From low resource information extraction to identifying influential nodes in knowledge graphs
CoRR(2024)
摘要
We propose a pipeline for identifying important entities from intelligence reports that constructs a knowledge graph, where nodes correspond to entities of fine-grained types (e.g. traffickers) extracted from the text and edges correspond to extracted relations between entities (e.g. cartel membership). The important entities in intelligence reports then map to central nodes in the knowledge graph. We introduce a novel method that extracts fine-grained entities in a few-shot setting (few labeled examples), given limited resources available to label the frequently changing entity types that intelligence analysts are interested in. It outperforms other state-of-the-art methods. Next, we identify challenges facing previous evaluations of zero-shot (no labeled examples) methods for extracting relations, affecting the step of populating edges. Finally, we explore the utility of the pipeline: given the goal of identifying important entities, we evaluate the impact of relation extraction errors on the identification of central nodes in several real and synthetic networks. The impact of these errors varies significantly by graph topology, suggesting that confidence in measurements based on automatically extracted relations should depend on observed network features.
更多查看译文
AI 理解论文
溯源树
样例
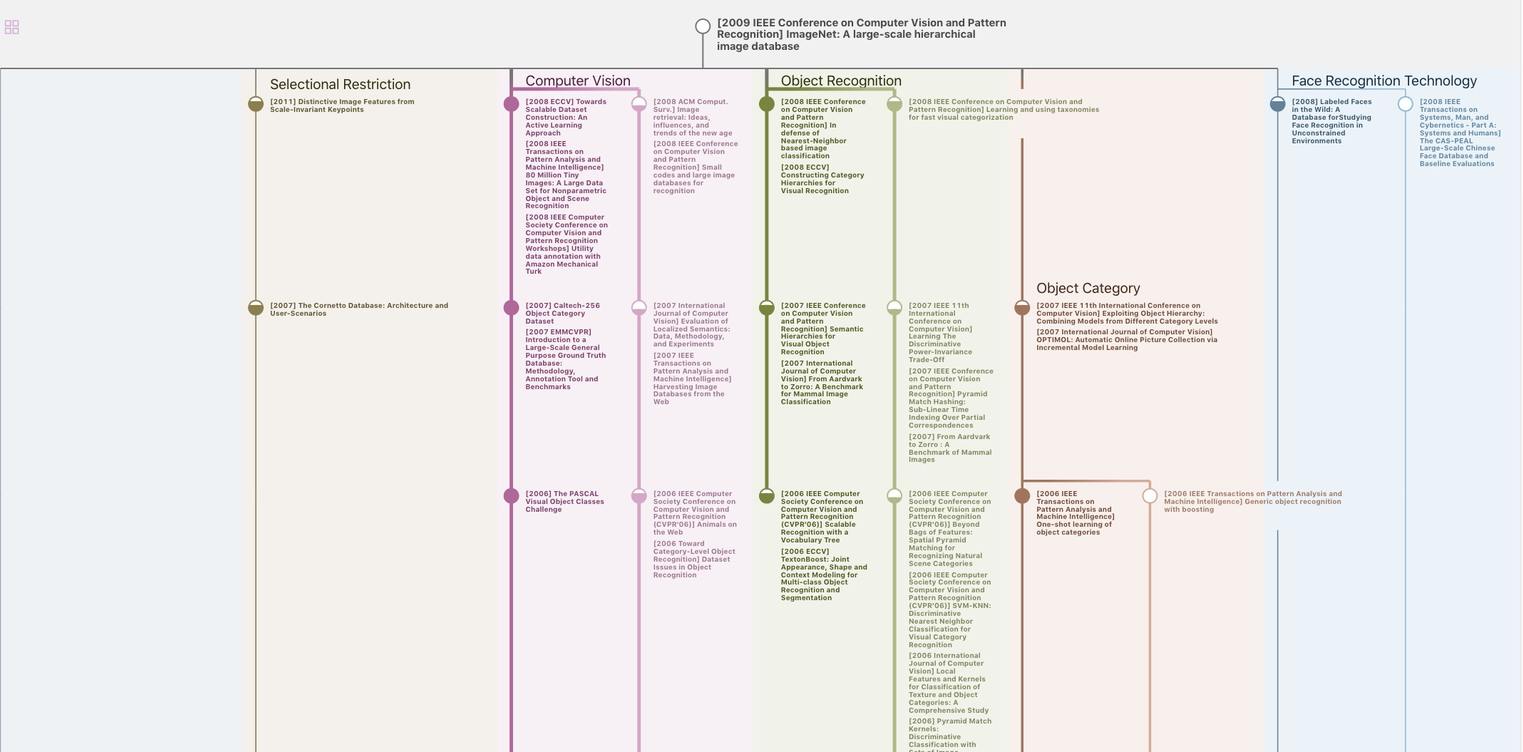
生成溯源树,研究论文发展脉络
Chat Paper
正在生成论文摘要