Self-Supervised Hyperspectral Anomaly Detection Based on Finite Spatialwise Attention
IEEE TRANSACTIONS ON GEOSCIENCE AND REMOTE SENSING(2024)
摘要
Hyperspectral anomaly detection (HAD) is of great value in both practical and theoretical terms. However, due to the lack of available semantic labels, previous works mainly relied on unsupervised or semisupervised methods to construct learning models, which inevitably lacked semantic guidance and led to limited AD effectiveness. Besides, few previous methods jointly mine spectral and spatial global dependencies, which limits their effectiveness in practical scenarios. To address the above-mentioned problems, we design a novel self-supervised HAD method, named the Self-Supervised HAD method based on the finite spatialwise attention (FSA). The core of the proposed method is the designed self-supervised HAD transFormer (SSHADFormer). It explores the specific spectral attributes of hyperspectral images (HSIs) to reconstruct background HSI from a given RGB image, which solves the difficulty of acquiring semantic information and enhances the agility of AD models. In addition, we propose an FSA mechanism. The mechanism mines the cluster structure of the background spectrum in a data-driven manner, enhancing the discriminative ability between background and anomalous targets while avoiding anomalous target interference during training. Extensive experiments on six public datasets demonstrate the effectiveness and agility of the proposed method.
更多查看译文
关键词
Hyperspectral anomaly detection (HAD),self-attention mechanism,self-supervised learning,spatial cluster,transformer
AI 理解论文
溯源树
样例
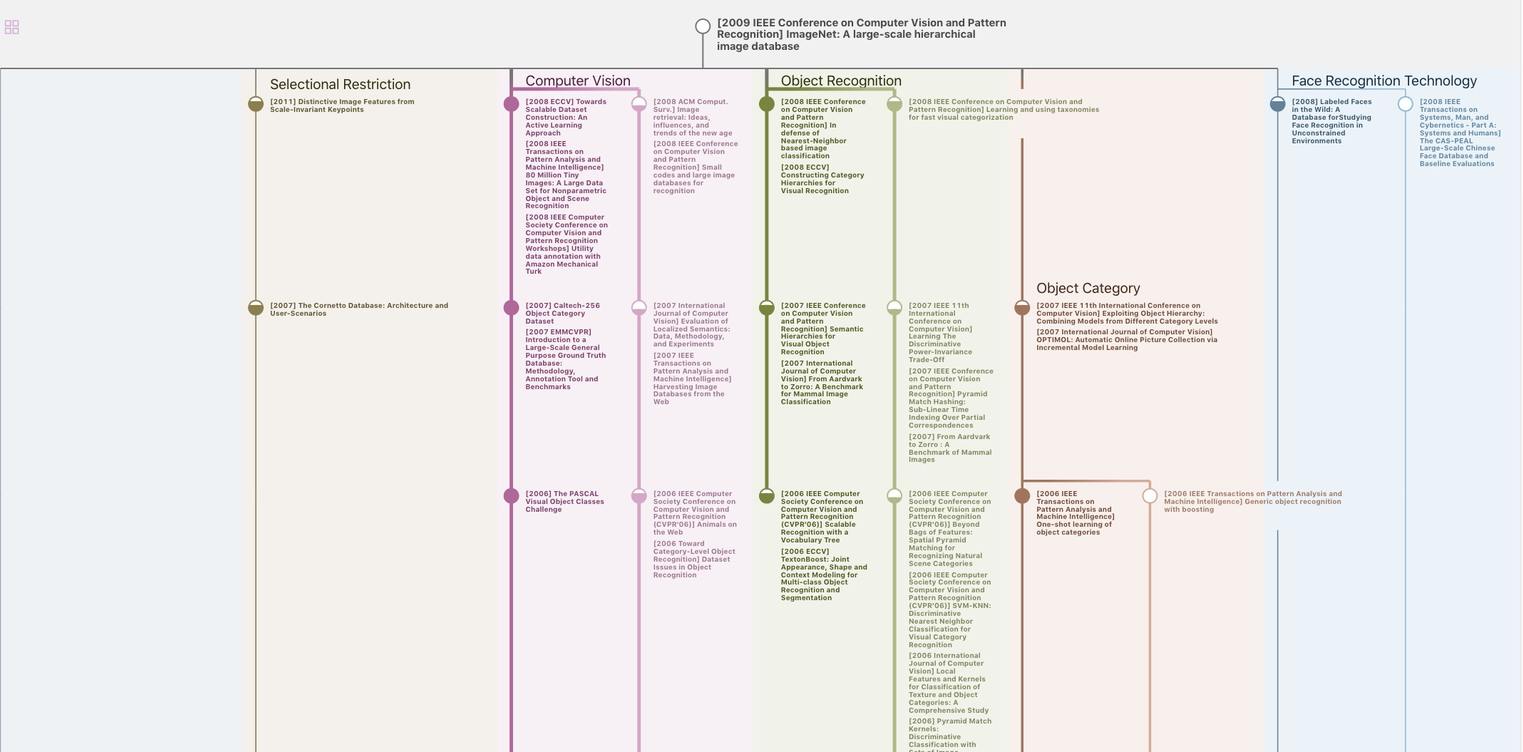
生成溯源树,研究论文发展脉络
Chat Paper
正在生成论文摘要