High-performance soil class delineation via UMAP coupled with machine learning in Kurdistan Province, Iran
GEODERMA REGIONAL(2024)
摘要
In response to the demand for spatial information on the soil to support the sustainable management of soil resources, this study applies a digital soil mapping approach to predict soil classes for a 7000 ha area, located in Kurdistan province, Iran. Based on a stratified random sampling design, 91 soil profiles were situated, described, and classified into soil great groups. Environmental covariates used for modelling soil classes included terrain derivatives, remote sensing data, distance-based rasters, and legacy geospatial information (e.g., geological map). To address the issue of data multi-collinearity among the predictors, three dimensionality reduction techniques were tested: the principal component analysis (PCA), t-distributed stochastic neighbor embedding (tSNE), and the novel Uniform Manifold Approximation and Projection (UMAP). An initial suite of 160 environmental covariates was reduced to 10 for all the methods and used to train a Random Forest (RF) model. The most effective model coupled UMAP with the Random Forest (RF-UMAP) machine-learner, which yielded a kappa index and overall accuracy values of 0.73 and 0.80, respectively. Within Kurdistan, topography and parent material were the main soil-forming factors influencing the prediction of the soil classes. Overall, the use of UMAP outperformed PCA and t-SNE. This study demonstrates the value of using advanced dimension reduction methods to facilitate the handling of non-linear relationships among predictor variables when using RF.
更多查看译文
关键词
Entisols and Inceptisols,Iran,Machine learning,Soil classes,Land surface parameters,Legacy soil information,Data reduction,Random forest,Uniform manifold approximation and,projection
AI 理解论文
溯源树
样例
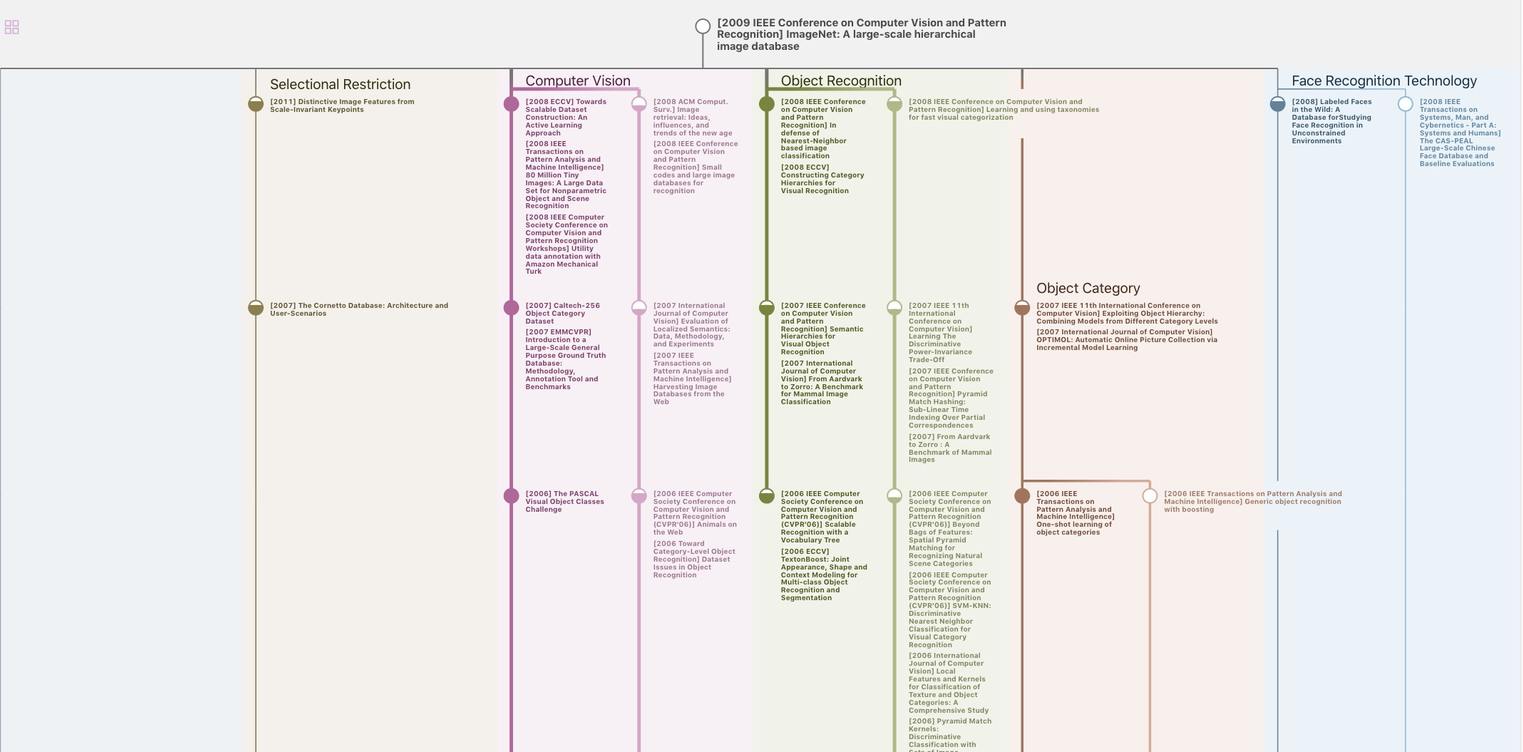
生成溯源树,研究论文发展脉络
Chat Paper
正在生成论文摘要