DiffTAD: Denoising diffusion probabilistic models for vehicle trajectory anomaly detection
KNOWLEDGE-BASED SYSTEMS(2024)
摘要
Vehicle trajectory anomaly detection plays an essential role in the fields of traffic video surveillance, autonomous driving navigation, and taxi fraud detection. Deep generative models have been shown to be promising solutions for anomaly detection, avoiding the costs involved in manual labeling. However, existing popular generative models such as Generative Adversarial Networks (GANs) and Variational AutoEncoders (VAEs) are often plagued by training instability, mode collapse, and poor sample quality. To resolve the dilemma, we present DiffTAD, a novel vehicle trajectory anomaly detection framework based on the emerging diffusion models. DiffTAD formalizes anomaly detection as a noisy -to -normal process that progressively adds noise to the vehicle trajectory until the path is corrupted to pure Gaussian noise. The core idea of our framework is to devise deep neural networks to learn the reverse of the diffusion process and to detect anomalies by comparing the difference between a query trajectory and its reconstruction. DiffTAD is a parameterized Markov chain trained with variational inference and allows the mean square error to optimize the reweighted variational lower bound. In addition, DiffTAD integrates decoupled Transformer -based temporal and spatial encoders to model the temporal dependencies and spatial interactions among vehicles in the diffusion models. Experiments on the real -world trajectory dataset TRAFFIC demonstrate that our DiffTAD achieves significant improvements over existing state-of-the-art methods, with the maximum enhancements reaching 25.87% and 35.59% in terms of AUC and F1. While on the synthetic datasets CROSS, SynTra, and MAAD, the maximum improvements in AUC/F1 are 27.47%/38.56%, 25.38%/31.42%, and 58.22%/50.04%, respectively.
更多查看译文
关键词
Anomaly detection,Vehicle trajectory,Diffusion models,Spatio-temporal transformer,Reconstruction error
AI 理解论文
溯源树
样例
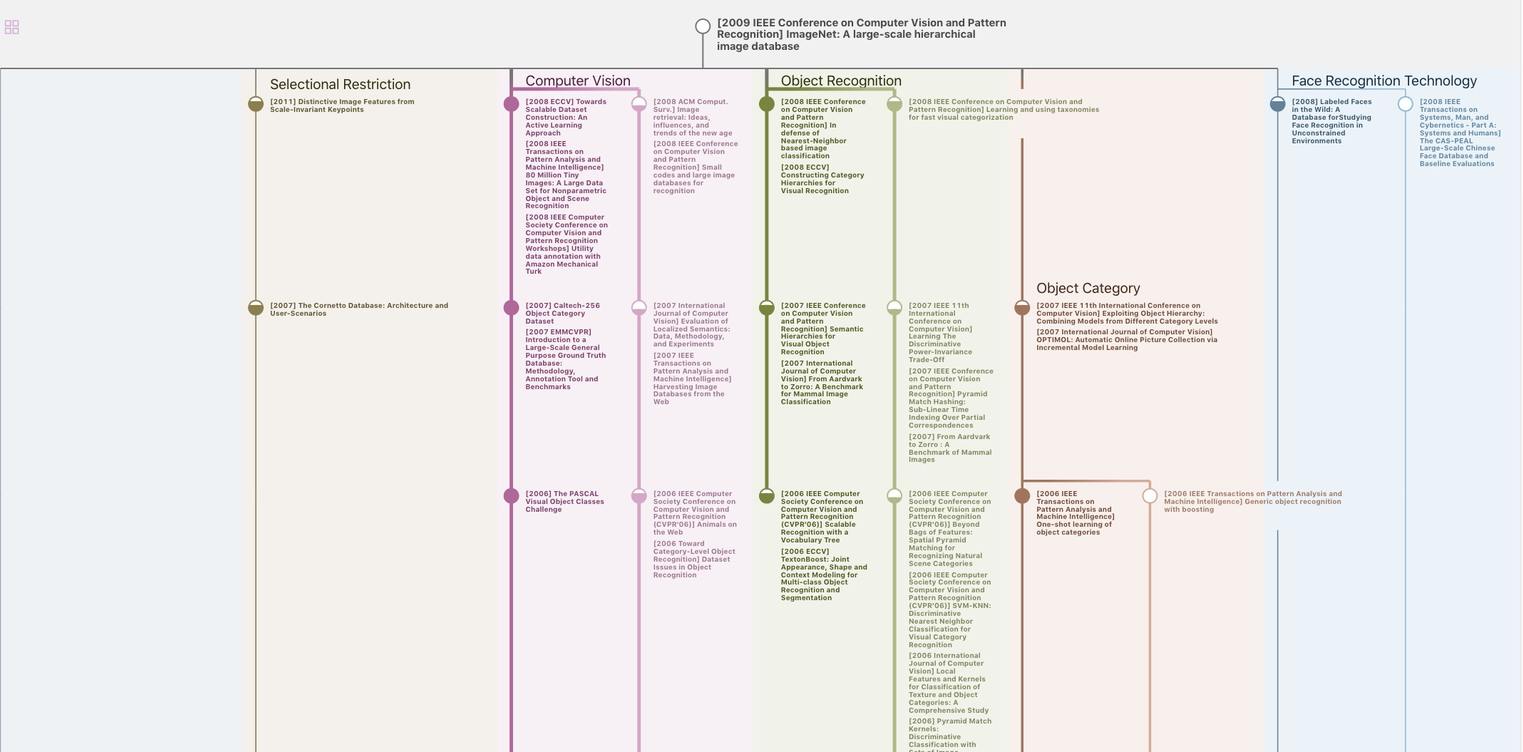
生成溯源树,研究论文发展脉络
Chat Paper
正在生成论文摘要