A semi-self-supervised learning model to recognize handwritten characters in ancient documents in Indian scripts
Neural Computing and Applications(2024)
摘要
An optical character recognition (OCR) system segments the character from the given document before recognizing it. The recognition of such character images requires the class labels to be associated with each character sample in the training set, and this requires the placing of all the samples of each segmented character in various distinct folders. However, it has to be done manually, and thus, it is a time-consuming process. The ancient documents suffer from humidity spots, ink stains, and faded portions of text which makes the character recognition task even more challenging for the ancient documents. The present article proposes a novel semi-self-supervised learning-based OCR method to recognize each character segmented from the ancient documents handwritten in Devanagari and Maithili scripts. The proposed method has two modules—feature extraction module and recognition module. The feature extraction module has extracted deep hierarchical features from each pre-segmented character image employing generative self-supervised learning approach. The recognition module has focused on important features using an attention mechanism and learns the long temporal sequence using the Gated Recurrent Unit variant of recurrent neural network classifier to classify each segmented character into its proper class. The feature extraction module in the proposed method has been trained using the 60% (unlabelled) of the dataset, whereas the recognition module has been trained using the 5% (manually labelled) of the dataset. The performance of the proposed novel OCR method has been evaluated on two self-generated datasets of ancient handwritten documents in Devanagari and Maithili scripts. The experimental results demonstrate that the proposed OCR method outperforms the state-of-the-art (SOTA) methods in this regard. The proposed OCR method has improved the character recognition accuracy in comparison with the SOTA methods by 2.27% and 3.48% in Devanagari and Maithili scripts, respectively.
更多查看译文
关键词
Character recognition,Semi-self-supervised learning,Ancient handwritten documents,Indian scripts
AI 理解论文
溯源树
样例
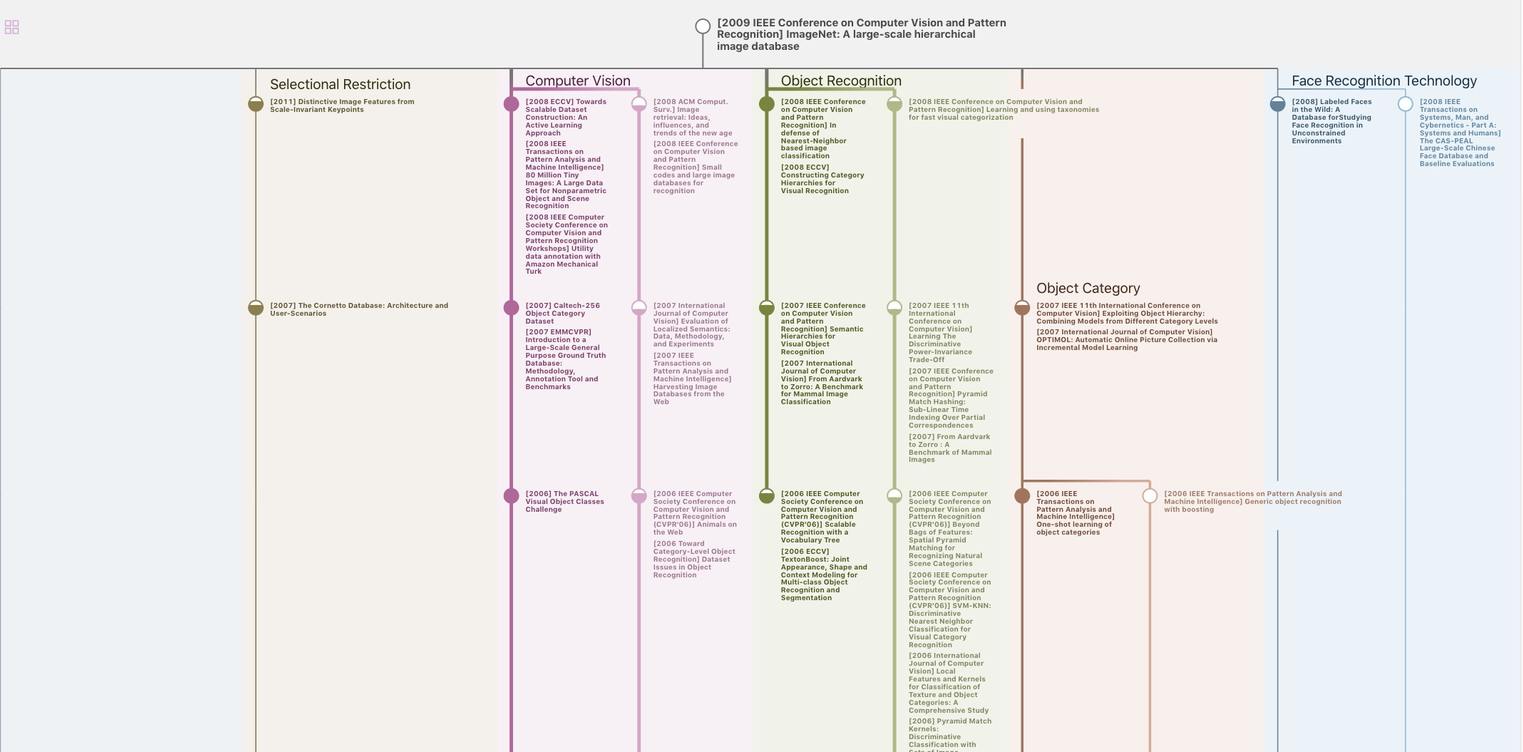
生成溯源树,研究论文发展脉络
Chat Paper
正在生成论文摘要