the vertical direction. The proposed NSFET has three nanosheets: two with embedded charge-trapping layers and one without a trap layer. This helps enhance the electric field along the channel direction of the device and thus the conductance of an electronic synaptic device. The estimated conductance values from the vertical nanosheet FET are used as a weight in the convolutional neural network (CNN) algorithm for image classification for the Canadian Institute for Advanced Research (CIFAR)-10 dataset. Results show improved performance in terms of nonlinearity, accuracy, and a higher conductance value compared to the conventional NSFET (identical sheets with a charge-trapping layer). Moreover, the engineered NSFET device has a high density and achieves similar to 88.8% accuracy for image classification, which makes it a promising candidate for next-generation neuromorphic computing.
MoreTranslated text
Key words
vertical NSFET,synaptic transistor,potentiation,depression,LTP and LTD,neural network,neuromorphic computing
AI Read Science
Must-Reading Tree
Example
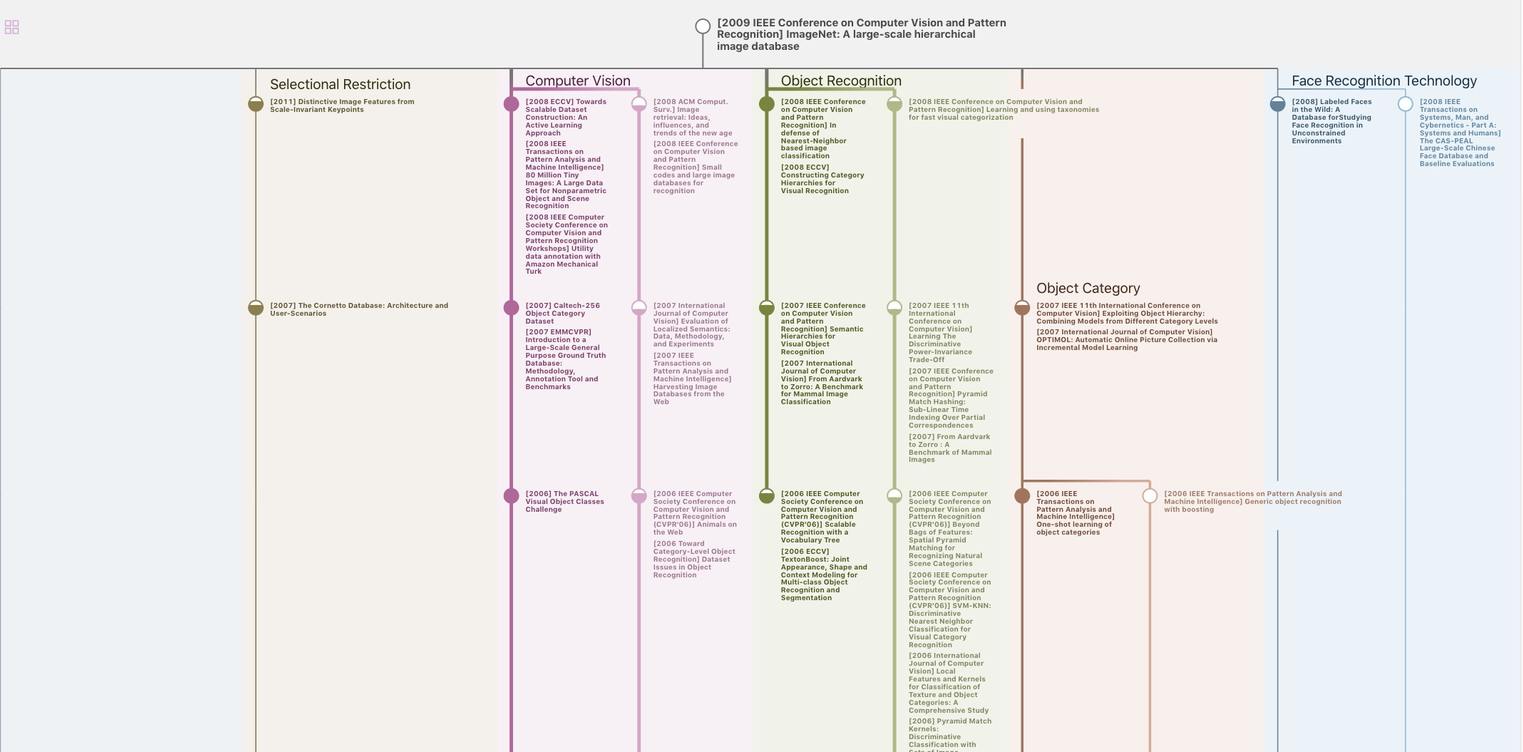
Generate MRT to find the research sequence of this paper
Chat Paper
Summary is being generated by the instructions you defined