Robust Reconstruction of the Void Fraction from Noisy Magnetic Flux Density Using Invertible Neural Networks
SENSORS(2024)
摘要
Electrolysis stands as a pivotal method for environmentally sustainable hydrogen production. However, the formation of gas bubbles during the electrolysis process poses significant challenges by impeding the electrochemical reactions, diminishing cell efficiency, and dramatically increasing energy consumption. Furthermore, the inherent difficulty in detecting these bubbles arises from the non-transparency of the wall of electrolysis cells. Additionally, these gas bubbles induce alterations in the conductivity of the electrolyte, leading to corresponding fluctuations in the magnetic flux density outside of the electrolysis cell, which can be measured by externally placed magnetic sensors. By solving the inverse problem of the Biot-Savart Law, we can estimate the conductivity distribution as well as the void fraction within the cell. In this work, we study different approaches to solve the inverse problem including Invertible Neural Networks (INNs) and Tikhonov regularization. Our experiments demonstrate that INNs are much more robust to solving the inverse problem than Tikhonov regularization when the level of noise in the magnetic flux density measurements is not known or changes over space and time.
更多查看译文
关键词
machine learning,invertible neural networks,normalizing flows,water electrolysis,Biot-Savart law,inverse problems,current tomography,random error diffusion
AI 理解论文
溯源树
样例
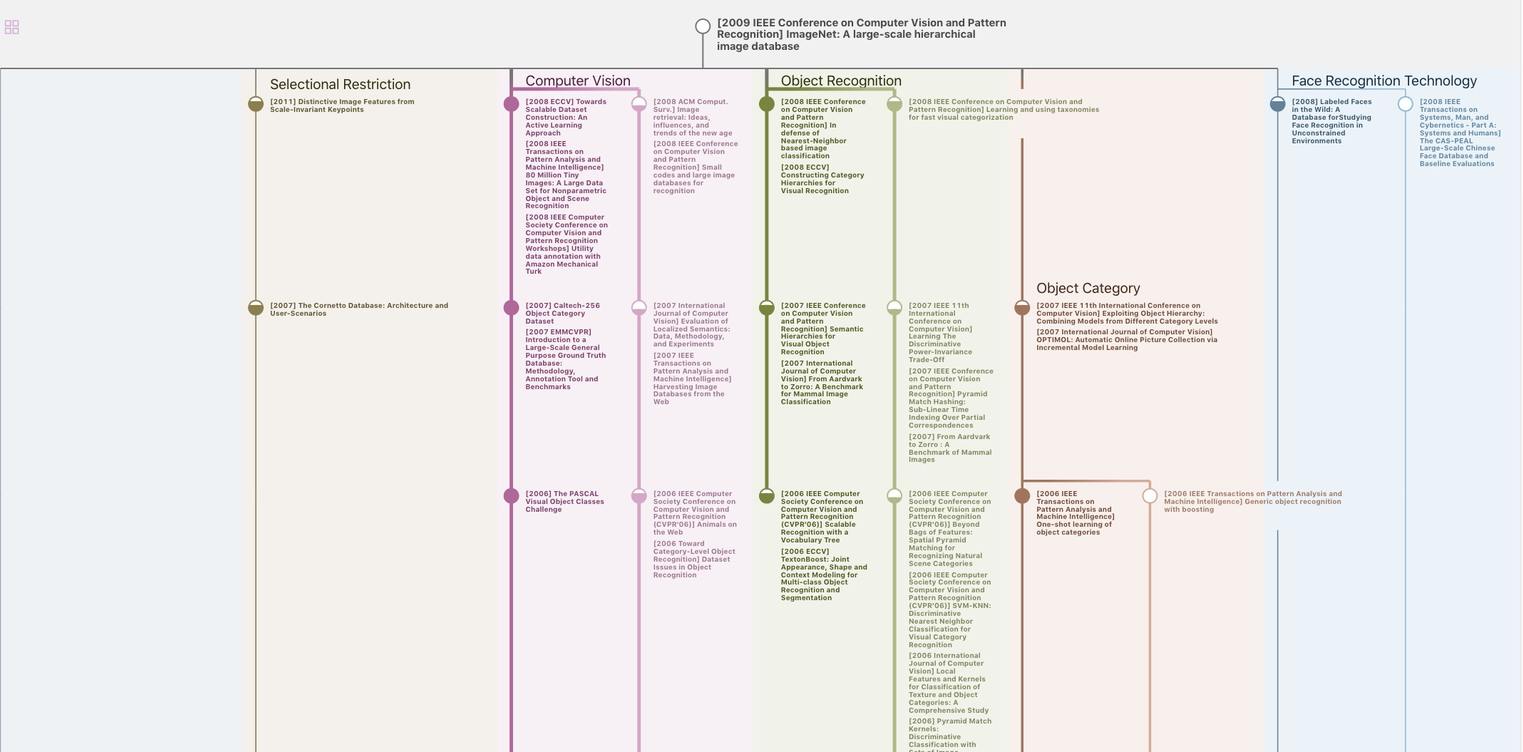
生成溯源树,研究论文发展脉络
Chat Paper
正在生成论文摘要