Automated Laryngeal Cancer Detection and Classification Using Dwarf Mongoose Optimization Algorithm with Deep Learning
CANCERS(2024)
摘要
Simple Summary Laryngeal cancer poses a major global health burden, with late-stage diagnoses contributing to decreased survival rates. Recently, deep learning and deep convolutional neural network models have exhibited significant attention in the diagnosis of various diseases like skin cancer and diabetic retinopathy. Therefore, this study focuses on the design and development of a deep learning-based laryngeal cancer detection and classification model. The proposed model exploited a hyperparameter-tuned EfficientNetB0 model with a multi-head bidirectional gated recurrent unit for classification. In addition, the Dwarf Mongoose Optimization algorithm is applied for the hyperparameter tuning process. The experimental results stated that the proposed model is found to be an accurate and reliable approach for automated detection of laryngeal cancer.Abstract Laryngeal cancer (LCA) is a serious disease with a concerning global rise in incidence. Accurate treatment for LCA is particularly challenging in later stages, due to its complex nature as a head and neck malignancy. To address this challenge, researchers have been actively developing various analysis methods and tools to assist medical professionals in efficient LCA identification. However, existing tools and methods often suffer from various limitations, including low accuracy in early-stage LCA detection, high computational complexity, and lengthy patient screening times. With this motivation, this study presents an Automated Laryngeal Cancer Detection and Classification using a Dwarf Mongoose Optimization Algorithm with Deep Learning (ALCAD-DMODL) technique. The main objective of the ALCAD-DMODL method is to recognize the existence of LCA using the DL model. In the presented ALCAD-DMODL technique, a median filtering (MF)-based noise removal process takes place to get rid of the noise. Additionally, the ALCAD-DMODL technique involves the EfficientNet-B0 model for deriving feature vectors from the pre-processed images. For optimal hyperparameter tuning of the EfficientNet-B0 model, the DMO algorithm can be applied to select the parameters. Finally, the multi-head bidirectional gated recurrent unit (MBGRU) model is applied for the recognition and classification of LCA. The simulation result analysis of the ALCAD-DMODL technique is carried out on the throat region image dataset. The comparison study stated the supremacy of the ALCAD-DMODL technique in terms of distinct measures.
更多查看译文
关键词
laryngeal cancer,Dwarf Mongoose Optimization,deep learning,endoscopy,median filtering,multi-head bidirectional gated recurrent unit
AI 理解论文
溯源树
样例
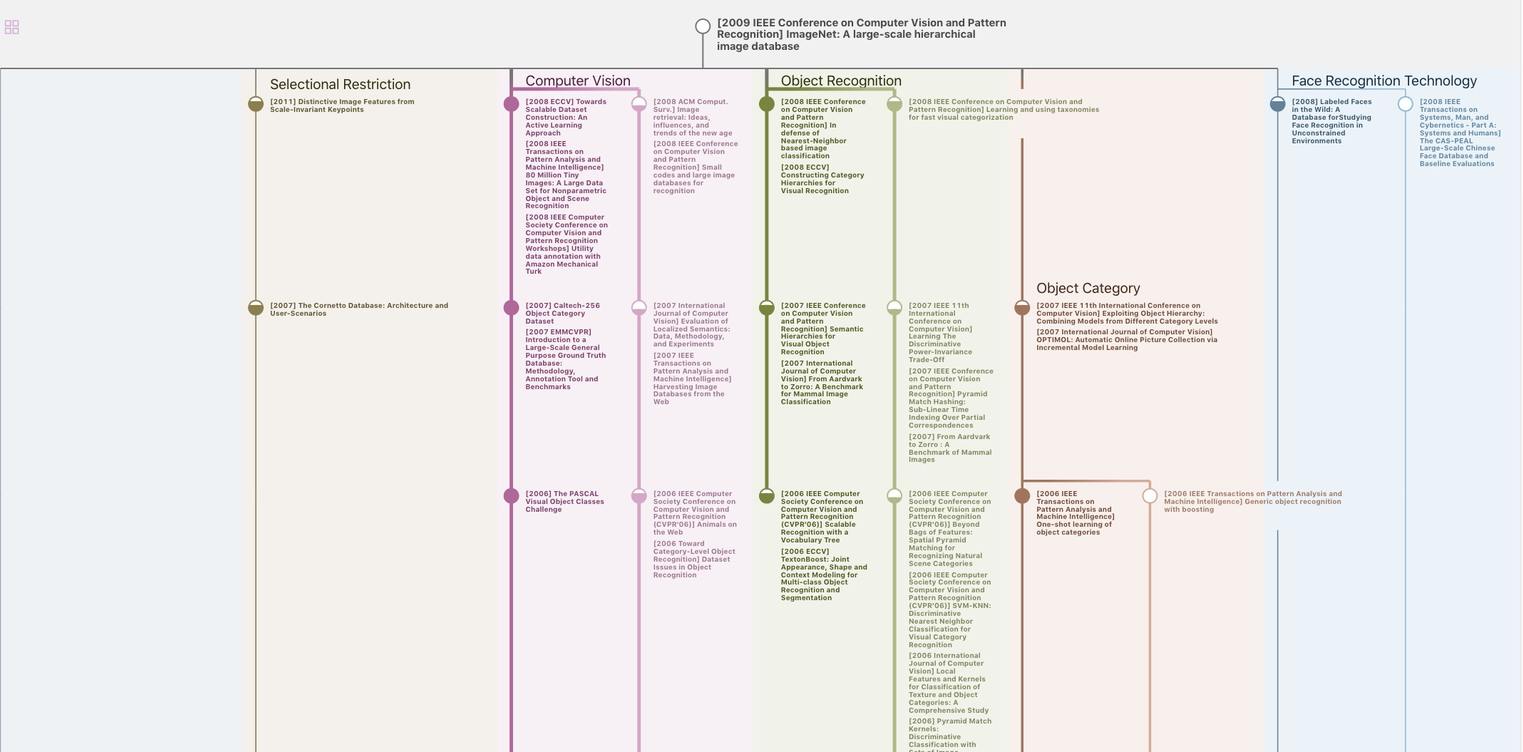
生成溯源树,研究论文发展脉络
Chat Paper
正在生成论文摘要